Neural Networks: Hiding Invisible Doors with Unbreakable Locks
Mar. 03, 2023.
1 min. read.
2 Interactions
Using digital signatures and encryption, researchers create undetectable backdoors in machine learning models.
Computer scientists have demonstrated that undetectable backdoors can be implanted in machine learning models with the same certainty as cutting-edge encryption methods. Researchers have developed various methods to conceal their own sample backdoors in machine learning models in order to better understand machine learning model vulnerabilities. The researchers investigated two scenarios for outsourcing neural network training. In the first scenario, a company provides training data to a third party without specifying what type of neural network to build or how to train it. In the second scenario, a company specifies the network architecture and training procedure to be used but lacks the computational resources to train it. The team’s research discovered that undetectable backdoors could still be planted in the “white-box” scenario.
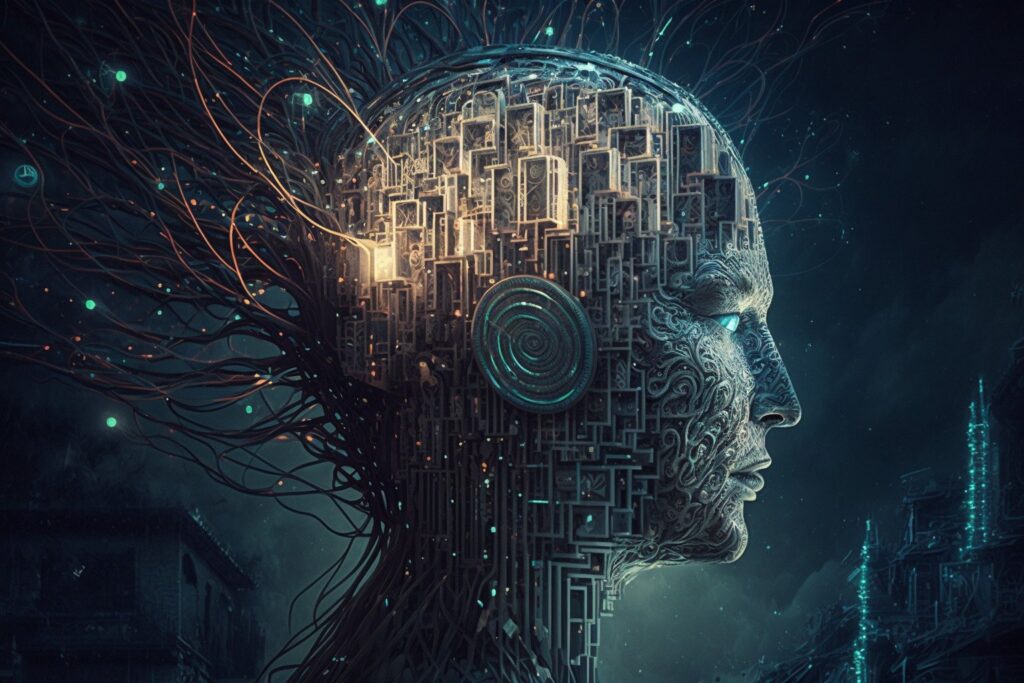
The researchers’ method is based on the mathematics that underpins digital signatures. They included a “verifier” module, which controls a backdoor by modifying the model’s output if it detects a special signature. An attacker knows the corresponding secret key, which is a function that generates a unique signature for any possible input and then slightly modifies the input to encode that signature. Companies that do not request a specific type of neural network and only test the trained model on new data may be vulnerable to black-box undetectable backdoors. White-box undetectable backdoors would be invisible even to a defender who could inspect all network details at the end of the training process. While the new work has some trade-offs, such as a focus on relatively simple models, it establishes a new theoretical link between cryptographic security and machine learning vulnerabilities, suggesting new directions for future research in the two fields.
Source: Quanta Magazine (link)
Images: MidJourney, Prompts by Lewis Farrell
Interesting story? Please click on the ? button below!
Let us know your thoughts! Sign up for a Mindplex account now, join our Telegram, or follow us on Twitter.Â
0 Comments
0 thoughts on “Neural Networks: Hiding Invisible Doors with Unbreakable Locks”