Bias Whispers: The Untold Story of Learning Without Synaptic Change
Feb. 06, 2025. 4 mins. read.
38 Interactions
Forget synaptic weight changes—what if learning happens differently? A new study shows neural networks can adapt by adjusting only biases, reshaping our understanding of AI and the brain.
For decades, neuroscience and artificial intelligence have focused on synaptic plasticity—the strengthening or weakening of connections between neurons—as the fundamental mechanism of learning. However, a groundbreaking study led by Ezekiel Williams and colleagues challenges this view, demonstrating that neural networks can learn and adapt by modifying only their biases while keeping synaptic weights fixed. This revelation not only reshapes our understanding of artificial intelligence but also provides new insights into the adaptability of biological neural systems.
Unlocking Hidden Potential: Bias Learning in Neural Networks
Traditionally, training a neural network involves adjusting both synaptic weights and biases. Synaptic weights determine how strongly neurons influence each other, while biases set the baseline activity of individual neurons. In a radical departure from conventional training methods, the researchers froze synaptic weights at random values and allowed only biases to change. Surprisingly, these “bias-learning” networks were still capable of performing complex tasks, including image classification and forecasting the behavior of dynamical systems.
The researchers found that, despite fixed connectivity, bias learning alone enabled networks to form functional representations necessary for task execution. This suggests that neural networks can adapt by merely fine-tuning neuronal activity rather than restructuring their connections—offering a potential paradigm shift in understanding neural computation.
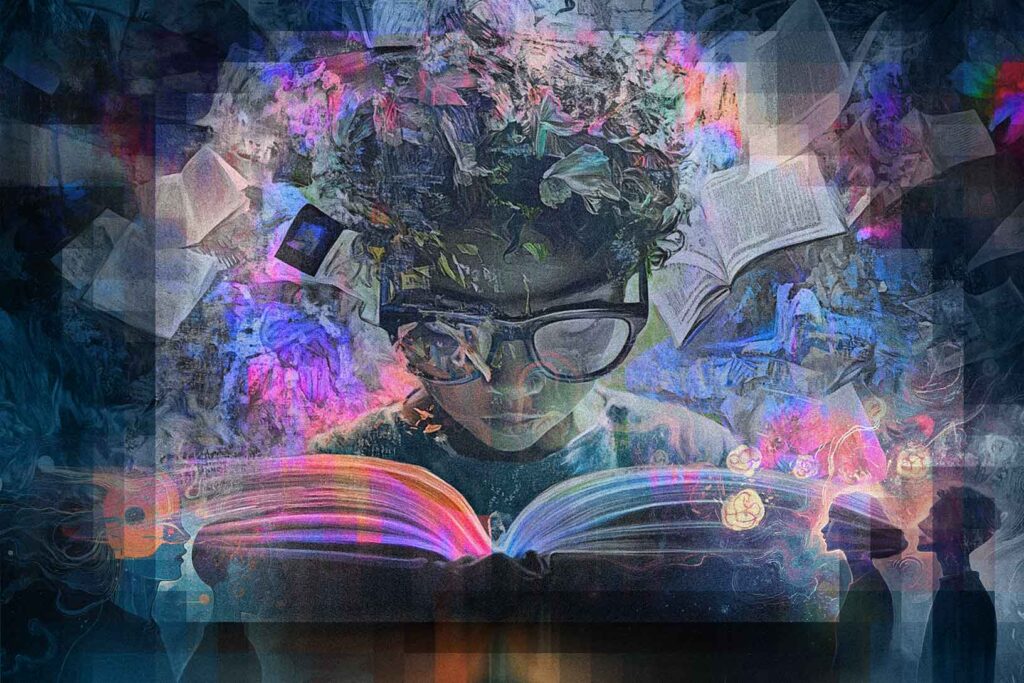
Multitasking Marvels: One Network, Many Talents
A particularly striking aspect of bias learning is its ability to support multitasking. The study demonstrated that a single neural network with fixed random weights could perform multiple classification tasks by simply adjusting biases for each task. This is akin to an orchestra playing different musical pieces by changing the volume levels of instruments rather than altering their arrangement.
Analysis of these networks revealed functional specialization, with clusters of neurons emerging for specific tasks. This suggests that bias learning not only enables multitasking but also facilitates the self-organization of neural circuits, even without changes in connectivity. Such findings have profound implications for artificial intelligence, where bias fine-tuning could be leveraged for efficient multi-task learning and transfer learning in deep learning models.
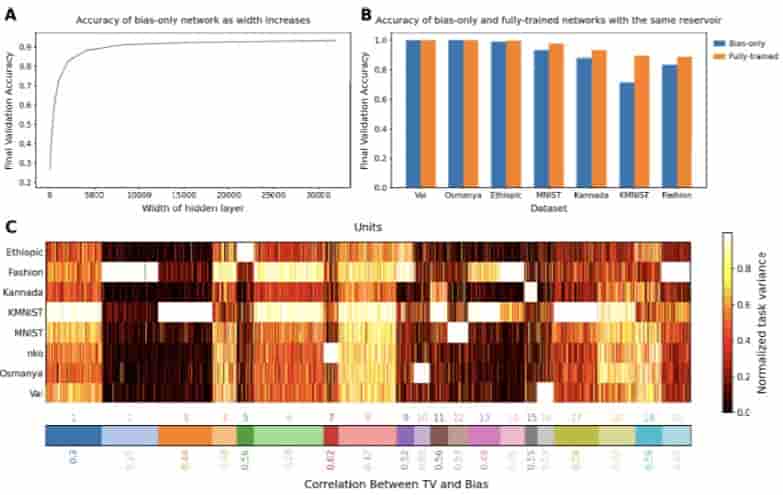
Echoes in the Brain: Bias and Non-Synaptic Plasticity
The findings align with emerging discoveries in neuroscience that highlight the role of non-synaptic plasticity in learning. The brain employs mechanisms such as shunting inhibition, neural gain modulation, and threshold adaptation to regulate activity independently of synaptic weight changes. These processes bear a striking resemblance to bias learning in artificial networks, suggesting that biological systems might exploit similar principles for cognitive flexibility and adaptation.
Moreover, the study explored bias learning in recurrent neural networks (RNNs), which are often used to model time-dependent processes in neuroscience. By adjusting only biases, these networks successfully generated and predicted the trajectories of dynamical systems, mimicking the brain’s ability to produce complex motor and cognitive patterns. This supports the notion that the brain could dynamically regulate neural function through bias adjustments rather than extensive synaptic remodeling.
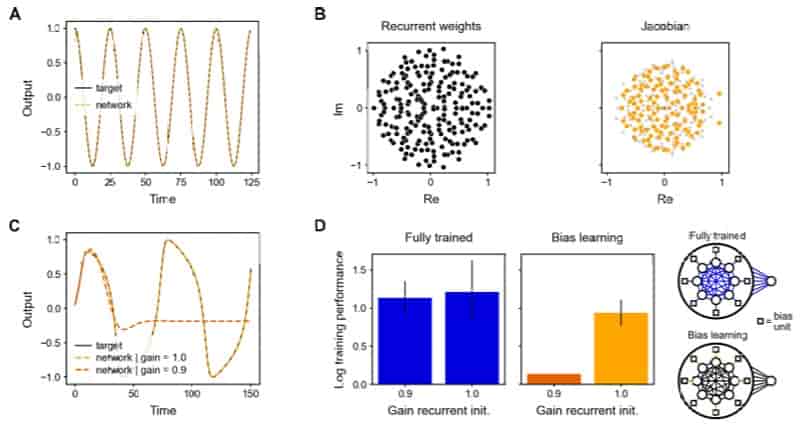
Theoretical and Practical Implications
The theoretical contributions of this study extend beyond artificial intelligence and neuroscience. The researchers provided mathematical proofs demonstrating that neural networks with fixed weights but learned biases can serve as universal function approximators with high probability. This suggests that the flexibility of neural computation may stem not only from synaptic modifications but also from changes in intrinsic neuronal properties.
Practically, bias learning presents a promising avenue for more efficient AI models. Traditional deep learning requires extensive retraining of weights, consuming significant computational resources. Bias learning could enable rapid adaptation to new tasks with minimal computational overhead, offering new strategies for developing adaptive and energy-efficient AI systems.
Conclusion
The discovery of bias learning challenges the long-held belief that synaptic weight changes are the sole driver of learning. By demonstrating that neural networks can learn and specialize through bias modulation alone, this study bridges artificial intelligence and neuroscience, shedding light on alternative pathways for cognitive adaptation. As research progresses, bias learning may not only revolutionize AI but also deepen our understanding of how the brain fine-tunes its functions to navigate an ever-changing world.
References
Williams, Ezekiel, Avery Hee-Woon Ryoo, Thomas Jiralerspong, Alexandre Payeur, Matthew G. Perich, Luca Mazzucato, and Guillaume Lajoie. “Expressivity of Neural Networks With Random Weights and Learned Biases.” arXiv.org, July 1, 2024. https://arxiv.org/abs/2407.00957.
Let us know your thoughts! Sign up for a Mindplex account now, join our Telegram, or follow us on Twitter.
6 Comments
6 thoughts on “Bias Whispers: The Untold Story of Learning Without Synaptic Change”
This is mind-blowing really! The idea that neural networks can learn just by adjusting biases, without changing weights, completely shifts how we think about adaptation in AI and the brain.
🟨 😴 😡 ❌ 🤮 💩
This article is a fascinating look at how bias learning in neural networks challenges traditional AI methods. It shows that networks can adapt and perform complex tasks through bias adjustments alone without changing synaptic weights, opening the door for more efficient AI systems. The parallels to non-synaptic plasticity in the brain are especially intriguing, suggesting nature might use similar strategies.
🟨 😴 😡 ❌ 🤮 💩
good read
🟨 😴 😡 ❌ 🤮 💩
This is amazing!!!
It suggests that we are devising many ways that may lead us to AGI. Viva Ezekiel Williams
🟨 😴 😡 ❌ 🤮 💩
This is a fascinating breakthrough!
The idea that neural networks can adapt by adjusting biases rather than synaptic weights opens up exciting possibilities for more efficient AI systems. It’s inspiring to see such innovative research that could revolutionize the way we approach learning in both artificial and biological systems. Looking forward to seeing where this leads!
🟨 😴 😡 ❌ 🤮 💩
This is a fascinating exploration of bias learning in neural networks! The idea that learning can occur through bias adjustments alone, without the need for synaptic weight changes, is a significant shift in our understanding of both artificial intelligence and biological systems. It opens up exciting possibilities for developing more efficient AI models that can adapt quickly to new tasks with minimal computational resources.
Moreover, the parallels drawn between bias learning and non-synaptic plasticity in the brain highlight the potential for a deeper understanding of cognitive flexibility. This research not only challenges long-held beliefs about learning mechanisms but also paves the way for innovative approaches in AI development. I'm eager to see how these findings will influence future research and applications in both fields!
🟨 😴 😡 ❌ 🤮 💩