DeepMind's transformer model challenges Stockfish 16 in chess, achieving 2895 Lichess blitz Elo rating without search algorithms.
Artificial Intelligence (AI) has been a significant player in the world of chess for decades, with systems like IBM’s Deep Blue making headlines in the late 90s for defeating world champion Garry Kasparov. More recently, AI advancements have led to the development of systems like AlphaZero and Stockfish 16, which use machine learning techniques to improve their gameplay.
Research in the area still continues robustly, as exemplified by a recent paper from Google DeepMind. The DeepMind researchers have trained a transformer model with 270 million parameters using supervised learning on a dataset of 10 million chess games. Each game in the dataset was annotated with action-values provided by the powerful Stockfish 16 engine, which led to approximately 15 billion data points.
In the world of chess, a player’s skill level is often measured using the Elo rating system. An average club player might have an Elo rating of around 1500, while a world champion’s rating is typically over 2800. A Lichess blitz Elo rating of 2895, as mentioned in this paper, indicates a very high level of skill, comparable to the top human players in the world.
The model was able to achieve a Lichess blitz Elo rating of 2895 when playing against human opponents, and it was also successful in solving a series of challenging chess puzzles. Remarkably, these achievements were made without any domain-specific tweaks or explicit search algorithms.
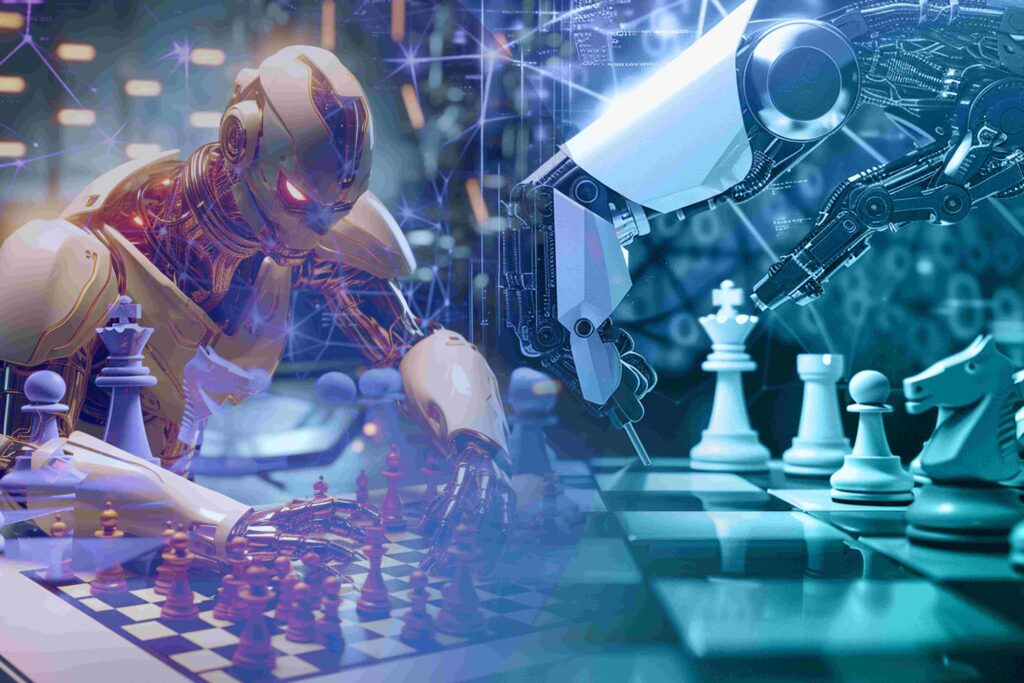
In terms of performance, the model outperformed AlphaZero’s policy and value networks (without MCTS) and GPT-3.5-turbo-instruct. The researchers found that strong chess performance only arises at sufficient scale. They also conducted an extensive series of ablations of design choices and hyperparameters to validate their results.
The researchers concluded that it is possible to distill a good approximation of Stockfish 16 into a feed-forward neural network via standard supervised learning at sufficient scale. This work contributes to the growing body of literature showing that complex and sophisticated algorithms can be distilled into feed-forward transformers. This implies a paradigm shift away from viewing large transformers as mere statistical pattern recognizers to viewing them as a powerful technique for general algorithm approximation.
The paper also discusses the limitations of the model. While the largest model achieves very good performance, it does not completely close the gap to Stockfish 16. All scaling experiments point towards closing this gap eventually with a large enough model trained on enough data. However, the current results do not allow the researchers to claim that the gap can certainly be closed.
Another limitation discussed is that the predictors see the current state but not the complete game history. This leads to some fundamental technical limitations that cannot be overcome without small domain-specific heuristics or augmenting the training data and observable information.
Finally, when using a state-value predictor to construct a policy, the researchers consider all possible subsequent states that are reachable via legal actions. This requires having a transition model ? (?, ?), and may be considered a version of 1-step search. While the main point is that the predictors do not explicitly search over action sequences, the researchers limit the claim of ‘without search’ to their action-value policy and behavioral cloning policy.
In conclusion, the paper presents a significant advancement in the field of AI and chess, demonstrating that a complex, search-based algorithm, such as Stockfish 16, can be well approximated with a feed-forward neural network via standard supervised learning. This has implications for the broader field of AI, suggesting that complex and sophisticated algorithms can be distilled into feed-forward transformers, leading to a paradigm shift in how we view and utilize large transformers.
Let us know your thoughts! Sign up for a Mindplex account now, join our Telegram, or follow us on Twitter.
8 Comments
8 thoughts on “Grandmaster-Level Chess Without Search”
Nice Article
🟨 😴 😡 ❌ 🤮 💩
Great!
🟨 😴 😡 ❌ 🤮 💩
🟨 😴 😡 ❌ 🤮 💩
🟨 😴 😡 ❌ 🤮 💩
All really interesting stuff!
🟨 😴 😡 ❌ 🤮 💩
Good!
🟨 😴 😡 ❌ 🤮 💩
Over time humans will be replaced by AI. This makes me a little worried because little by little all fields will be taken over by AI
🟨 😴 😡 ❌ 🤮 💩
nice write
🟨 😴 😡 ❌ 🤮 💩