Solving aging – is AI all we need? Should resources be diverted away from biotech in order to accelerate the advent of god-like AI?
Dec. 02, 2024.
22 mins. read.
14 Interactions
There are two competing strategies for how aging should be solved: one focusing on AI first, one to develop biological therapies now. What’s your pick?
Love, attention, and scale
In June 1967, the Beatles premiered a glorious new song: All you need is love. The performance was the UK’s contribution to what was the world’s first global satellite television broadcast, and was simultaneously watched by over 400 million people in 25 different countries. The broadcast occurred during what became known as the Summer of Love, and the song became a powerful anthem of flower power.
The Beatles’ manager Brian Epstein had described the performance as the band’s finest moment, but it turned out that singing “all you need is love” wasn’t quite enough to bring about a world of peace and harmony.
Almost exactly 50 years later, a group of eight researchers at Google were searching for a title for an article they were about to publish. They settled on “Attention is all you need” – the title being the brainchild of the only Briton on the team, Llion Jones, who had grown up in north Wales, not far from Liverpool, the home of the Beatles. The article has attained legendary status within the global AI community, for its introduction of the transformer technology that underpins breakthrough AI initiatives such as ChatGPT.
Despite omitting architectural features that were previously thought to be essential for many text-based processing tasks, transformers excelled in these same tasks. The key innovation, which was to pay special attention to whichever parts of the input appeared most salient, turned out to give these AI systems a strong competitive advantage. The Attention is all you need paper correctly predicted that transformers could handle not just text but also other kinds of data, including pictures and sounds.
How far might transformers take AI? A third claim has increasingly been heard: “Scale is all you need”. Feed transformer systems ever larger amounts of data, and provide them with ever more powerful computer chips to crunch all that data into models with ever greater numbers of parameters, and there’s no limits to the degree of intelligence that can result. The “scale is all you need” hypothesis looks forward to AIs with fully general reasoning capabilities by doing more of the same.
In this context, I want to examine yet another “all you need” hypothesis. It’s a hypothesis that is already changing investment decisions and personal career trajectories. It’s the hypothesis that, whatever major problem you hope to solve, the best way to solve it is to start by creating general intelligence.
In this way of thinking, AI is all you need. An AI with god-like abilities will be able to race ahead of slow-witted humans to solve all the fundamental problems of science, medicine, and human existence.
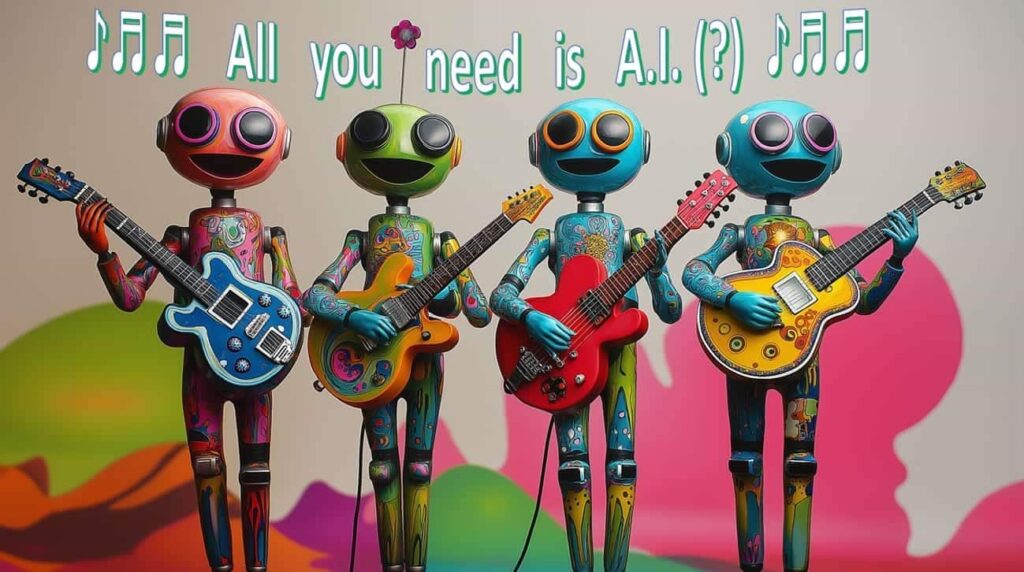
Machines of loving grace
The same thought is expressed in the recent provocative essay by the founder and CEO of Anthropic, Dario Amodei: Machines of Loving Grace – How AI Could Transform the World for the Better.
Amodei states it as follows: “I think that most people are underestimating just how radical the upside of AI could be… My basic prediction is that AI-enabled biology and medicine will allow us to compress the progress that human biologists would have achieved over the next 50-100 years into 5-10 years”.
Amodei gives some examples of the discoveries that AI-enabled science could make:
- “Design of better computational tools like AlphaFold and AlphaProteo”
- “More efficient and selective CRISPR” (for gene-editing)
- “More advanced cell therapies”
- “Materials science and miniaturization breakthroughs leading to better implanted devices”
- “Better control over stem cells, cell differentiation, and de-differentiation, and a resulting ability to regrow or reshape tissue”
- “Better control over the immune system: turning it on selectively to address cancer and infectious disease, and turning it off selectively to address autoimmune diseases”.
Who wouldn’t like such a vision?
According to this logic, spending effort in the next few years to create AI with these capabilities is a better investment than spending the same effort to improve biology and medicine here and now.
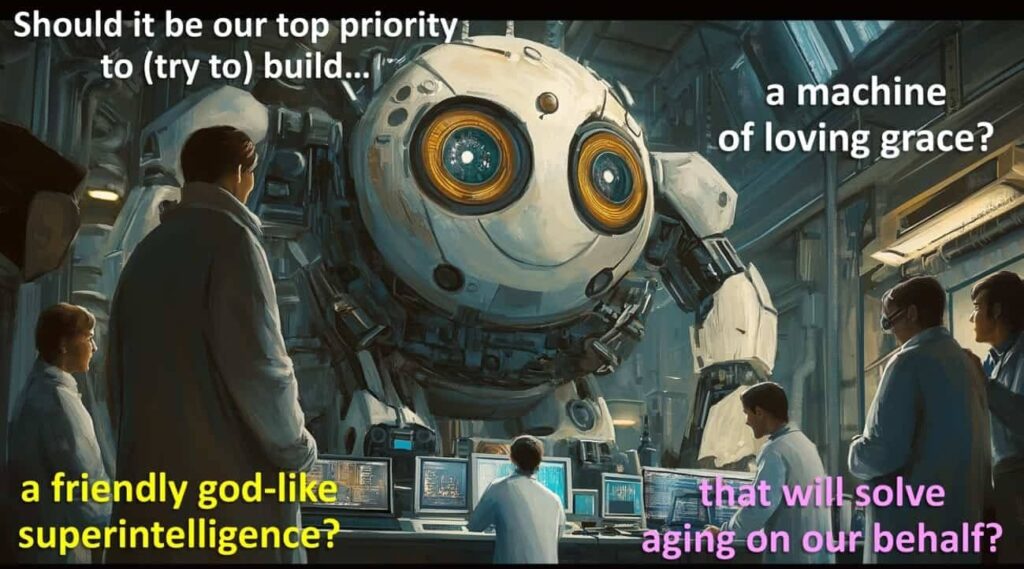
Funding is what marshals effort, and any funds at someone’s disposal should, it appears, be directed towards improving AI, rather than towards companies or foundations that are seeking to improve biology or medicine. Right?
A two-step mission statement
Back in February 2015, Demis Hassabis was relatively unknown. There had been a bit of press about the purchase of his company, DeepMind, by Google, for £400 million, but most people had little conception of what the company would accomplish in the following years.
Hassabis was giving a talk at CUTEC – the Cambridge University Technology and Enterprise Club. A photo from that talk is preserved on Reddit:
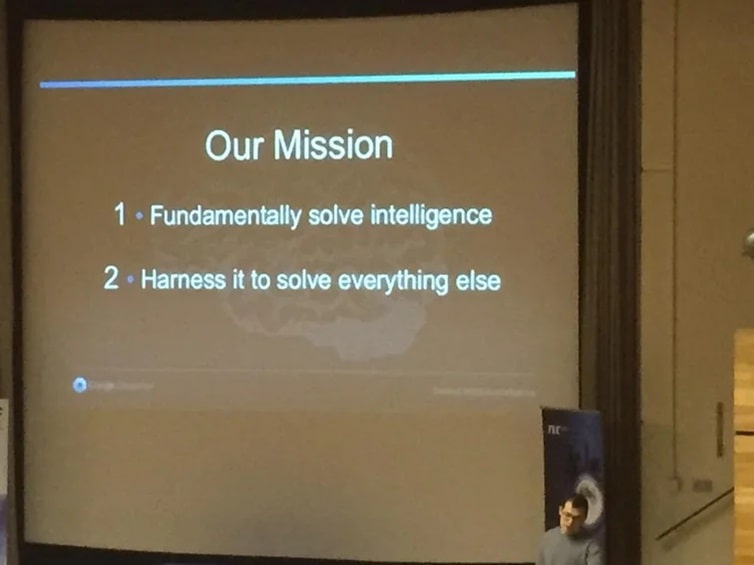
You can also read on that page on Reddit, from nearly ten years ago, some fascinatingly scathing comments about that mission statement:
- “Ridiculous and poorly-defined goals”
- “FFS [what] a mission statement [for] a company”
- “‘Fundamentally solve intelligence’ in the linked screenshot above is a whole load of nonsense”
- “I don’t even think we have a working definition for ‘intelligence’ yet. We don’t even know how it works in humans… How can we hope to recreate it before knowing what it is?”
But step forward to October 2024, with the announcement of the winners of this year’s Nobel Prize in Chemistry, “for computational protein design”. The mission statement outlined long ago for DeepMind now seems much more credible.
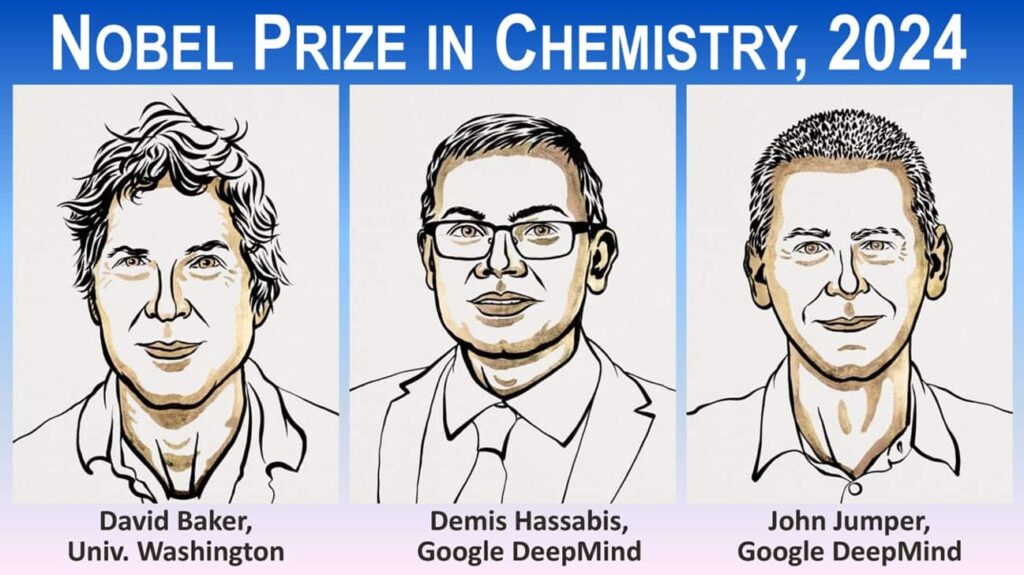
Once intelligence has been “fundamentally solved”, it should be relatively straightforward to solve climate change, economic distribution, cancer, dementia, and aging, right?
After all, given an AI model that can correctly predict how a long string of amino acids will fold up as a protein in three dimensions, won’t a scaled-up version of that model be able to predict other interactions between biochemical molecules – and, indeed, to predict how biological cells will respond to all kinds of proposed interventions?
The data bottleneck
One person striking a note of caution against exuberant forecasts of rapid additional progress about AI in medicine, was someone who shared the Nobel Prize with Demis Hassabis, namely David Baker of the University of Washington.
In an article published in MIT Technology Review shortly after the Nobel Prize, Baker pointed out that “AI needs masses of high-quality data to be useful for science, and databases containing that sort of data are rare”.
Indeed, the stunning success of DeepMind’s AlphaFold AI was fundamentally dependent on prior decades of painstaking work by numerous scientists to assemble what is known as PDB – the protein data bank.
The third of the joint winners, John Jumper of DeepMind, acknowledged this dependency in a press conference after the prize was announced. Jumper said, “I also want to really thank the giants on whose shoulders we stand, I think the entire experimental community, the people that developed the ability to measure protein structures, especially to Helen Berman and other pioneers of the Protein Data Bank, the PDB, who had the foresight to put these data together to make it available”.
Helen Berman had pioneered the PDB from 1971. As she graciously commented in a recent interview, “I am a very lucky person to have had an idea as a student, pursued that idea for more than 50 years, and then seen brand new science emerge for which three people have won this year’s Nobel Prize. It is really gratifying”.
Remarkably, Berman’s interest in protein folding predates even the Beatles song. In an online living history memoir written in 2012, Berman notes “In 1966 …I became fascinated by the world of protein folding. As part of my Ph.D. qualifier, … I proposed to perform structure-based sequence comparisons of known proteins…”.
Progress in determining protein structures was slow, for a long time, before becoming faster. This slide from a 2009 presentation by Berman that graphs the growth in the total number of proteins documented in PDB will look familiar to anyone familiar with singularitarian ideas:
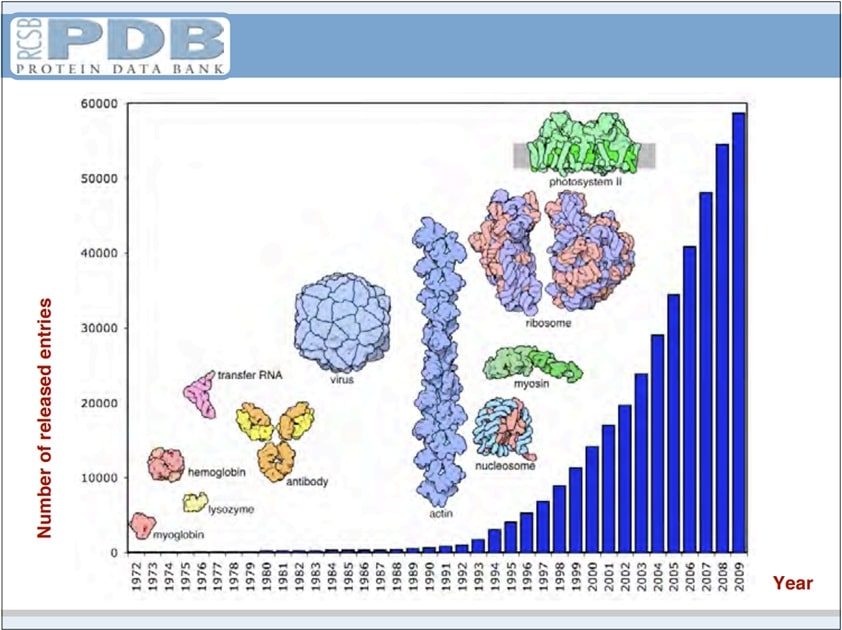
In the MIT Technology Review article, ‘A data bottleneck is holding AI science back’, David Baker pointed out that “If the data that is fed into AI models is not good, the outcomes won’t be dazzling either. Garbage in, garbage out”.
The subtitle of that article says it straightforwardly: “AI’s usefulness for scientific discovery will be stunted without high-quality data”.
So, we can forget “AI is all we need”. Before we can develop an AI that can solve aging for us, we will need to obtain suitable data on which that AI can be trained. We’ll need the equivalent of PDB for all the interventions that might remove or repair the low-level biological damage that we call aging.
Unless, that is, the AI has a very special kind of superintelligence, which allows it to reach conclusions even in the absence of adequate data. Let’s turn to that option next.
AI Zero?
The AI which achieved worldwide renown in March 2016 by defeating human Go superstar Lee Sedol, namely AlphaGo, gained that ability by being able to study around 160,000 games played between expert-level human Go players. The design of that version of the AI utterly depended on learning which moves tended to be selected by the best human players in a wide variety of situations.
AlphaGo’s success against Lee Sedol was rightly celebrated, but what happened in the following year was arguably even more startling. As reported in an article in Nature in October 2017, a new version of the AI, dubbed “AlphaGo Zero”, was given no data from human games; nor did it receive any human feedback on moves it suggested. Instead, it started tabula rasa, knowing only the rules of the game, before proceeding to play itself 4.9 million times in just three days.
AlphaGo Zero new self-play algorithms proved sufficient to reach higher levels than the earlier version (sometimes called “AlphaGo Lee”) that played Lee Sedol. When AlphaGo Zero played 100 games against AlphaGo Lee, it won every single game.
A similar pattern can be observed in the progress of AIs that process text. The trend is to require less and less explicit human guidance.
Consider AIs that translate between two languages. From the 1950s onward, designers of these systems provided ever-larger numbers of rules about grammar and sentence structure – including information about exceptions to the rules. Later systems depended on AIs observing, by themselves, statistical connections in various matching sets of text – such as the official translations of materials from the European Parliament, the Canadian Parliament, and the United Nations.
Managers noticed that the statisticians tended to produce better results than linguists who toiled to document every jot and tittle of grammatical variations. Infamously, Frederick Jelinek, a lead researcher at IBM, remarked that “Every time I fire a linguist, the performance of the speech recognizer goes up”. Performance jumped up again with the adoption of deep neural networks from 2012 onward, with the translations now being accurate not only at the word-for-word level but also at the sentence-for-sentence level.
A final significant jump came when transformer-based AIs were adopted. (The word “transformer” had been chosen to reflect the ability of these systems to transform text from one language into another.) As mentioned earlier, transformers are powerful because their algorithms can work out the strengths of connections between different parts of text input by themselves; they don’t need these connections to be pointed out by humans.
Could something similar happen with medical AIs of the future? Could such an AI find sufficient reliable information in an ocean of less reliable data, and therefore propose what steps should be taken to solve aging?
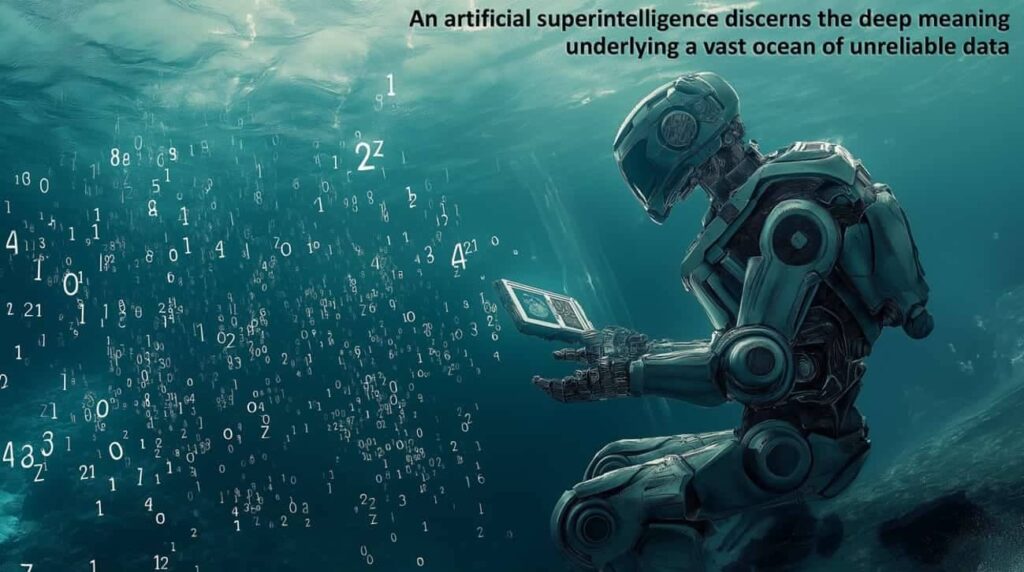
AI omniscience?
To recap: AlphaGo Lee needed detailed guidance from humans, before it could improve itself to superhuman level; but its successor, AlphaGo Zero, attained that level (and exceeded it) simply by power of its own vast intelligence.
Might it be similar with medical AI? Today’s AI medical systems are constrained by the extent of data, but might a future AI be able to work out all the principles of biology (including biology in which there is no aging) by starting tabula rasa (with a blank slate)?
All You Need Is Love said, “there’s nothing you can know that isn’t known” – the ‘all you need is AI’ approach would mean there’s nothing can be known it doesn’t know. Effectively, the AI would be omniscient.
Well, count me sceptical. It’s my view that some things need to be discovered, rather than simply deduced.
For example, why are there eight planets in our solar system, rather than thirteen? No principles of astronomy, by themselves, could determine that answer. Instead, the configuration of our solar system depends on some brute facts about the initial conditions under which the solar system formed. The only way to know the number of planets is to count them.
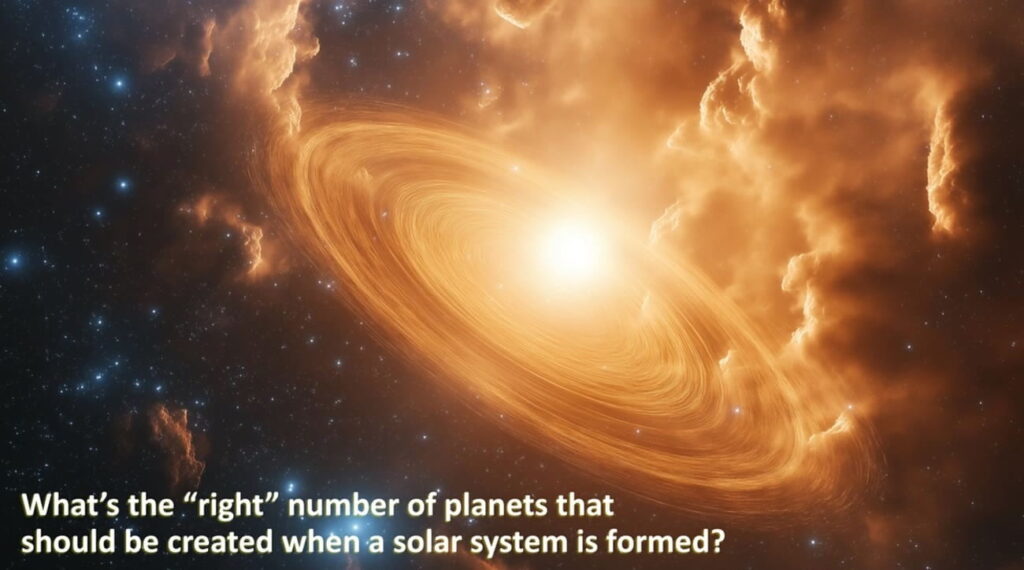
Again, why has life on our planet adopted a particular coding scheme, in which specific triplets of the nucleotides A, T, C, and G result in specific amino acids being formed? Why did homo sapiens lose the ability to synthesize vitamin C, or other genetic features which would be useful to us? Why are particular genes found on specific chromosomes? The only way to know which genes are located where is to look and see. No “AI Zero” is going to discover the answer by meditating in a void.
Therefore, I do not accept that “AI is all you need”. Data is also needed. That is, critical data.
This need is correctly recognized in the article Machines of Loving Grace by Dario Amodei, which I’ve already quoted. Amodei includes in the article “a list of factors that limit or are complementary to intelligence”. One of these items is “Need for data”.
Amodei comments: “Sometimes raw data is lacking and in its absence more intelligence does not help. Today’s particle physicists are very ingenious and have developed a wide range of theories, but lack the data to choose between them because particle accelerator data is so limited. It is not clear that they would do drastically better if they were superintelligent – other than perhaps by speeding up the construction of a bigger accelerator.”
AI as Principal Investigator?
Amodei offers a bold solution to this lack of data: “The right way to think of AI is not as a method of data analysis, but as a virtual biologist who performs all the tasks biologists do, including designing and running experiments in the real world (by controlling lab robots or simply telling humans which experiments to run – as a Principal Investigator would to their graduate students), inventing new biological methods or measurement techniques, and so on.”
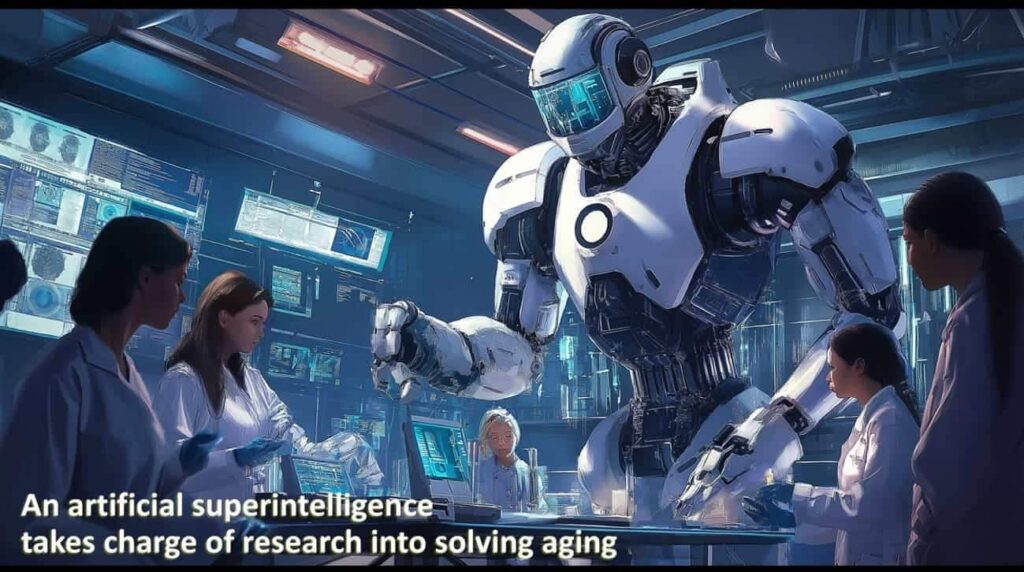
Amodei adds: “It is by speeding up the whole research process that AI can truly accelerate biology.”
He continues: “I want to repeat this because it’s the most common misconception that comes up when I talk about AI’s ability to transform biology: I am not talking about AI as merely a tool to analyze data. …I’m talking about using AI to perform, direct, and improve upon nearly everything biologists do.”
Amodei highlights the power of intelligence to transcend the limitations of its data: “You might believe that technological progress is saturated or rate-limited by real world data or by social factors, and that better-than-human intelligence will add very little. This seems implausible to me – I can think of hundreds of scientific or even social problems where a large group of really smart people would drastically speed up progress, especially if they aren’t limited to analysis and can make things happen in the real world”. Replace the “large group of really smart people” by an artificial superintelligence, and Amodei expects progress in science to rocket forward.
It’s an attractive vision, and I urge everyone to read Amodei’s entire essay carefully. (It covers many more topics than I can address in this article.)
But in case anyone is inclined to deprioritize existing research into promising lines of rejuvenation biotechnology, I have four remaining concerns: three negative and one strongly positive.
Three concerns and a huge opportunity
My first concern is that the pace of progress in AI capabilities will significantly slow down. For example, the data scaling laws may hit an impasse, so that applying more data to train new AI systems will fail to create the kind of superintelligence expected.
Personally I think that such a “wall” is unlikely, especially since AI developers have many other ideas in mind for how AI could be improved. But the possibility needs to be considered.
Second, it’s possible that AI capabilities will continue to surge ahead, but the resulting AI systems get involved in catastrophic harm against human wellbeing. In this scenario, rather than the AI curing you and me of a fatal condition – aging – it will cause us to die as a side-effect of a bad configuration, bad connectivity to fragile global infrastructure, an alien-like bug in its deep thinking processes, or simple misuse by bad actors (or naïve actors).
The leaders of the corporations which are trying to create artificial superintelligence – people like Demis Hassabis, Dario Amodei, Sam Altman, Elon Musk, Ben Goertzel, and a number of Chinese counterparts – say they are well aware of these dangers, and are taking due care to follow appropriate safety processes. But creating artificial superintelligence is an intensely competitive race, and that risks corners being cut.
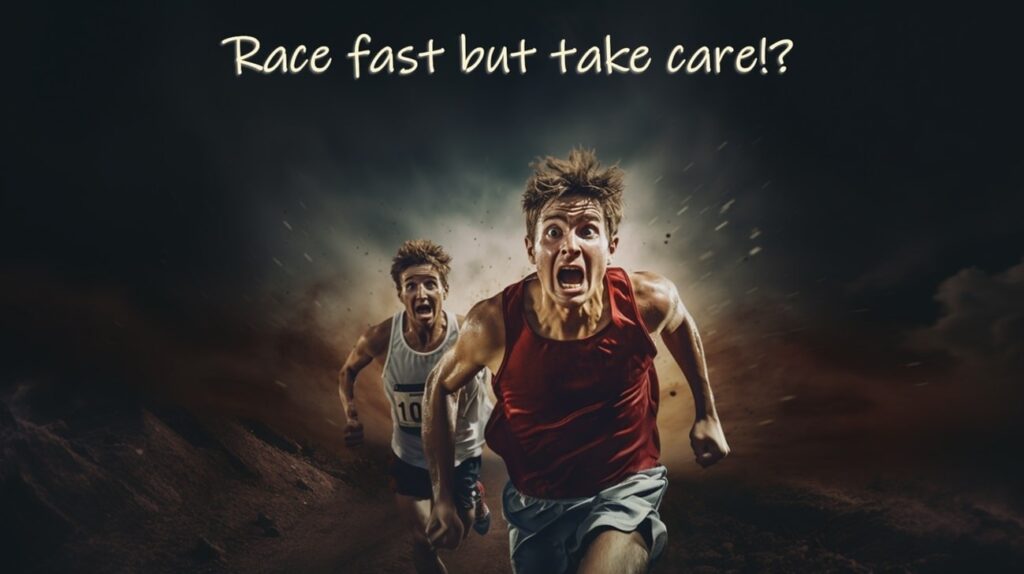
Third, the public may, very reasonably, demand more safeguards against the kind of suicide race just depicted. Specifically, an agreement might be reached by the USA and China, with the support of many other countries, that all progress towards artificial superintelligence should be blocked.
This agreement, with appropriate monitoring and enforcement mechanisms, would have the same effect as in the first concern above: AI progress hits a wall. But this time, it will be a wall imposed by regulations, rather than one intrinsic to the engineering of AI.
Some critics have responded that the chances are very slim for such an agreement to be reached and adopted. However, I disagree. That’s on account of both a stick and a carrot.
The stick is the growing public awareness of the catastrophic risks that new generations of AI bring. (That awareness is still on the slow part of the exponential growth curve, but may well accelerate, especially if there is a scandalous disaster from existing AI systems, something like an AI Chernobyl.)
The carrot is a clearer understanding that all the benefits we want from artificial superintelligence can also be obtained from an AI with humbler powers – an AI that:
- Is only modestly more capable than today’s best AIs
- Lacks any possibility to develop autonomy, sentience, or independent volition
- Will remain a passive, safe, but incredibly useful tool.
In a moment, I’ll say more about this huge opportunity. But first, let me interject an analogy about the choices facing humanity, as we contemplate how we might manage AI.
Peaceful progress or violent overthrow?
“Tear down the barricades!”
“Expropriate the expropriators!”
“Lock up the élites!”
“String up the capitalists!”
“Overthrow the ruling class!”
Such are the calls of revolutionaries in a hurry. However, the lesson of history is that violent revolutions tend to end up “devouring their own children” – to quote a phrase spoken by Jacques Mallet du Pan (referring to the French Revolution sending its original leaders to the guillotine) and also by former Hitler loyalist Ernst Röhm.
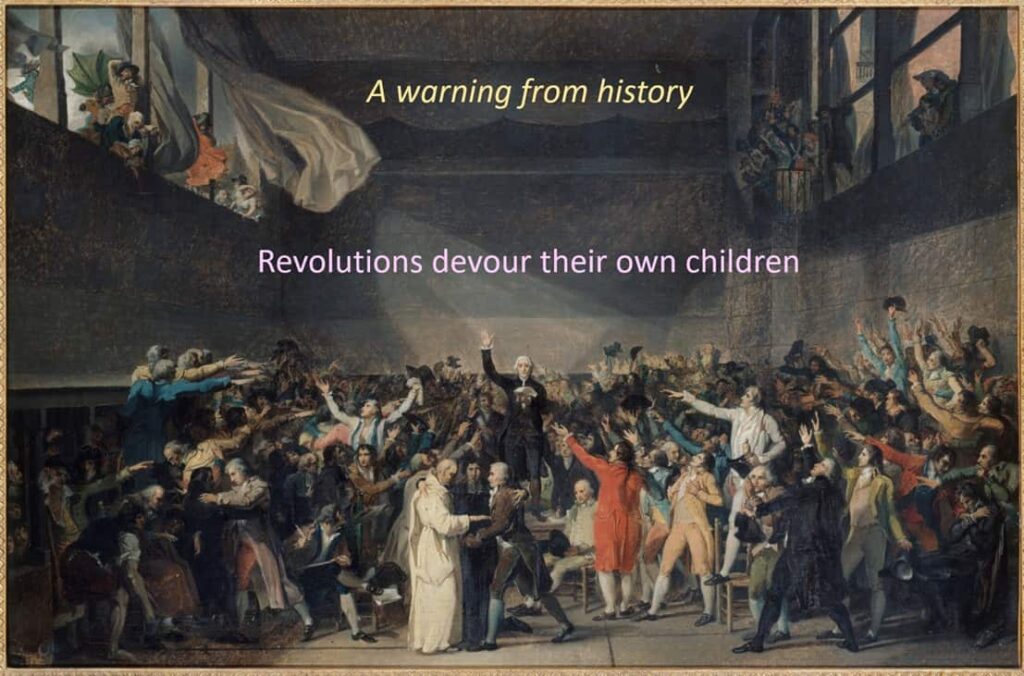
Similar remarks could have been uttered by many of the one-time supporters of Vladimir Lenin or Joseph Stalin, who subsequently found themselves denounced and subject to show trials.
However, the saying is not entirely correct. Some revolutions avoid subsequent internal bloodbaths: consider the American Revolutionary Wars of Independence, and the Glorious Revolution of 1689 in England.
Revolutionaries must uphold principle ahead of power-seeking, maintain a clear grip of reality (rather than becoming lost in self-deception), and continue to respect wise process (rather than allowing dictatorial leaders to do whatever they please) – in such cases, a revolution can lead to sustained progress with increased human flourishing.
Now consider the difference between what can be called “democratic socialists” and “Marxist-Leninists”. The former highlight ways in which the plight of the working class can be alleviated, stage by stage, through gradual societal reform. The latter lose patience with such a painstaking approach, and unleash a host of furies.
In case it’s not clear, I’m on the side of the democratic socialists, rather than the would-be revolutionaries who make themselves into gods and absolute arbiters.
For how humanity chooses to develop and deploy AI, I see the same choice between “harness accelerationists” and “absolute accelerationists”.
Harness accelerationists wish to apply steering and brakes, as well as pressing firmly on the throttle when needed.
Absolute accelerationists are happy to take their chances with whatever kind of AI emerges from a fast and furious development process. Indeed, the absolute accelerationists want to tear down regulation, lock up safety activists, and overthrow what they see as the mediocrity of existing international institutions.
Once again, in case it’s not clear, I’m on the side of harnessing acceleration. (Anyone still on X aka Twitter can see the “h/acc” label in my name on that platform.)
Harnessing requires more skill – more finesse – than keeping your foot pressed hard to the floor. I understand why absolute accelerationists find their approach psychologically comforting. It’s the same appeal as the Marxist promise that the victory of the working class is inevitable. But I see such choices as being paths toward humanitarian catastrophe.
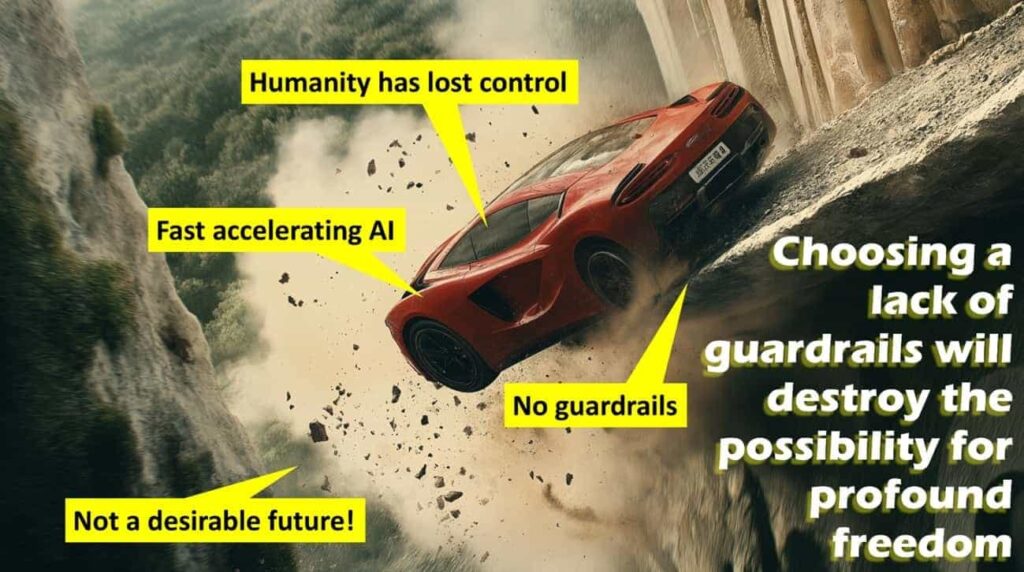
Instead, we can proceed quickly to solving aging, without awaiting the emergence of a hopefully benevolent god-like AI.
Solving aging – without superintelligence
Above, I promised three concerns and one huge opportunity. The opportunity is that it’s pretty straightforward to solve aging, without waiting for a potentially catastrophically dangerous artificial superintelligence. There are low-hanging fruits which aren’t being picked – in part because funding for such projects is being diverted instead to AI startups.
Aging occurs because the body’s damage-repair mechanisms stop working. Our metabolism runs through countless biochemical interactions, and low-level biological damage arises as a natural consequence – due to injuries inflicted by the environment, bad lifestyle choices, the inevitable side-effects even of good lifestyle choices, or (perhaps) because of programmed obsolescence. When we are young, lots of that damage is routinely repaired or replaced soon after it occurs, but these replacement and repair mechanisms lose their effectiveness over time. The consequence is that our bodies become more prone to all sorts of disease and infirmity. That’s aging.
The most promising path to solving aging is to comprehensively reinforce or complement these damage-repair mechanisms. The low-hanging fruit is that we have a long list of ways this might be achieved:
- By taking inspiration from various animal species in which at least some of the damage-repair mechanisms are better than in humans
- By understanding what’s different about the damage-repair mechanisms in ‘human superagers’
- By designing and applying new interventions at the biotech or nanotech levels.
To be clear, this does not mean that we have to understand all of human biological metabolism. That’s horrendously complicated, with numerous side-effects. Nor do we even need to understand all the mechanisms whereby damage accumulates. Instead, we just need to observe, as engineers, what happens when new damage-repair mechanisms are applied in various animals.
These mechanisms include senolytics that clean up senescent cells (sometimes called “zombie cells”), extending telomeres at the ends of chromosomes, reversing some of the epigenetic alterations that accumulate on our DNA, introducing specially programmed new stem cells, nanoparticles which can break-up accumulated plaques and tangles, re-energising the mitochondria within our cells – and much more.
In each case, some useful research is being done on the viability of introducing these repair mechanisms. But nothing like enough.
We especially need tests of the long-term effects of damage-repair mechanisms, especially applied in combination. These tests can determine something that even an artificial superintelligence would find difficult to predict by meditating in a void: which damage-repair interventions will positively synergize with each other, and which ones have antagonistic effects.
These are the kind of tests being pursued by one organisation where I need to declare an interest: the Longevity Escape Velocity Foundation (LEVF), where I have a role on the leadership team, and whose underlying ideas I have supported for nearly 20 years since first coming across them in meetings of what was the forerunner of London Futurists.
LEVF is carrying out a number of extended projects on large numbers of mice, involving combining treatments that have already been proven to individually extend the lifespan of mice treated from middle age. Interim results of the first such project, RMR1, can be reviewed here (RMR = Robust Mouse Rejuvenation), and plans for the second one, RMR2, have been posted here.
Rather cheekily, may I suggest that the 1967 slogan of the Beatles, All you need is love, got two letters wrong in the final word?
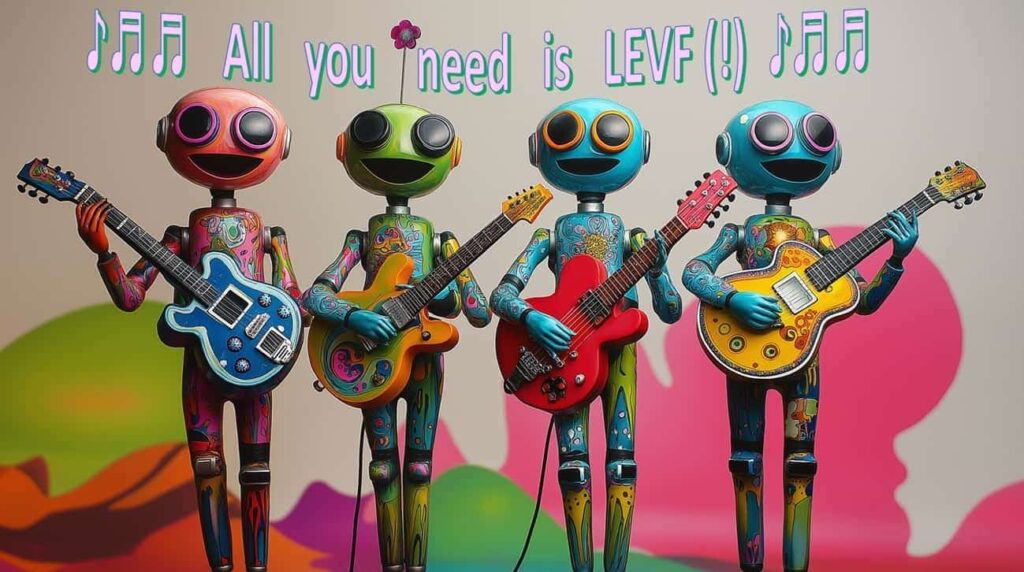
Two scenarios for trying to solve aging
To conclude, I envision two competing scenarios ahead, for how aging should be solved:
- An “AI first” strategy, in which important research into rejuvenation biotechnology is starved of funding, with money being preferentially allocated to general AI initiatives whose outcomes remain deeply uncertain.
- A “damage-repair research now” strategy, in which projects such as RMR2 receive ample funding to proceed at pace (and, even better, in multiple different versions in parallel, including in animals larger than mice), with the data produced by such experiments then being available to train AIs which can complement the ingenuity of pioneering human researchers.
What’s your pick?
Let us know your thoughts! Sign up for a Mindplex account now, join our Telegram, or follow us on Twitter.
3 Comments
3 thoughts on “Solving aging – is AI all we need? Should resources be diverted away from biotech in order to accelerate the advent of god-like AI?”
But if you consider the time it took us to achieve what we have achieved before AI and then after AI, there is a significant difference that indicates the time span after AI is shorter. Though all the points in your article are valid, I think the world will choose the AI path because it is the shorter path.
🟨 😴 😡 ❌ 🤮 💩
David, you are becoming one of my favorite writers on Mindplex. I am usually known for my long comments, but in this one, you have said it all. Thank you!
🟨 😴 😡 ❌ 🤮 💩
As a general approach to betting on things amid uncertainty, I'd say the following: Create a convex payoff with limited downside and unlimited upside — build up optionality. It might be prudent to account for what could be called 'single event dominance,' where any of the alternatives may lead to overwhelming success and make all the difference. Thus, as we don't know which path is the best, it is better to place some fuel in all of them. Further, according to this philosophy, extra risks with little added potential are unnecessary and should be avoided. X-risks definitely fall into that category.
Importantly, there is a lot of value in creating tools by which several different entities can think about this question independently, with access to proper information, and determine which paths are already sufficiently resourced (in their view) and invest where they see the expected marginal utility to be the highest. Of course, after that, it comes to the question of information and value systems to keep collective track of value creation, but that's already a different (yet fascinating) topic.
As a final point, I don't completely agree with you regarding regulation and the reliability of international institutions. I can't see how public opinion can effectively channel into these kinds of questions in the West, let alone in China, for example. With this context, investing some effort to improve democratic processes, rebuild those institutions, and find ways to increase educated participation might have a surprisingly high payoff.
And now I can't resist mentioning that tomorrow, 4.12., there begins a Constitutional Convention as part of the process where liquid democracy is combined with a constitutional republic for the first time in human history, as far as I know.
And as the very final point, the view you presented about working on aging in practice is very reasonable and makes a lot of sense. For example, exploring hyperbarics combined with stem cell therapies is indeed low-hanging fruit. No ASI is needed for that.
Thanks for the article! It was an enjoyable read.
And thanks for (and letting me know about) the LEVF!
🟨 😴 😡 ❌ 🤮 💩