How Generative AI Learns Concepts: Unveiling the Concept Space Framework
Apr. 30, 2025. 6 mins. read.
1 Interactions
How do AI models really learn? A new framework maps their internal learning paths—revealing why some concepts emerge early and others spark sudden leaps in intelligence.
Introduction
From producing surreal paintings to decoding protein structures, generative AI models have demonstrated startling prowess. Yet a fundamental mystery remains: how do these models learn the abstract concepts they so seamlessly manipulate? What governs the order in which a model learns to recognize a circle, distinguish a dog from a cat, or grasp complex grammar structures? A new research framework, introduced by Park et al., titled ‘Emergence of Hidden Capabilities: Exploring Learning Dynamics in Concept Space‘, proposes an answer. The authors present a method to track and understand the learning process of generative models by embedding concepts into a geometric space—a “concept space”—where learning unfolds not as an invisible computation, but as a measurable trajectory.
This approach reframes AI learning as a structured, trackable progression through abstract representations. Through this lens, Park and colleagues not only reveal the hidden order by which generative models internalize concepts, but also shed light on the sudden, emergent abilities often attributed to these models.
From Performance to Process: What Is the Concept Space Framework?
Mapping Learning as a Trajectory
Traditionally, machine learning has emphasized outputs: does a model classify correctly, generate realistic images, or follow instructions? But such results offer little insight into how learning unfolds internally. The concept space framework introduces a geometric abstraction. Here, each concept—such as a shape, a digit, or a semantic category—is treated as a basis vector within a high-dimensional space. The model’s state of knowledge is represented as a point (or trajectory) in this space. As training proceeds, the model traces a path through this space, revealing the order and rate at which it learns each concept.
Crucially, this approach allows researchers to monitor not just what a model knows, but when it learns each element of that knowledge. For instance, in a dataset with digits from 0 to 9, the model might first master 1s and 0s before progressing to less frequent or visually complex digits like 8 or 5.
The Concept Signal: A Quantitative Driver
What determines the order in which a model learns? Park et al. introduce the notion of a “concept signal,” a measurable quantity that reflects how strongly a dataset promotes the learning of a given concept. A concept with a high signal appears frequently, is visually distinct, or is supported by many variations. High-signal concepts are learned earlier and more robustly.
This concept signal is extracted from the gradients that update the model’s weights during training. In essence, it quantifies how much a given data point pulls the model toward understanding a particular concept. By aggregating this information, researchers can predict which concepts will emerge early in training—and which will lag.
Sudden Jumps: The Geometry of Epiphanies
One of the most striking findings is the observation of “trajectory turns”— abrupt changes in the model’s direction through concept space. These turns signal moments of conceptual discovery, when the model rapidly gains proficiency in a previously elusive concept. Such behavior echoes human “aha” moments, and provides a mechanistic explanation for emergent capabilities often observed in large models: these aren’t magical leaps, but structured transitions triggered by sufficient conceptual grounding.
Experimental Insights: Validating the Concept Space
Synthetic Datasets: Testing the Theory
To validate their framework, the researchers first constructed controlled synthetic datasets, where each data point was labeled by a specific concept (e.g., geometric shapes like triangles or circles). By varying the frequency and clarity of these concepts in the data, they manipulated the concept signal directly.
The results confirmed their hypotheses: high-signal concepts were learned earlier, and modifying the signal predictably changed the order in which concepts emerged. These experiments provided a clean, interpretable setting to demonstrate the explanatory power of the framework.
Real-World Experiments: MNIST and Beyond
Moving to real-world datasets, the authors applied the framework to models trained on MNIST digits and CelebA facial attributes. In both cases, the concept signal accurately predicted learning order. For example, in MNIST, frequent and simple digits like “1” and “0” were mastered early, while more complex or ambiguous digits like “5” or “8” emerged later.
Furthermore, the framework captured the sudden transitions in concept understanding: trajectory turns were strongly associated with rapid accuracy improvements and changes in output quality.
Practical Implications and Future Potential
Optimizing Training Curricula
One of the most actionable insights from the concept space framework is its potential for curriculum design. By identifying high-signal concepts early, training data can be structured to accelerate learning, improve generalization, and reduce resource consumption. For instance, feeding the model easier or more foundational concepts first could mirror pedagogical strategies in human education.
Explaining Emergent Behaviors
Modern AI models often exhibit surprising capabilities not explicitly trained for—from doing arithmetic to reasoning abstractly. The concept space framework suggests a mechanism: these abilities may emerge when the model crosses a threshold in concept space, triggering a cascade of related conceptual gains. Such analysis could help predict and control these behaviors, improving safety and interpretability.
Toward Interpretability and Debugging
By providing a map of what the model knows at any given point in training, the concept space framework offers a tool for interpreting and debugging AI systems. If a model behaves oddly, tracing its trajectory can reveal which concepts are underdeveloped or overfit, aiding targeted interventions.
Generalization Across Modalities
While the study focuses on vision tasks, the framework is designed to be modality-agnostic. Future applications may extend to natural language processing, multi-modal models, and reinforcement learning, wherever a model must internalize abstract representations.
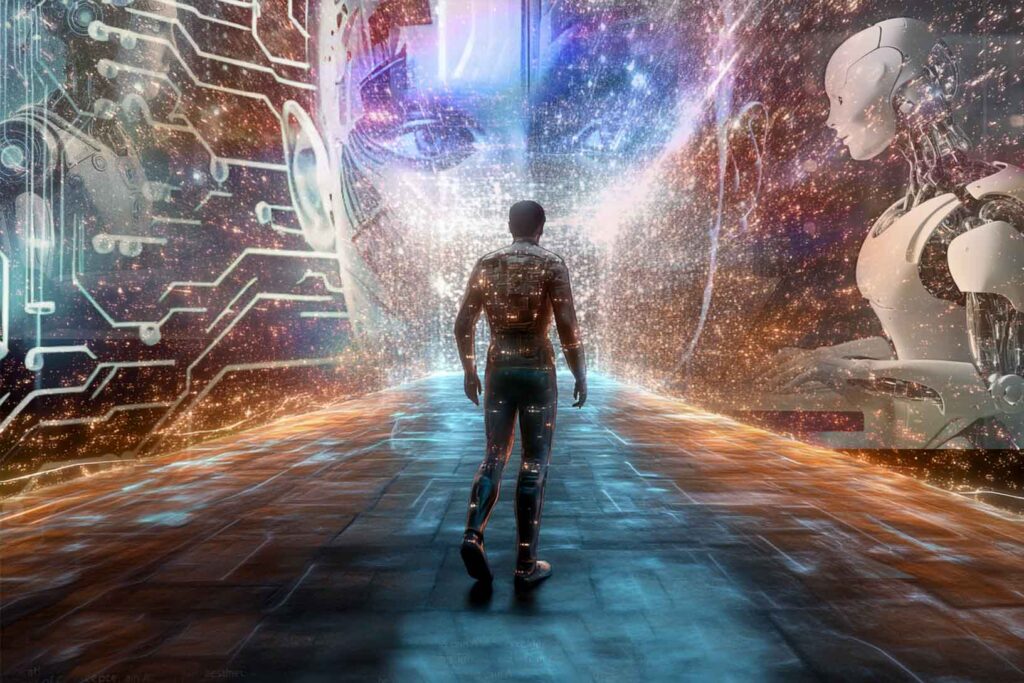
Open Questions and Challenges
The authors acknowledge limitations. The framework currently requires well-defined and labelable concepts; tasks with ambiguous or overlapping categories pose challenges. Additionally, as model size and data complexity grow, computational costs rise.
Extending the approach to unsupervised or self-supervised settings, where concepts must be inferred rather than predefined, remains an open frontier. Moreover, developing automated methods for discovering latent concepts within large datasets could supercharge the framework’s utility.
Broader Impact: From AI Education to Societal Applications
By shedding light on the inner workings of AI learning, the concept space framework has broad implications. In education, it could inform the design of AI-driven tutors that adapt teaching strategies based on concept acquisition. In industry, it offers tools for monitoring model development, enhancing transparency in finance, healthcare, and legal systems. Most of all, it pushes the field closer to interpretable AI, where the how and why of model behavior are as clear as the results.
Conclusion
The concept space framework marks a major milestone in our understanding of artificial intelligence. By framing learning as a trajectory through concept space, Park et al. reveal the hidden geometry behind model development. Their findings on concept signal, emergent turns, and predictable learning order offer both theoretical insights and practical tools. As this approach evolves, it holds promise not just for improving AI, but for reshaping how we think about intelligence—human and machine alike.
Reference
Park, Core Francisco, Maya Okawa, Andrew Lee, Hidenori Tanaka, and Ekdeep Singh Lubana. “Emergence of Hidden Capabilities: Exploring Learning Dynamics in Concept Space.” arXiv.org, June 27, 2024.. https://arxiv.org/abs/2406.19370
Let us know your thoughts! Sign up for a Mindplex account now, join our Telegram, or follow us on Twitter.
0 Comments
0 thoughts on “How Generative AI Learns Concepts: Unveiling the Concept Space Framework”