Tyche: A Breakthrough in Medical Image Segmentation with Stochastic In-Context Learning
Jul. 19, 2024. 4 mins. read.
30 Interactions
MIT has introduced Tyche, a pioneering framework for medical image segmentation that leverages stochastic learning to enhance diagnostic accuracy and reduce the need for retraining, transforming healthcare practices.
Introduction
Medical image segmentation is a critical task in healthcare, providing essential information for diagnosis and treatment planning. Traditional methods in this domain often suffer from significant limitations, including the need for retraining models for new tasks and the production of deterministic outputs that fail to capture medical image ambiguities. Researchers at MIT have made a substantial leap forward with the introduction of Tyche, a novel framework that leverages stochastic in-context learning to address these challenges.
Addressing Core Challenges in Medical Image Segmentation
The segmentation of medical images involves identifying and delineating structures within various imaging modalities like MRI, CT scans, and ultrasounds. Existing models typically require retraining for new segmentation tasks, a process demanding considerable computational resources and domain expertise. Moreover, these models generally produce a single, deterministic result, which does not account for the inherent variability and ambiguity in medical images.
Introducing Tyche: An Innovative Framework
Tyche is designed to overcome these limitations by integrating probabilistic segmentation with an in-context learning framework. The framework operates with two primary variants:
- Tyche-Train-time Stochasticity (Tyche-TS): This variant is trained to produce multiple segmentation candidates during the training phase. It learns the distribution of possible labels and generates diverse segmentations by allowing interactions among different predictions.
- Tyche-Inference-time Stochasticity (Tyche-IS): This utilizes a deterministic model trained traditionally and employs test-time augmentation during inference to produce varied segmentations without the need for retraining.
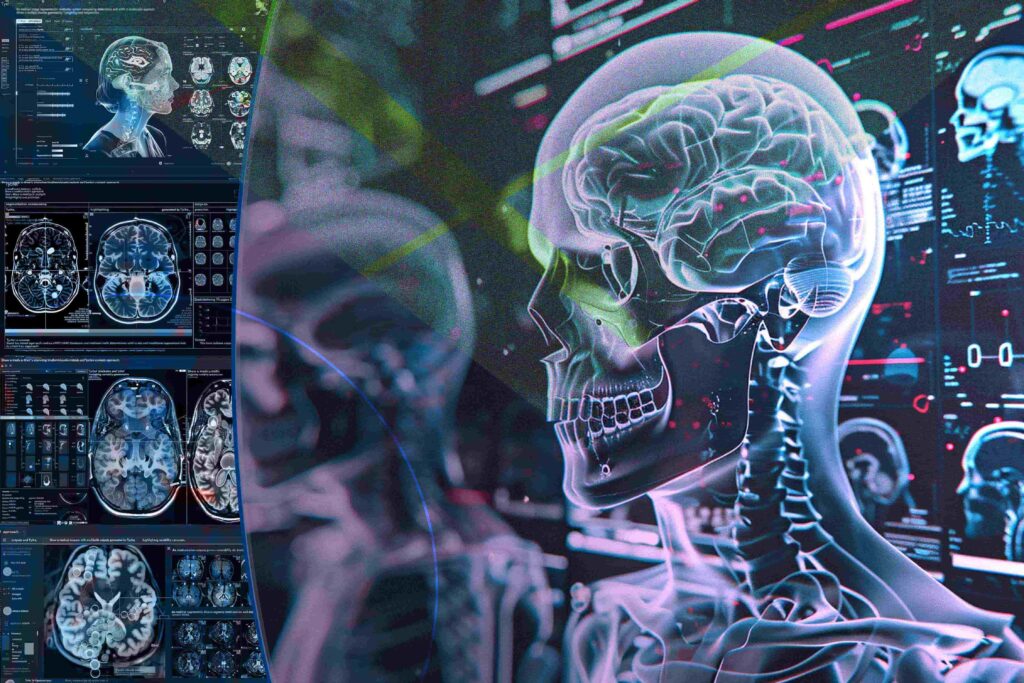
Methodology and Technical Innovations
The Tyche framework requires an image to be segmented and a contextual set of image-segmentation pairs that define the task. Tyche-TS incorporates stochastic elements during training to encourage diversity in segmentation predictions. This is achieved using the innovative SetBlock mechanism, which integrates multiple predictions and introduces noise to foster diverse candidate outputs. In contrast, Tyche-IS generates multiple segmentation candidates during inference by applying augmentation techniques to both the target image and the context set. This approach effectively diversifies predictions using a pre-existing deterministic model, ensuring robust performance without additional training.
A key component of Tyche’s methodology is the Best Candidate Dice Loss, tailored to optimize the best prediction among the multiple candidates. This loss function drives the model towards generating a variety of plausible segmentations, thereby enhancing the flexibility and applicability of the framework.
Demonstrated Efficacy and Versatility
The researchers tested Tyche on twenty unseen medical imaging tasks, benchmarking its performance against existing methods. The results were impressive, with Tyche displaying superior performance when compared to both in-context learning baselines and interactive segmentation methods. Notably, Tyche’s results closely aligned with those produced by specialized stochastic models meticulously trained for specific tasks.
One of Tyche’s most significant advantages is its generalizability. The framework effectively handles images from datasets not encountered during training, outperforming other segmentation methods regarding metrics like the best candidate Dice score and Generalized Energy Distance (GED). This capability is particularly valuable in clinical settings where the diversity of tasks and image types is vast.
Practicality and Efficiency
Tyche’s two variants offer a well-balanced trade-off between computational efficiency and prediction quality. Both Tyche-TS and Tyche-IS have shown to be practical for clinical use, making them viable options for real-world medical imaging applications.
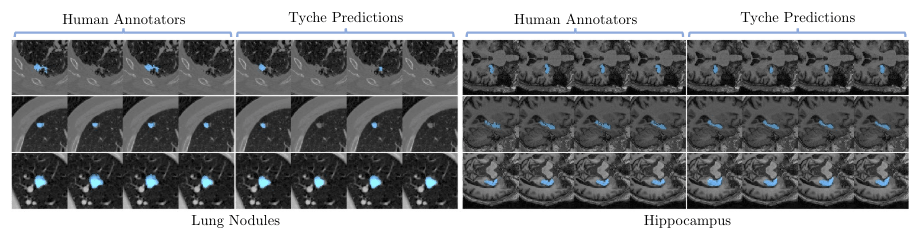
Future Directions
The advent of Tyche opens several exciting avenues for future research. Further exploration is needed to understand the types of uncertainty captured by its stochastic mechanisms. Additionally, expanding Tyche’s applicability to more complex support sets and various image modalities could broaden its impact significantly.
Conclusion
Tyche represents a transformative advancement in the field of medical image segmentation. By addressing the need for stochastic predictions and reducing the dependency on task-specific retraining, Tyche delivers robust, diverse, and practical solutions for medical professionals. Its ability to generalise across different tasks and datasets paves the way for more efficient and effective medical imaging applications, heralding a new era in medical diagnostics and treatment planning.
Reference
Rakic, Marianne, Hallee E. Wong, Jose Javier Gonzalez Ortiz, Beth Cimini, John Guttag, and Adrian Dalca V. “Tyche: Stochastic In-Context Learning for Medical Image Segmentation.” arXiv.org, January 24, 2024. https://arxiv.org/abs/2401.13650.
Code available at: https://github.com/mariannerakic/tyche
Let us know your thoughts! Sign up for a Mindplex account now, join our Telegram, or follow us on Twitter.
9 Comments
9 thoughts on “Tyche: A Breakthrough in Medical Image Segmentation with Stochastic In-Context Learning”
Tyche's approach to medical image segmentation really impressive. Its ability to make diverse predictions without needing constant retraining is a huge step forward
🟨 😴 😡 ❌ 🤮 💩
Tyche's novel approach revolutionizes medical image segmentation by integrating probabilistic elements, significantly improving prediction diversity and accuracy. It's a major step forward in enhancing the efficiency and reliability of medical imaging applications.
🟨 😴 😡 ❌ 🤮 💩
This could change how we do diagnostics and treatment planning. I see a lot of potential.
🟨 😴 😡 ❌ 🤮 💩
Tyche marks a significant step forward in medical image segmentation. By integrating stochasticity into the segmentation process, the framework addresses the limitations of traditional deterministic models and offers a more robust and adaptable approach. The ability to generate multiple segmentation candidates without extensive retraining is particularly valuable for clinical settings with diverse image data. Tyche's versatility and strong performance across various metrics underscore its potential to improve diagnostic accuracy and treatment planning. Further research into the generalizability of Tyche across different imaging modalities and clinical applications would be beneficial.
🟨 😴 😡 ❌ 🤮 💩
Impressive work! Tyche's innovative approach to medical image segmentation is a game-changer for improving diagnosis and treatment planning.
🟨 😴 😡 ❌ 🤮 💩
good, the article represents a positive and innovative advancement in medical technology.
🟨 😴 😡 ❌ 🤮 💩
it seems good technology to reduce medical errors that are occur by doctors by digitalizing reading of medical records
🟨 😴 😡 ❌ 🤮 💩
loved it..
🟨 😴 😡 ❌ 🤮 💩
The Tyche framework's stochastic in-context learning approach to producing diverse segmentation outputs is a game-changing innovation that could dramatically improve the reliability and adaptability of medical image analysis.
🟨 😴 😡 ❌ 🤮 💩