Cooperative Learning: How Videos and Text Are Helping AI Understand the World
Sep. 30, 2024.
5 mins. read.
11 Interactions
What happens when AI combines sight and language? Explore how merging visual and textual data can revolutionize machine learning.
The field of artificial intelligence has made remarkable strides in recent years, but one persistent challenge remains: teaching machines to understand complex information from multiple sources. Researchers from Sakana AI recently explored this issue in their paper, “Cooperative Learning of Disentangled Representations from Video and Text.” They introduce a new approach that enables AI systems to learn by combining visual and textual data, offering new potential for improving how machines comprehend and process the world around them.
The Problem with Single-Source Learning
In most machine learning models today, AI systems are trained to recognize patterns using either video data or text data—but rarely both at the same time. While this method has led to great advances in image recognition and natural language processing, it has its limitations. When AI only learns from one source, it lacks the rich context that human perception naturally incorporates. For example, a machine might recognize a scene in a video, but it might not fully grasp the meaning without understanding the accompanying text or spoken language.
Disentangled Representations: A New Approach
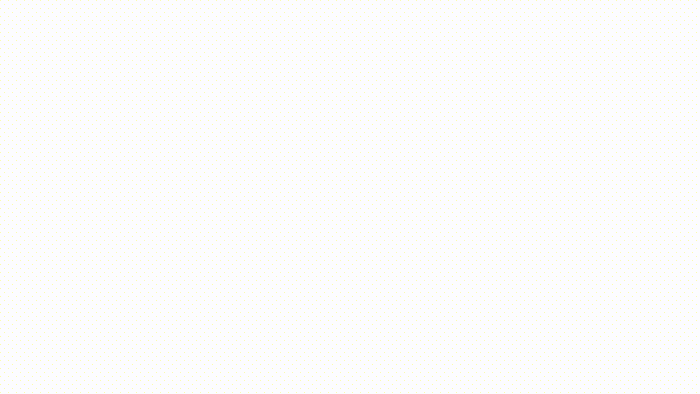
To overcome these limitations, the researchers propose a method called disentangled representation learning, where the AI system separates important factors from both videos and text. These factors might include objects in a scene, actions being performed, or the relationship between words and visuals. By disentangling these elements, the model can learn more effectively from both sources, capturing a more complete understanding of the world.
Specifically, disentangled representation learning helps in several ways:
- Separation of Key Factors: By isolating different elements such as objects in a scene, actions being performed, and the relationships between words and visuals, the AI can more clearly distinguish and analyze each component. This separation allows the model to focus on specific aspects of the data, leading to a more comprehensive understanding of each source.
- Enhanced Contextual Understanding: The method combines the visual and textual data in a way that integrates context. For example, understanding a video of a cooking process becomes more accurate when the AI also processes the recipe text, linking the ingredients and steps with the visual cues. This results in a richer and more nuanced representation of the information.
- Improved Learning Efficiency: By disentangling these elements, the AI can learn more efficiently from both sources. It avoids the confusion that may arise from treating the data as a monolithic whole, allowing for better alignment and interpretation of visual and textual information.
- Real-World Applicability: This approach enables the AI to better handle real-world scenarios where data is inherently multimodal. For instance, in autonomous driving, disentangled learning helps in correlating visual inputs (like road signs) with textual instructions (like speed limits), thus improving decision-making.
The novelty of this approach lies in how the system learns cooperatively. Rather than treating video and text as independent sources of information, the model uses both in tandem, allowing the text to provide context for the visuals and vice versa. This cooperative learning leads to richer representations, where the AI understands more than just the surface-level features of the video or the literal meaning of the text.
Training AI to Learn Like Humans
This cooperative learning approach mirrors the way humans process information. When we watch a video, we don’t just see the images on the screen—we also use language to explain what’s happening, drawing connections between our senses. For instance, in a documentary, we understand the visuals of animals in their habitat through the narrator’s explanation, which adds layers of meaning to what we see.
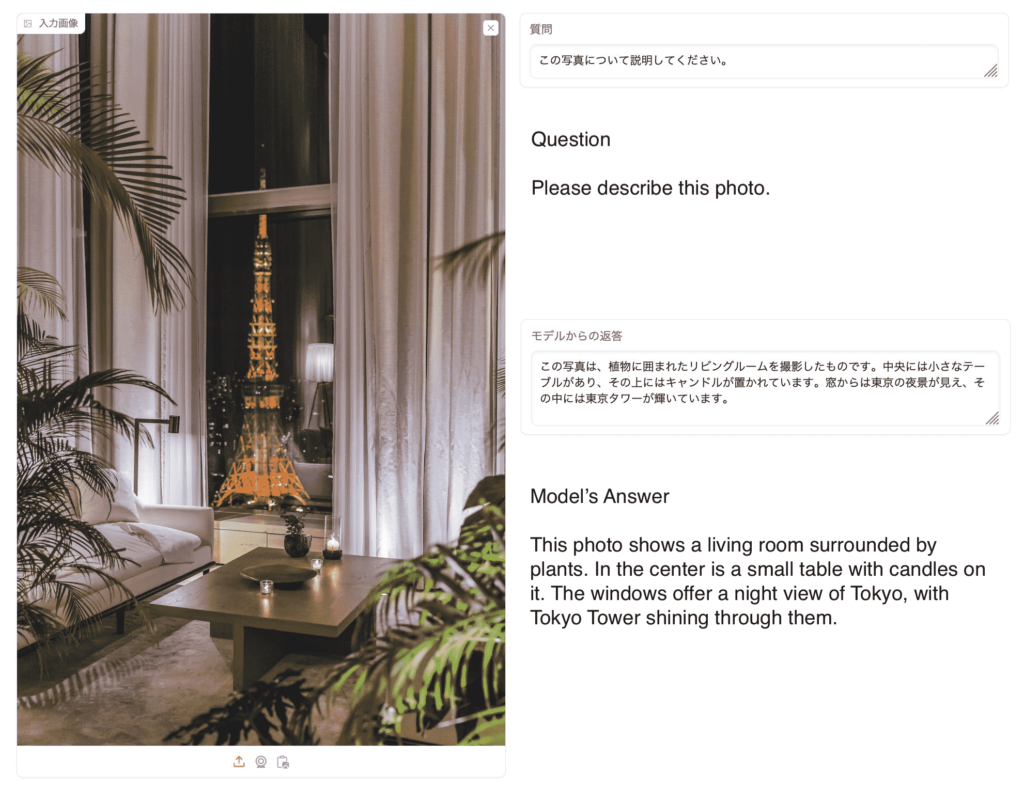
In the same way, this method allows AI to combine video and textual data, learning richer, disentangled representations of the real world. The model is trained to align video clips with textual descriptions, helping it to better understand how specific scenes in a video correspond to the descriptions in text. This multimodal learning opens up new possibilities for AI systems to handle tasks that require deep understanding across different types of data.
Potential Applications of Cooperative Learning
The implications of this research are vast. One potential application is in autonomous systems, such as self-driving cars, which must constantly analyse visual and verbal information to make decisions. By disentangling the visual and textual components, an AI-powered car could better understand road signs, traffic signals, or verbal instructions from passengers.
Another area where this could have a significant impact is content recommendation systems. With a deeper understanding of both videos and textual content, systems like YouTube or Netflix could offer more personalised recommendations, matching videos to users based on a nuanced understanding of both the video content and the textual descriptions or subtitles.
Challenges and Future Directions
While this cooperative learning model shows great promise, it also comes with challenges. For one, aligning text with videos in a meaningful way requires high-quality data and well-labelled examples. Moreover, disentangling representations in a way that consistently improves performance remains a difficult task, especially in diverse real-world scenarios.
The researchers also acknowledge that more work is needed to explore how this model performs across different types of videos and texts, as well as how it might be extended to other modalities, like audio or sensor data.
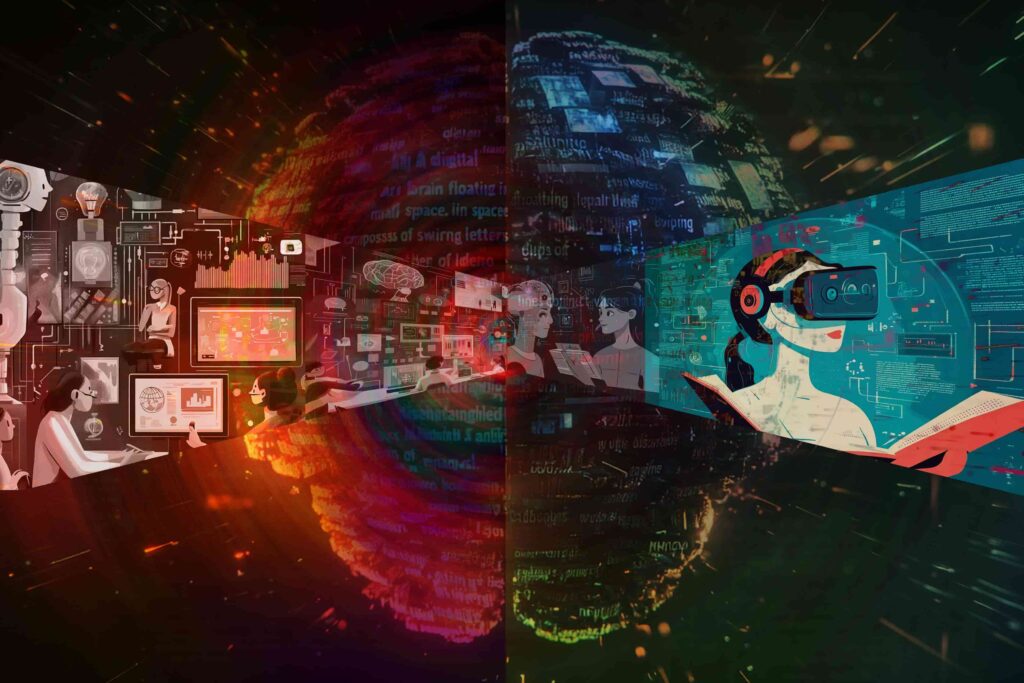
Conclusion
The paper “Cooperative Learning of Disentangled Representations from Video and Text” offers a new perspective on how artificial intelligence can learn more effectively from multiple data sources. By allowing AI to learn cooperatively from both video and text, the researchers are helping push the boundaries of machine perception. This approach holds the potential to revolutionize fields from autonomous systems to content recommendation, paving the way for AI that can understand the world with a level of depth and context that’s more human than ever before.
Reference
Sakana.AI. “Evolving New Foundation Models: Unleashing the Power of Automating Model Development,” March 21, 2024. https://sakana.ai/evolutionary-model-merge/.
Wang, Qiang, Yanhao Zhang, Yun Zheng, Pan Pan, and Xian-Sheng Hua. “Disentangled Representation Learning for Text-Video Retrieval.” arXiv.org, March 14, 2022. https://arxiv.org/abs/2203.07111.
Let us know your thoughts! Sign up for a Mindplex account now, join our Telegram, or follow us on Twitter.
3 Comments
3 thoughts on “Cooperative Learning: How Videos and Text Are Helping AI Understand the World”
Teaching AI to learn from both video and text is a big step forward, helping machines understand the context better, like how we connect what we see with what we hear or read in real life.
🟨 😴 😡 ❌ 🤮 💩
"AI is leveling up with a crash course in reality, thanks to videos and text teaming up like the ultimate study buddies! This approach is like teaching a toddler how to navigate the world—except the toddler is a machine learning genius. Love how this takes AI from 'book smart' to 'street smart'! What do you think will be the coolest thing AI learns to 'understand' next? 🌎🤖"
🟨 😴 😡 ❌ 🤮 💩
This innovative approach by Sakana AI is a game-changer, bridging the gap between video and text learning for AI. It paves the way for more human-like understanding and opens doors to exciting real-world applications
🟨 😴 😡 ❌ 🤮 💩