AI for faster quantum mechanical simulations in material science
Nov. 06, 2024.
2 mins. read.
6 Interactions
Neural networks make the calculations needed for understanding how materials behave at the atomic level significantly faster.
Researchers at KAIST have found ways to speed up quantum mechanical simulations by integrating an artificial intelligence (AI) model called DeepSCF. This model was developed to make the calculations needed for understanding how materials behave at the atomic level significantly faster.
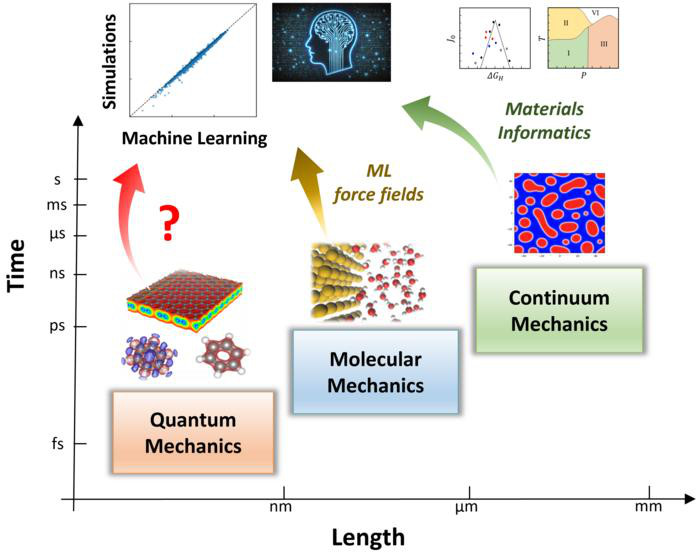
One of the key methods used in quantum mechanical simulations is Density Functional Theory (DFT), which calculates the properties of materials from first principles, meaning it starts from the basic laws of quantum physics without any empirical parameters. However, DFT calculations are computationally intensive because they involve solving complex equations to determine electron density in three-dimensional space, a process known as the self-consistent field (SCF) method. This method iteratively calculates electron density until the results stabilize, which can take many iterations, making it time-consuming especially for larger systems.
The KAIST researchers introduced the DeepSCF model to overcome these limitations. DeepSCF uses a neural network similar to those used for 3D computer vision to learn the 3D patterns of electron density and chemical bonding.
The researchers describe the DeepSCF project and early results in a paper published in npj Computational Materials.
Instead of repeatedly solving equations through SCF, DeepSCF recognizes chemical bonding patterns, learning from a dataset of known molecular structures how electron density should look for new or altered materials.
AI predictions replace intensive computations
This innovation replaces the traditional, computationally heavy steps with AI predictions. The researchers trained the neural network with data from various organic molecules, enhancing the model’s ability to generalize across different chemical scenarios by applying random rotations and deformations to these structures. This allows the model to not only predict electron densities but also other quantum mechanical properties, with an accuracy comparable to conventional DFT calculations but much faster.
According to the researchers, DeepSCF represents a leap forward in materials science and quantum chemistry, offering a tool that can quickly predict material properties without the need for extensive computational resources. DeepSCF opens up new possibilities for simulating larger and more complex material systems efficiently.
“Since quantum mechanical electron structure calculations underpin materials simulations across all scales,” says research leader Yong-Hoon Kim in a KAIST press release, “this research establishes a foundational principle for accelerating material calculations using artificial intelligence.”
Let us know your thoughts! Sign up for a Mindplex account now, join our Telegram, or follow us on Twitter.
0 Comments
0 thoughts on “AI for faster quantum mechanical simulations in material science”