The advancement of machine learning applications in various domains necessitates the development of robust frameworks that can handle large-scale data efficiently. To address this challenge, a paper titled “Implementing and Benchmarking a Fault-Tolerant Parameter Server for Distributed Machine Learning Applications” (which sounds like a mouthful but is a pretty simple concept once you break down the words) introduces a powerful Parameter Server Framework specifically designed for large-scale distributed machine learning. This framework not only enhances efficiency and scalability but also offers user-friendly features for seamless integration into existing workflows. Below, we detail the key aspects of the framework, including its design, efficiency, scalability, theoretical foundations, and real-world applications.
Key Features of the Parameter Server Framework
User-Friendly Interface
The framework allows easy access to globally shared parameters for local operations on client nodes, simplifying the complexities often encountered in distributed environments. A notable attribute of this framework is its focus on user accessibility, achieved through the streamlined implementation of asynchronous communication and the support for flexible consistency models. This design choice facilitates a balance between system responsiveness and rapid algorithm convergence, making it an attractive solution for practitioners and researchers alike.
Enhanced Efficiency
Efficiency is at the core of the framework’s design, leveraging asynchronous communication coupled with advanced consistency models like the “maximal delayed time” model and a “significantly-modified” filter. These features are crucial in enabling the system to converge to a stationary point under predetermined conditions. The framework’s asynchronous nature permits substantial improvements in processing speeds, effectively addressing the latency issues typically associated with large-scale data processing.
Scalability and Fault Tolerance
Designed to be elastically scalable, the framework supports dynamic additions and subtractions of nodes, thereby accommodating varying computational demands effortlessly. It also integrates fault tolerance mechanisms that ensure stable long-term deployment, even in the face of potential hardware failures or network issues. This level of reliability is essential for enterprises that depend on continual data processing and analysis.
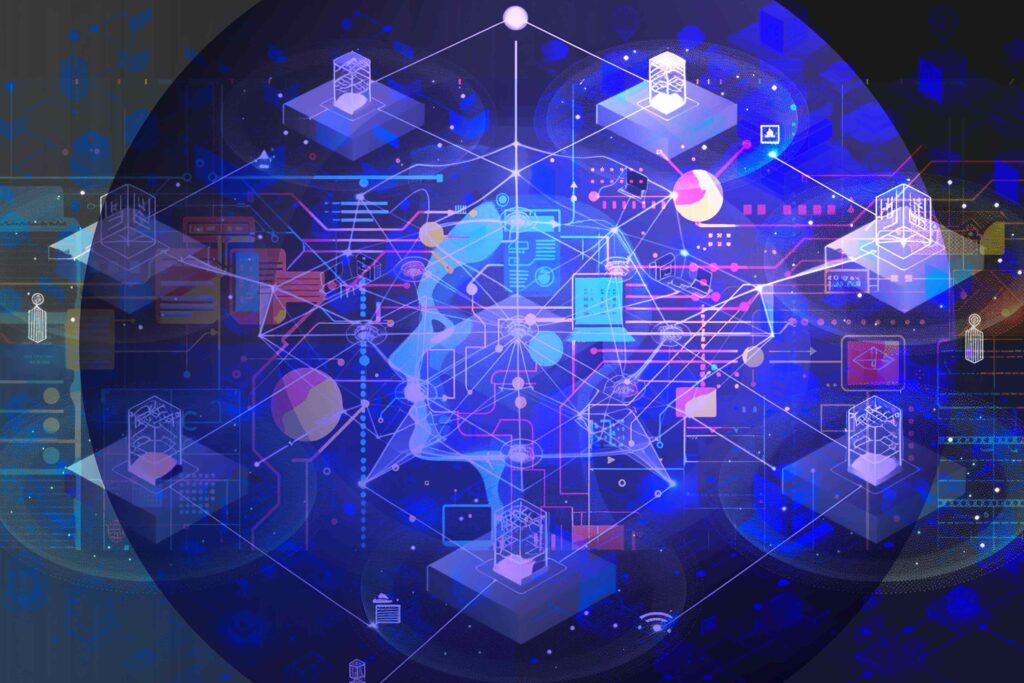
Applications and Theoretical Foundation
The Parameter Server Framework is not only practical but also grounded in solid theoretical principles. It supports complex optimization problems, including nonconvex and nonsmooth challenges, using proximal gradient methods. This theoretical backing is crucial for tasks such as risk minimization, distributed Gibbs sampling, and deep learning. The structure of the framework is designed around server nodes that manage globally shared parameters and client nodes that perform computations asynchronously, thus optimizing the workload distribution.
Implementation Details
Server Nodes: These nodes are responsible for managing global parameters efficiently.
Client Nodes: Client-side operations are executed asynchronously, enhancing overall system performance.
Experimental Validation
The framework has been tested on real-world datasets, including L1-regularized logistic regression and Reconstruction Independent Component Analysis (RICA), demonstrating its capability to handle complex, data-intensive tasks. The results show linear scalability with the increase in the number of client nodes, indicating a substantial speedup that validates the framework’s effectiveness in large-scale settings.
Conclusion
The Parameter Server Framework offers a sophisticated solution to the challenges of large-scale distributed machine learning. With its user-friendly interface, high efficiency, scalability, fault tolerance, and solid theoretical foundation, the framework is poised to significantly impact the field of machine learning. The experimental results underscore its practicality and effectiveness, making it an invaluable tool for researchers and practitioners aiming to leverage the full potential of distributed computing in machine learning.
Let us know your thoughts! Sign up for a Mindplex account now, join our Telegram, or follow us on Twitter.