Revolutionizing Medical QA: The Impact of Knowledge Graph Agents in Biomedical AI
Dec. 14, 2024.
5 mins. read.
6 Interactions
Revolutionizing medical decision-making: KGAREVION blends AI with structured data for unmatched accuracy. Can this innovation transform diagnostics and patient care?
Introduction
Imagine a world where healthcare professionals receive precise, contextually tailored answers to intricate medical questions almost instantaneously. This is no longer a distant dream, thanks to recent advancements in AI-driven medical question-answering (QA) systems. Researchers from Harvard, the University of Illinois, and Imperial College London have developed a groundbreaking approach to address the complexities of biomedical knowledge. Introducing KGAREVION—a Knowledge Graph-based Agent that leverages structured data and AI to filter inaccuracies and connect relevant information seamlessly. This innovative model is poised to transform medical QA, paving the way for AI systems that understand and augment medical decision-making with unprecedented precision.
Redefining Medical Reasoning with Knowledge Graphs
Knowledge Graphs (KGs) offer a sophisticated method of representing intricate relationships within biomedical data. These graphs act as detailed maps where nodes signify entities—such as diseases, treatments, or genes—and edges depict the connections between them. Unlike conventional AI systems, KGAREVION employs a “grounded knowledge” strategy, cross-referencing generated information against these graphs to validate its accuracy. This ensures that outputs are not only precise but also rooted in domain-specific expertise, a critical requirement for decision-making in medicine. According to Harvard researchers, this strategy exemplifies how KGs excel in areas where generic AI models struggle to address the nuances of medical science.
Bridging General and Specialized Knowledge
While large language models (LLMs) like GPT-4 and LLaMA3 demonstrate exceptional general knowledge, they often fall short in specialized fields such as medicine. KGAREVION bridges this divide by seamlessly integrating the general reasoning power of LLMs with the domain-specific precision of medical KGs. When faced with a query, the system retrieves pertinent information from its knowledge graph, verifies its validity, and refines the response to ensure relevance and accuracy. This dual-layered approach is particularly effective in healthcare, where the complexity of queries demands nuanced, context-aware answers. By personalizing responses to the specific needs of each query, KGAREVION sets a new standard for adaptability in medical AI.
A Multi-Step Framework for Complex Queries
Medical questions often encompass multifaceted relationships and overlapping concepts. KGAREVION’s architecture is designed to tackle these challenges through a meticulous multi-step process:
- Generate: The system extracts potential answers in the form of knowledge triplets from a large language model’s database.
- Review: It evaluates these triplets against grounded knowledge graphs to identify and discard inaccuracies.
- Revise: Errors or inconsistencies are rectified, ensuring alignment with validated medical knowledge.
- Answer: The refined, accurate answer is presented, addressing the query with exceptional clarity.
This comprehensive approach allows KGAREVION to excel where traditional models falter. Benchmark evaluations demonstrated a 5.2% improvement in accuracy compared to 15 baseline models, with notable success in addressing queries that require distinguishing between closely related medical terms or conditions. Additionally, on three newly curated datasets of varying complexity, KGAREVION achieved a remarkable 10.4% accuracy improvement, highlighting its capacity to handle intricate medical scenarios.
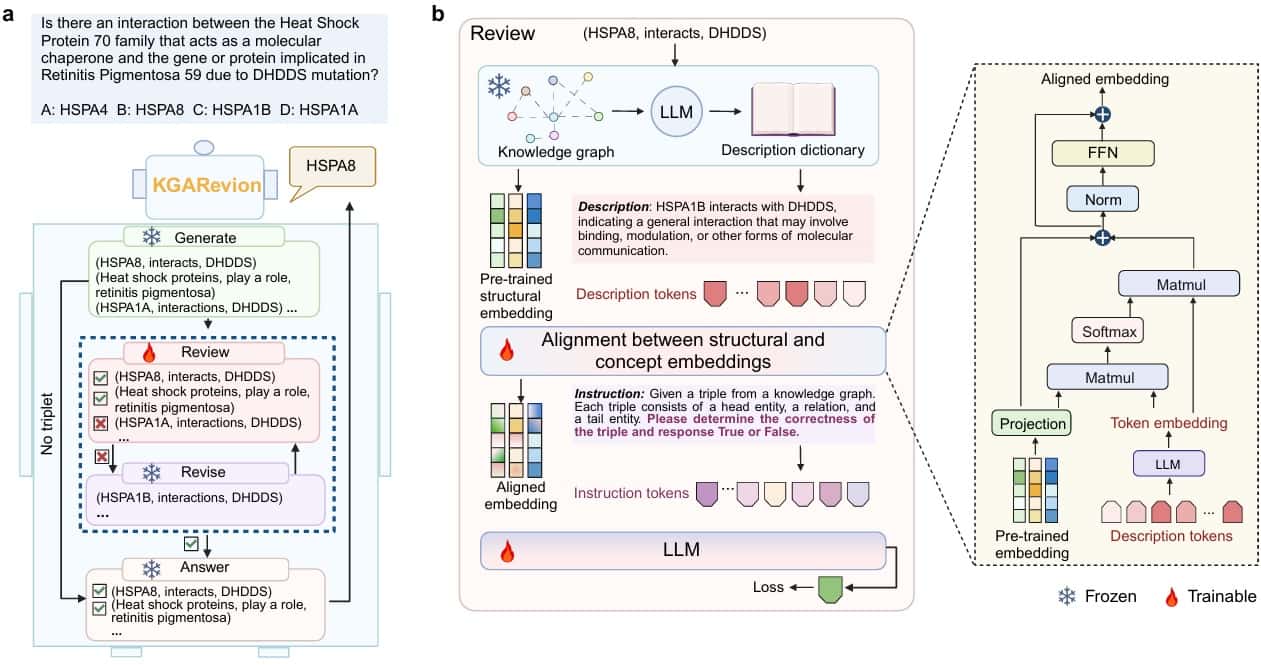
action, where embeddings get from KGs are structural embeddings, while concept embeddings from
LLMs (Credit: Su et al., “Knowledge Graph Based Agent for Complex QA,” arXiv.org, 2024.)
Overcoming Challenges in Knowledge Representation
One of the core challenges in deploying AI for medical QA is ensuring the system’s ability to process incomplete or ambiguous data. KGAREVION addresses this by leveraging the inferencing capabilities of knowledge graphs. These graphs enable the system to identify indirect relationships between medical entities, filling knowledge gaps that could hinder traditional models. For example, if direct links between symptoms and rare diseases are missing, KGAREVION can infer potential connections based on related pathways or genetic markers. This inferencing capability makes the system particularly adept at tackling rare or poorly understood conditions, offering clinicians valuable insights even in the face of limited data.
Expanding the Utility of Knowledge Graphs
Another advantage of KGAREVION lies in its capacity to integrate evolving medical research. The field of biomedicine is dynamic, with new discoveries and treatments emerging continuously. By incorporating updated knowledge graphs, KGAREVION remains current, adapting its reasoning to include the latest advancements. This dynamic adaptability ensures that clinicians and researchers have access to the most recent information, enhancing decision-making processes and fostering innovation in medical care. Furthermore, the modular design of knowledge graphs allows for specialized expansions, such as oncology-specific graphs or region-focused medical datasets, making KGAREVION versatile across various medical domains.
Transforming Healthcare: The Impact of KGAREVION
The broader implications of KGAREVION’s advancements extend far beyond medical QA. By integrating structured, domain-specific knowledge into AI, this system offers transformative potential for diagnostics, treatment planning, and patient-specific insights. For example, envision an AI capable of cross-referencing a patient’s medical history with the latest research findings in real-time, providing clinicians with personalized recommendations and risk assessments. Such capabilities could revolutionize patient care, enabling faster, more informed decision-making and reducing diagnostic errors.
Moreover, KGAREVION’s success underscores the growing importance of knowledge graphs in advancing AI’s capacity to address specialized, knowledge-intensive domains. As these graphs expand, their integration with AI systems like KGAREVION could become indispensable, offering tools that empower healthcare professionals to navigate the ever-growing landscape of medical information efficiently.
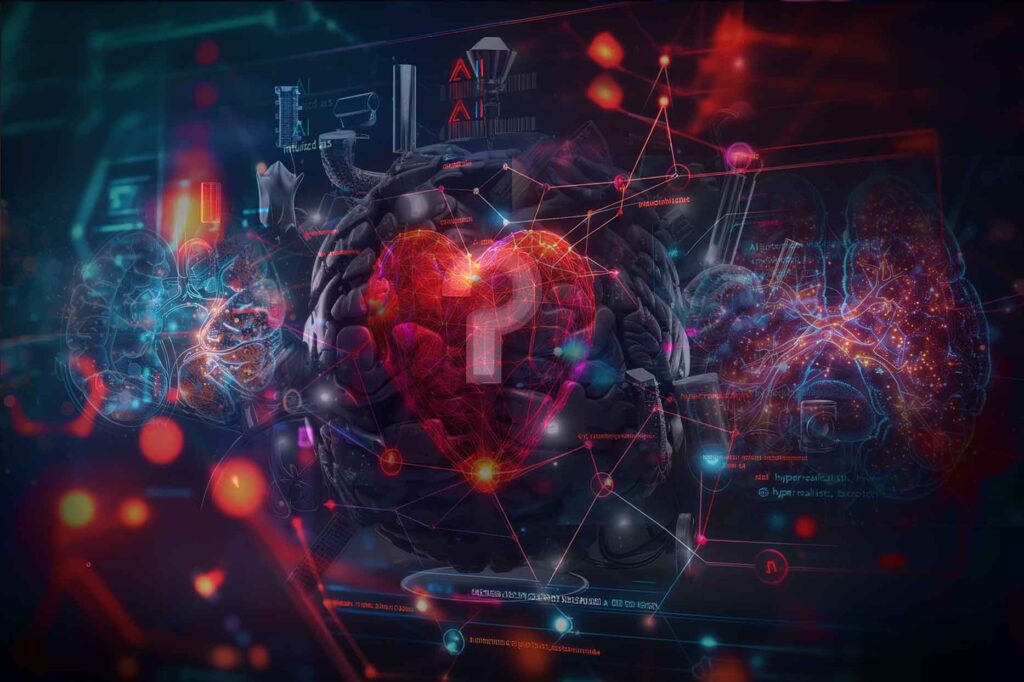
Ethical and Practical Considerations
As promising as KGAREVION’s capabilities are, its deployment raises important ethical and practical considerations. The reliance on knowledge graphs means that the accuracy and fairness of the system depend heavily on the quality of the underlying data. Biases in medical literature or incomplete datasets could inadvertently influence recommendations. To mitigate these risks, researchers advocate for transparent curation processes and regular audits of the knowledge graphs used. Additionally, there are practical challenges related to computational costs and the need for interdisciplinary collaboration to ensure the system’s efficacy and reliability in real-world settings.
Conclusion
KGAREVION represents a significant leap forward in the application of AI to medicine. By integrating knowledge graphs with cutting-edge AI techniques, it demonstrates how structured data can enhance the reliability and accuracy of medical QA systems. This multi-step verification process captures the intricacies of medical reasoning, providing robust, context-aware solutions where traditional models fall short. As further developments unfold, KGAREVION’s framework could inspire broader applications in healthcare, ultimately solidifying AI’s role as a trusted partner in complex medical fields. Through such advancements, we edge closer to a future where AI-driven insights not only complement but elevate the capabilities of clinicians, ensuring better outcomes for patients worldwide.
References
Su, Xiaorui, et al. “Knowledge Graph Based Agent for Complex, Knowledge-Intensive QA in Medicine.” arXiv.org, October 7, 2024. https://doi.org/10.48550/arXiv.2410.04660.
Let us know your thoughts! Sign up for a Mindplex account now, join our Telegram, or follow us on Twitter.
1 Comments
One thought on “Revolutionizing Medical QA: The Impact of Knowledge Graph Agents in Biomedical AI”
KGAREVION is an innovative AI system that improves medical question-answering by combining large language models with knowledge graphs for more accurate and context-aware responses. It enhances decision-making and patient care but requires addressing challenges like data bias and computational costs.
🟨 😴 😡 ❌ 🤮 💩