AI’s Linguistic Bias: A Silent Architect of Cultural Marginalization?
Dec. 03, 2024.
5 mins. read.
Interactions
Is AI subtly eroding cultural diversity? Explore how English-dominant processing in multilingual models challenges linguistic equity.
The potential benefits of artificial intelligence are huge, as are the dangers.
—Dave Waters
Introduction
Artificial intelligence (AI) is becoming deeply embedded in modern life, reshaping industries, communication, and even culture. Yet, beneath the surface of this technological marvel lies a concern that often goes unnoticed: the linguistic biases of multilingual large language models (LLMs) like Llama-2. These biases, especially their reliance on English as a latent processing language, could subtly erode linguistic diversity, marginalize non-English cultures, and inadvertently contribute to global cultural homogenization. This article highlights the mechanisms that lead to these biases and explores their far-reaching implications.
The English-Centric Lens
Multilingual LLMs such as Llama-2 are predominantly trained on datasets dominated by English, often comprising a vast majority of their training corpora. Despite this imbalance, they perform impressively across multiple languages. However, a closer look at their internal processing reveals an intriguing yet concerning mechanism: the use of English as a latent “concept space.”
A recent research tracking Llama-2’s embeddings through its layers demonstrates a three-phase progression. Initially, input data resides far from output embeddings in the model’s high-dimensional space. In the middle layers, the embeddings transition to an abstract conceptual representation that aligns more closely with English than with the input language. Finally, the embeddings adjust to output the appropriate language-specific tokens.
This mechanism is akin to a mental translator: an input sentence in Japanese might be internally represented as an English abstraction before being processed and rendered back into Japanese. While this process explains Llama-2’s robust multilingual capabilities, it reinforces an English-dominant perspective, skewing its linguistic neutrality and exacerbating cultural disparities.
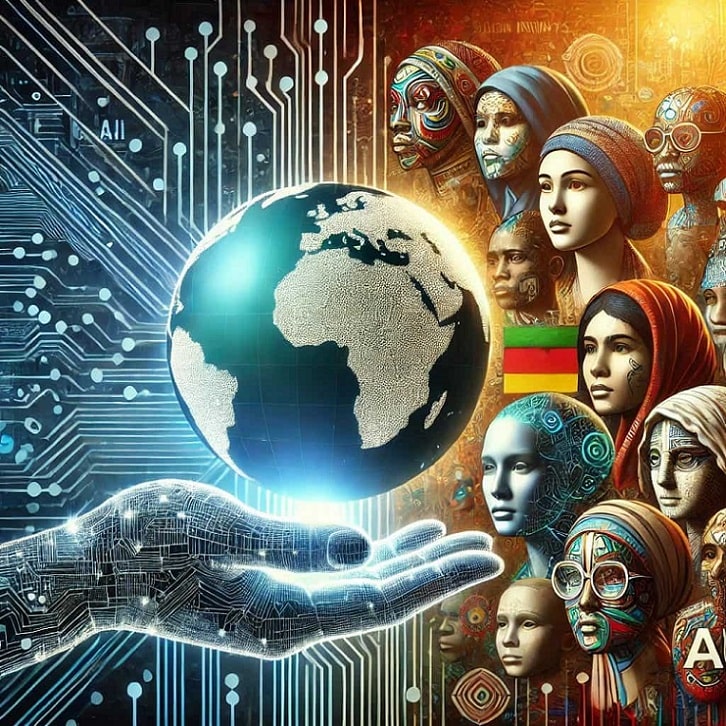
Lost in Translation: Bias in Translation and Representation
One practical implication of this bias lies in translation. For example, when encountering idiomatic phrases such as the Spanish “dar en el clavo” (to hit the nail or to to hit in the nail), Llama-2 might prioritize an English-centric generic equivalents and instead of translating it in a way that preserves the cultural context and imagery of the original Spanish, it might default to a more generic phrases like “to get it right”. This often results in translations that lose cultural nuance, oversimplifying or misrepresenting the richness of the original expression.
Such distortions extend beyond semantics. When AI operates predominantly through an English lens, it risks diluting the cultural essence embedded in language, particularly in contexts such as literature or oral traditions where word choice and phrasing hold deep cultural significance.
Cultural Implications: “Winners Write History”!
Linguistic bias in LLMs also has profound ethical implications. The phrase “winners write history” captures the historical tendency for dominant groups to shape narratives according to their perspectives. LLMs trained on English-dominated datasets may unknowingly perpetuate such biases, privileging dominant cultural viewpoints while marginalizing others.
Consider the role of multilingual AI in generating or summarizing historical content. The inherent reliance on English representations risks introducing subtle shifts in how historical events are framed, aligning them with English-speaking cultural narratives. Over time, such biases could influence collective memory, perpetuating inequalities in cultural representation and equity.
Ethical Considerations and the Path Forward
The findings from Llama-2’s study underscore the urgency of addressing linguistic biases in AI development. While the use of English as a latent processing language enhances generalization and cross-lingual tasks, it inadvertently prioritizes English-centric perspectives over others. This raises critical questions: How can we ensure AI systems are linguistically and culturally inclusive? And what steps can be taken to mitigate the risks of cultural homogenization?
Efforts must begin with the training datasets themselves. Diversifying training corpora to include more balanced representations of non-English languages is a crucial first step. Additionally, rethinking architectural designs to minimize reliance on a single dominant language during intermediate processing could help preserve linguistic diversity.
Conclusion
Generative AI and then LLMs holds unparalleled potential to bridge linguistic divides and democratize knowledge. Yet, as the case of Llama-2 reveals, it also risks perpetuating biases that reinforce cultural hierarchies and suppress diversity. As we continue to develop and deploy multilingual LLMs, the onus is on researchers, developers, and policymakers to ensure these systems promote inclusivity rather than cultural assimilation. Understanding the mechanics of linguistic biases is not just an academic exercise—it is essential to building an AI-powered future that respects and celebrates the world’s cultural diversity.
Reference
Wendler, Chris, Veniamin Veselovsky, Giovanni Monea, and Robert West. “Do Llamas Work in English? On the Latent Language of Multilingual Transformers.” arXiv.org, February 16, 2024. https://arxiv.org/abs/2402.10588.
Notes: For those of you who are interested to read the full paper, we have summarized it bellow as a starter.
- Core Question: The study investigates if multilingual models like Llama-2, trained predominantly on English data, exhibit an English-centric bias in their internal computations, even when handling non-English languages. This concern is significant for understanding inherent linguistic biases in these models.
- Findings:
- The research identified three distinct phases in how Llama-2 processes language inputs: (a) Initial input embedding, (b) transition through an abstract “concept space” closer to English, and (c) final token prediction specific to the input language.
- The “concept space” aligns more closely with English representations, indicating a potential English-centric intermediary processing stage.
- Methodology:
- The researchers used logit lens analysis to interpret the latent embeddings at various layers of the model. This method decodes token distributions at intermediate layers to understand language representation.
- To ensure clear results, they designed prompts with unambiguous continuations in multiple languages.
- Implications: The English-centric processing may influence multilingual performance and highlight potential biases that could affect applications in low-resource languages.
Let us know your thoughts! Sign up for a Mindplex account now, join our Telegram, or follow us on Twitter.
0 Comments
0 thoughts on “AI’s Linguistic Bias: A Silent Architect of Cultural Marginalization?”