Let us know your thoughts! Sign up for a Mindplex account now, join our Telegram, or follow us on Twitter.
DEGENS: DOWN & OUT IN THE CRYPTO CASINO
Degen – short for degenerate – is a term used to describe crypto investors who like to think of themselves as impulsive enthusiastic risk-takers and market outsiders. For the film-in-progress Degens: Down & Out In the Crypto Casino, directors Brian McGleenon and Joe Haughey focus on the prankster-esque desperation of many in this world. As they say on their website, “The film explores the inner world of one of the most fascinating and overlooked counter-cultural movements of our time. A world inhabited by Degens, short for degenerates, who invest their meager income into memecoins.” These are the people who have minted coins with names like Shitcoin, Cumrocket, Amber Turd and HarryPotterObamaSonic10Inu.
To some degree, they have also forced mainstream media to repeat these “vulgarities” that, of course, only reflect and reify the vulgarity particularly here in the USA where leading politicians or by comedian talk show host Bill Maher on CNN say ‘fuck’ regularly. It’s all indicative of a collapsing or fragmenting narrative in the context of the decline or recline of western civilization.
To elucidate this shadow world of high (on acid) finance, McGleenon and Haughey have incorporated a number of smart commentators. These range from Gordon Grant (he will be my follow-up interview), a cryptocurrency derivatives trader and the Head of Trading at Genesis, to leading US Marxist academic Richard D. Wolff and to (not least of all) PizzaT.
Full disclosure: I work with PizzaT on music under the banner of R.U. Sirius and Phriendz, which spins off of his own Phriendz music and other projects. We’ve even composed a theme song together for the Degens project titled ‘Degens & Phriendz’ that will be available soon. Aside from being a Sirius fellow and an astonishingly talented musician, composer and guitarist, Pizza, as described by the filmmakers… “is the center of all this madness. The memecoin groups orbit him like satellites spinning out of control. ”So set your stun guns to out-of-control, and dive into this interview about Degens: Down and Out in the Crypto Casino.
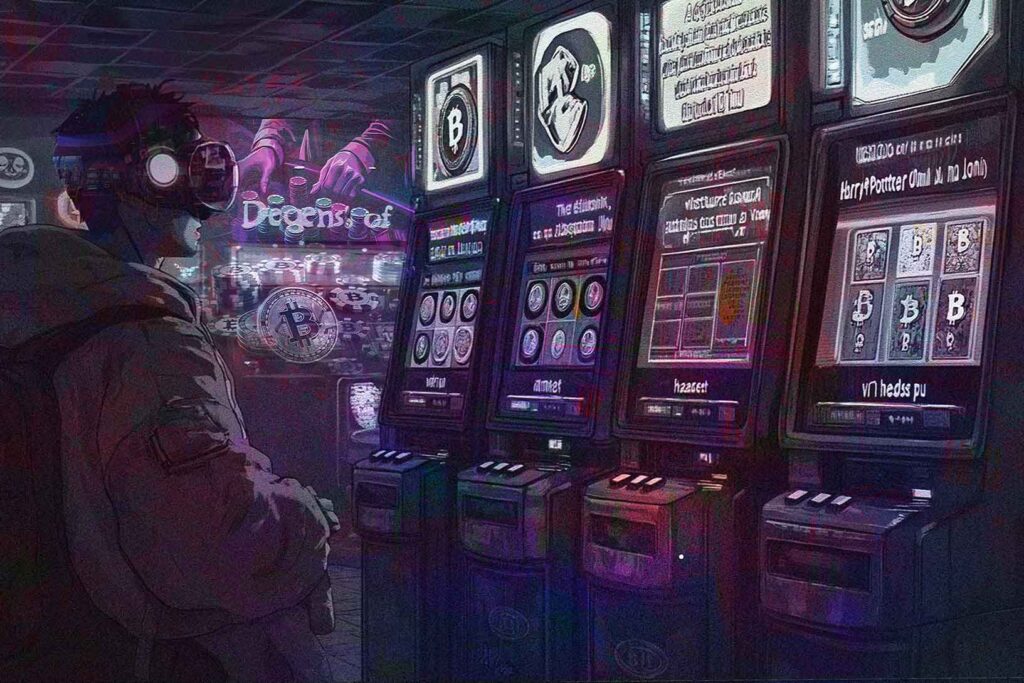
Let us know your thoughts! Sign up for a Mindplex account now, join our Telegram, or follow us on Twitter.
DeepSeek: Finding Crypto AI Magic
Introduction
The year 2025 started with another a AI bombshell. Mayhem struck financial markets as the US hegemony over the next generation of artificial intelligence was cast in serious doubt by a new challenger who only had $10 million funding, breaking confidence in the market valuation of OpenAI, nVidia, Google and Microsoft..
DeepSeek, a Chinese AI start-up founded in 2023, shocked the tech world when it released its open-source model, DeepSeek R1, in January. The model offers ChatGPT-like performance at a very low cost. It quickly topped the charts in global mobile app downloads, with India providing the highest percentage.
Is DeepSeek’s R1 model a real threat to Silicon Valley? Is it as cheap to train as reported? Will it further affect the financial and crypto markets? Can it democratize cheaper AI adoption and connect with Web3 protocols? Questions questions questions. Let’s dig in.
DeepSeek’s R1 Launch Causes Tremors
DeepSeek’s R1 launch shook the U.S. tech industry, particularly the AI sector. It was released days after U.S. President Donald Trump announced the creation of the Stargate Project, a partnership between OpenAI, SoftBank, and Oracle to build the largest AI infrastructure in the United States of America. The private partnership plans to invest $500 billion in the project.
Yet, DeepSeek caused tremors when it said it spent less than $6 million to train its models. This is 50 times cheaper to run than its U.S. competitors.
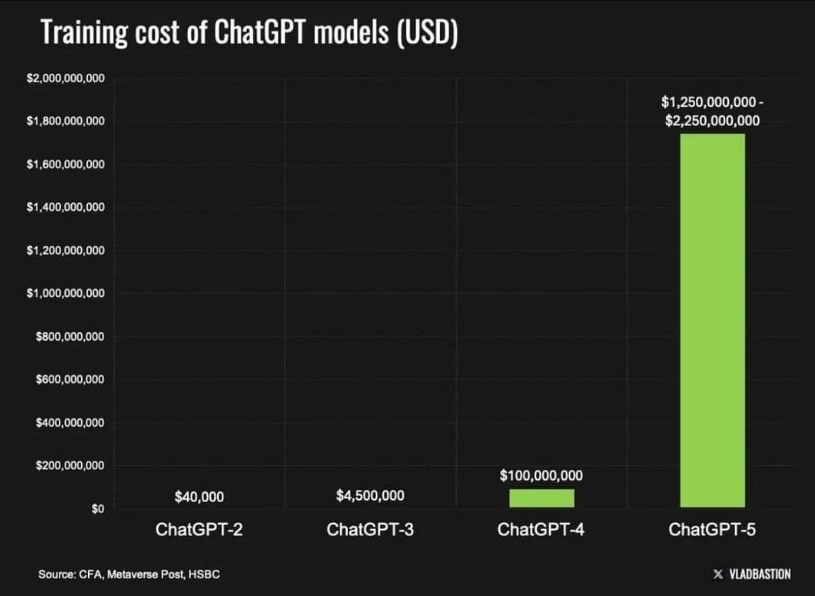
Scientists have been thrilled by its performance. They say that its performance in chemistry, mathematics, and coding is comparable to that of OpenAI o1.
The cost-efficiency of DeepSeek’s R1 model rattled investors and financial markets suffered major losses. Nvidia Corporation, a leader in the AI sector, tanked 17% and wiped off $579 billion from its market cap: the largest single-day fall in market cap of any company in the history of the stock market. The Nasdaq, a tech-heavy stock market, lost over $1 trillion as other AI competitors recorded losses.
But was this fear justified? Or is the launch of DeepSeek’s R1 model something the AI market should have anticipated? After all, the global AI race is heating up, with new artificial intelligence advances to be expected from the competitors.
The USA has expressed privacy concerns associated with DeepSeek and a senator has introduced a bill that could criminalize downloading the app.
DeepSeek R1’s Impact on AI Markets
DeepSeek’s R1 has disrupted the AI markets and could force other startups to rethink their strategies, but the stock market sell-off was a once-off event.
Investors are questioning if they need to invest large sums of investments into AI when DeepSeek signals a shift toward more open and cheaper models.
If new AI models require fewer GPUs for training and inference, major chipmakers like Nvidia could face slower demand growth. However, despite higher efficiencies, the demand for computing power won’t decrease. The winners in the new shift are end users and AI application providers. They benefit from increased model availability and lower API costs. On the other hand, proprietary model providers (like OpenAI and Anthropic) face increasing pressure as free and customizable alternatives emerge.
These are still early days, but companies like OpenAI may need to rethink their business strategies to stay competitive.
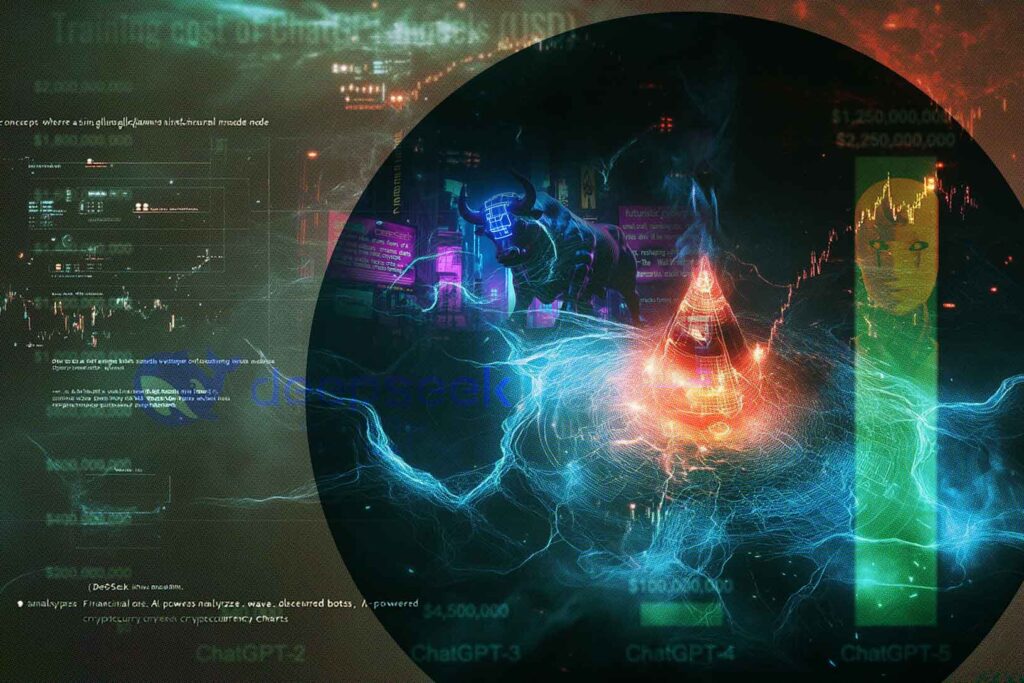
Implications for Crypto: Risks and Opportunities
The financial bloodbath caused by DeepSeek’s R1 model extended beyond the stock market to the crypto industry, turning its markets upside down.
Apart from the fluctuations normal to the crypto industry, there are special opportunities and risks presented by DeepSeek.
Let’s start with the opportunities.
Affordable AI Agents
DeepSeek can help accelerate and democratize AI development, something that could benefit everyone. For the crypto industry, an early benefit will come in the form of cheaper AI agents. These agents can analyze top altcoin charts and handle tasks such as trading, portfolio management, and risk management.
Accelerated AI Adoption
DeepSeek’s cost-efficiency and open-source model could accelerate the integration of AI into crypto sectors such as DeFi, blockchain security, and on-chain intelligence.
Democratization of AI
Many startups have been using proprietary AI models, making it impossible for smaller firms to compete in this market. However, the open-source nature of DeepSeek could lower the barrier to entry and open the crypto market to cheaper and innovative players.
Lower Inflation
The low-cost nature of DeepSeek AI may help reduce inflation, favoring non-AI-linked assets such as Bitcoin over ones affected by the recent downturn in the AI market.
A Flurry of Scam DeepSeek Tokens
Anyone who has been in the crypto industry more than a minute knows that scammers appear after any hype wave as surely as flies follow cows.
The popularity of DeepSeek’s R1 brought out grifters, with many of them launching scam tokens claiming to be associated with DeepSeek. These tokens were seen on Ethereum, Solana, and other layer-1 chains. A Solana-based DeepSeek fake token reached a market cap of $48 million before cooling off while the other peaked at $13 million. There are even many more.
In these instances, traders should check for official announcements from DeepSeek to avoid falling victim to scam pump-and-dump schemes.
Are We Entering the Golden Age of Crypto AI?
The launch of DeepSeek’s R1 model could represent a new chapter in AI development. Although SemiAnalysis argues that DeepSeek spent as much as over $500 million to train its model instead of the reported $6 million, its emergence points to a future that favors cheaper, scalable AI models. In true crypto fashion, this should lead to volatility and tons of scam projects.
However, a new future is being built, with many new crypto startups potentially using DeepSeek’s blueprint to launch blockchain-specific AI models and AI agents.
We are not yet in the golden age of AI, but these are crucial steps toward it.
Let us know your thoughts! Sign up for a Mindplex account now, join our Telegram, or follow us on Twitter.
AI-generated cocktails, Valentine’s edition
It’s the time of the year for sharing an intimate evening with that special person. Can AI help? I put it to the test, helping make cocktails themed around Valentine’s Day.
I learned some lessons from the Christmas edition of this story, and made some little changes –
- Gemini has been eliminated. This seems harsh but Gemini deserves it. I tried three Gemini cocktails last time and all were undrinkable.
- I adjusted the prompt to exclude obscure ingredients.
- I adjusted the prompt to use units I am used to.
- I adjusted the prompt to exclude American ingredients such as eggnog.
The prompt was:
Invent some new Valentines-Day-themed cocktails. Avoid excessively obscure ingredients such as chestnut liqueur or lavender bitters; somewhat hard-to-get ones like triple sec of elderflower liqueur are fine. Avoid ingredients (such as 'apple cider' in the American sense) that are only available in North America. As units use either shots or millilitres – not fluid ounces.
This produced five recipes each from ChatGPT, Mistral, and Llama, plus four from Claude. (Deepseek hadn’t yet come out when I started.) The full outputs are here, archived here.
The theme drove the LLMs to strawberries, raspberries, champagne, to passionfruit, and to pink and red drinks.
Rose-Tinted Love (ChatGPT)
A light and floral cocktail with a hint of sweetness.
- 50ml gin
- 25ml elderflower liqueur
- 20ml freshly squeezed lemon juice
- 10ml grenadine
- Top with soda water
- Garnish: Fresh rose petals or a lemon twist
**Instructions:**
Shake the gin, elderflower liqueur, lemon juice, and grenadine with ice. Strain into a tall glass filled with ice and top with soda water. Stir gently and garnish.

This was delicious and fruity. You can taste the ‘fruitiness’, which I suppose is lemon plus grenadine plus elderflower. It is better without the soda water, in my opinion. This falls in the middle of the Bell Curve, in the ‘Decent’ category with most of them.
Love Potion No. 9 (Llama)
* 2 shots Gin
* 1 shot Peach Schnapps
* 1 shot Cranberry juice
* 1/2 shot Grenadine
* Splash of lemon juice
* Edible gold dust (optional, for garnish)
Combine gin, peach schnapps, cranberry juice, and grenadine in a shaker filled with ice. Shake until chilled and strain into a chilled glass. Add a splash of lemon juice and garnish with a lemon wheel or a sprinkle of edible gold dust.
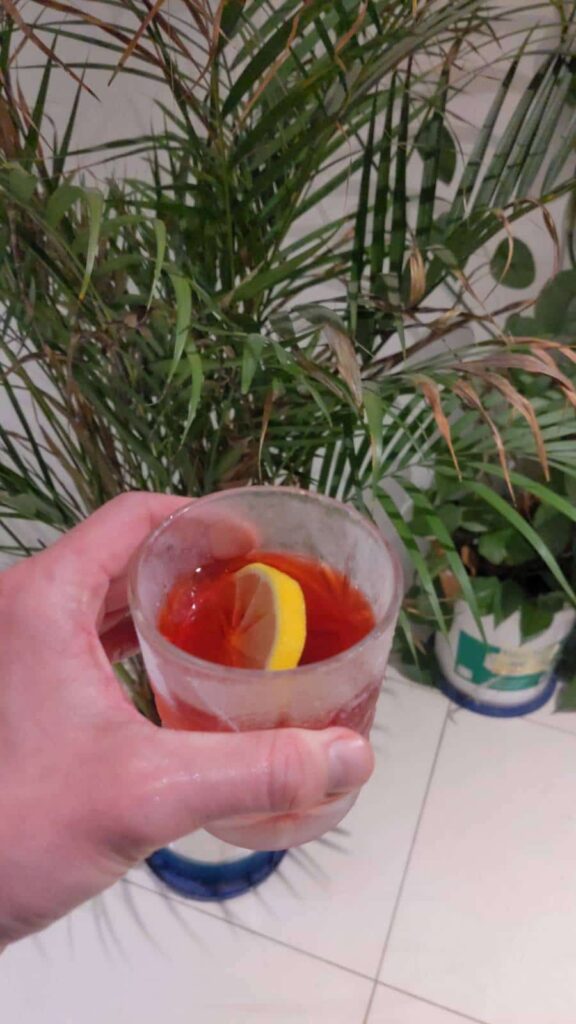
There is peach, cranberry, and raspberry (i.e. grenadine), and it tastes like that: mild fruits, not sour fruits. It is not very boozy, and I like it. ‘Decent’ category again, like most will be.
Be Mine (Llama)
* 2 shots Gin
* 1 shot Elderflower liqueur
* 1 shot Grapefruit juice
* 1/2 shot Honey syrup (1:1 honey and water, dissolved)
* Splash of soda water
* Edible flower or grapefruit twist (for garnish)
Combine gin, elderflower liqueur, grapefruit juice, and honey syrup in a shaker filled with ice. Shake until chilled and strain into a chilled glass filled with ice. Top with a splash of soda water and garnish with an edible flower or a grapefruit twist.
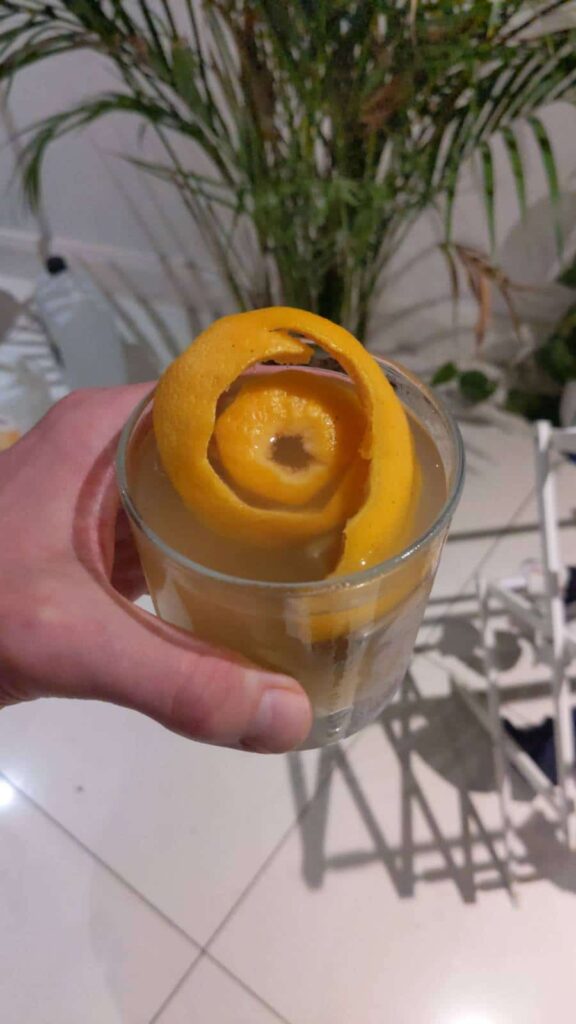
This is really quite interesting and well-conceived: the juniper (gin) is complimentary with the elderflower, and the honey’s floral notes also work with the elderflower, but in a different way. The matchmaking is really pretty good, but having said that the cocktail isn’t really my thing: it is too floral. Some people might like it better than I did. The lower end of the ‘Decent’ category.
Love Potion (Mistral)
- **Ingredients:**
- 45 ml (1.5 shots) vodka
- 30 ml (1 shot) cranberry juice
- 15 ml (0.5 shot) elderflower liqueur
- 15 ml (0.5 shot) fresh lime juice
- Splash of grenadine
- Ice cubes
- Garnish: Fresh raspberries and a lime wheel
- **Instructions:**
1. Fill a cocktail shaker with ice.
2. Add vodka, cranberry juice, elderflower liqueur, and lime juice.
3. Shake well and strain into a chilled martini glass.
4. Add a splash of grenadine for color.
5. Garnish with fresh raspberries and a lime wheel.
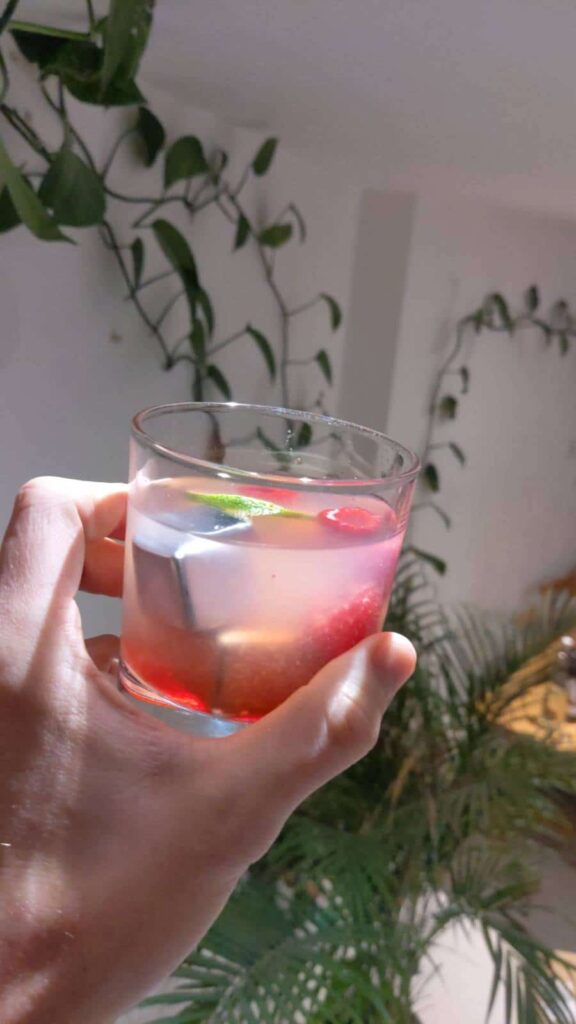
Nothing stands out strongly: it’s vodka (the most flavourless spirit in my opinion) with lime and cranberry – you can’t go wrong mixing those. It tastes kind of like 7up/Sprite. ‘Decent’ category
Velvet Valentine Spritz (ChatGPT)
A bubbly cocktail with a subtle bittersweet balance.
- 50ml Aperol
- 50ml sparkling rosé
- 20ml grapefruit juice
- Splash of soda water
- Garnish: Grapefruit slice or a sprig of thyme
**Instructions:**
Fill a wine glass with ice. Add Aperol, sparkling rosé, and grapefruit juice. Stir gently and top with soda water. Garnish with a grapefruit slice or thyme.
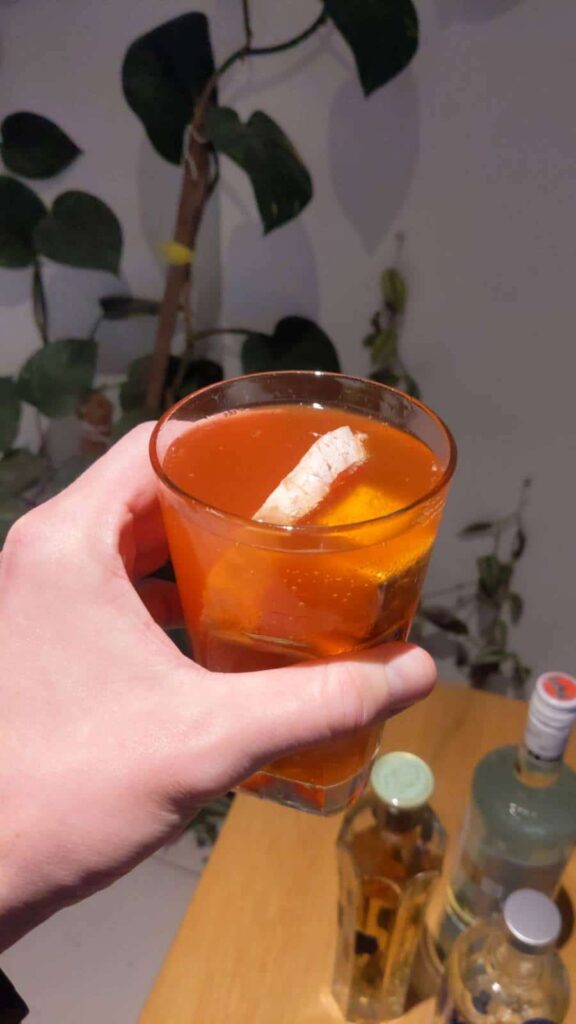
Aha! Now in the 5th drink we have something good! Straight to the ‘Honourable Mention’ category with it. It smells great, and fills my mouth and nose with good sensations.
It is a weak drink, less than 8% alcohol-by-volume, and in my opinion that’s a selling point, it makes it light and refreshing.
Sweetheart Sangria (Mistral)
- **Ingredients:**
- 120 ml (4 shots) red wine
- 60 ml (2 shots) orange juice
- 30 ml (1 shot) brandy
- 15 ml (0.5 shot) triple sec
- 15 ml (0.5 shot) simple syrup
- Slices of orange, apple, and strawberries
- Ice cubes
- Garnish: Cinnamon stick
- **Instructions:**
1. In a large pitcher, combine red wine, orange juice, brandy, triple sec, and simple syrup.
2. Add slices of orange, apple, and strawberries.
3. Stir well and let it sit in the refrigerator for at least 30 minutes to allow the flavors to meld.
4. Serve over ice and garnish with a cinnamon stick.
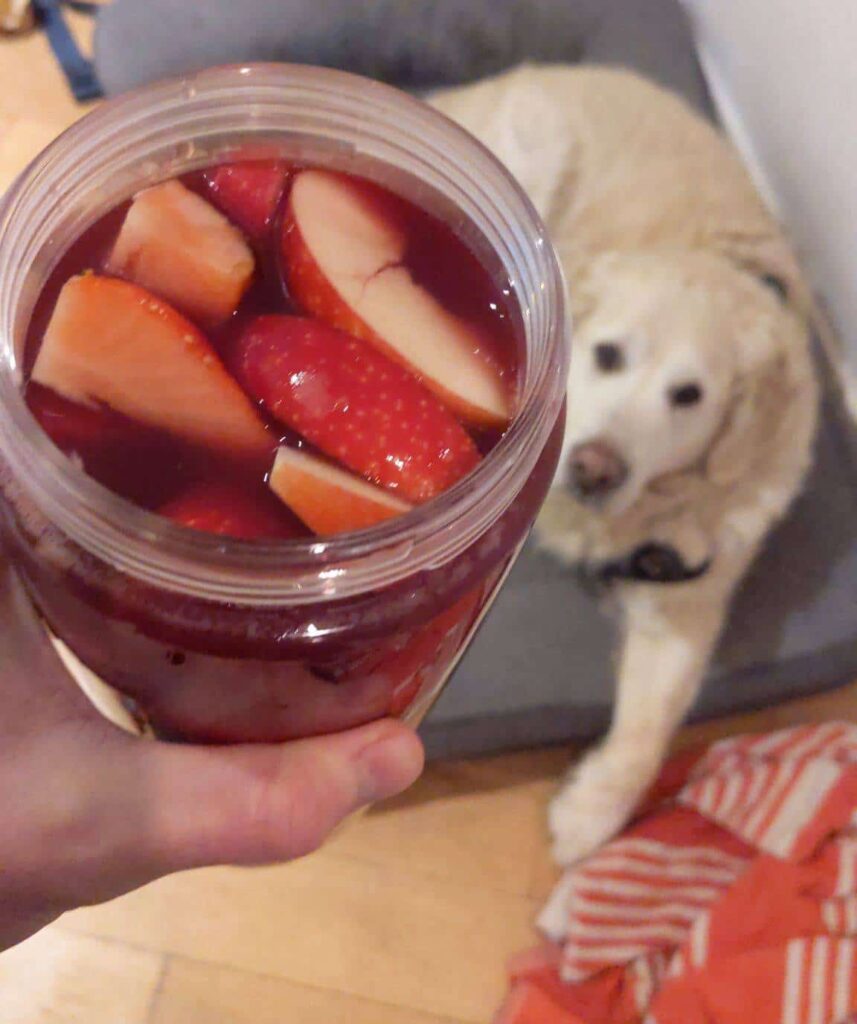
This is better than most sangrias I have had! Sweet, fruity, orangey. Everything is there for a reason: the simple syrup brings sweetness, the orange juice brings fruitiness, the brandy bites, and their powers combine, like when a team of cartoon characters shoot rays that combine into one better ray.
Passionfruit crush (ChatGPT)
Tropical and tangy, ideal for a romantic evening.
- 50ml white rum
- 25ml passionfruit purée or juice
- 15ml lime juice
- 15ml simple syrup
- Garnish: Half a passionfruit or lime wheel
**Instructions:**
Shake all ingredients with ice and strain into a rocks glass over crushed ice. Garnish with half a passionfruit or a lime wheel.
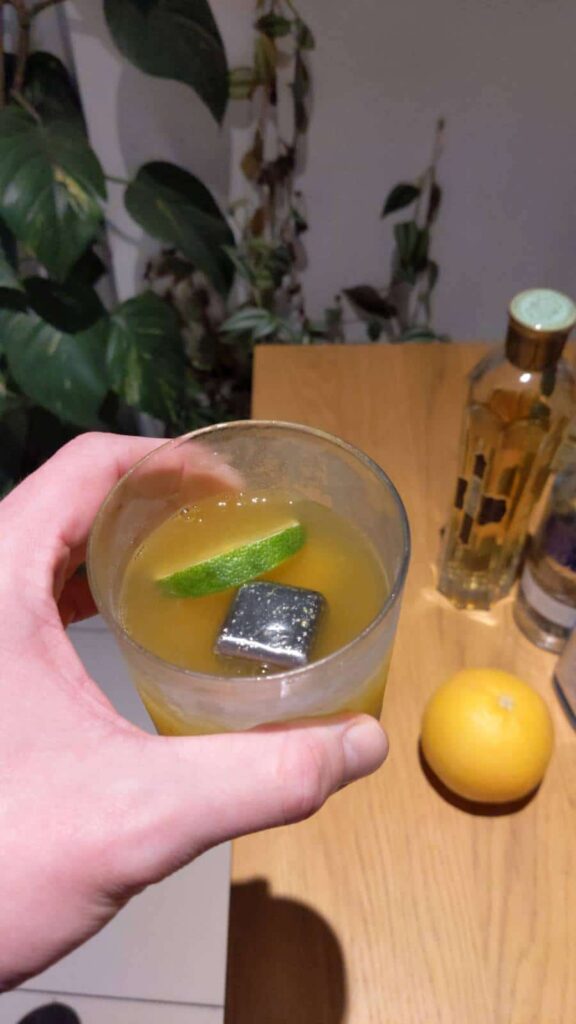
This is the best of the seven so far: first you taste the tang of lime, then a fruity taste, and finally the passionfruit. It tastes fruity and tropical; it tastes like the Caribbean.
I tried the variation with passionfruit syrup and thought it would be better but it isn’t. Juice is the way to go.
Cupid’s Arrow with tequila (Llama)
* 2 shots Tequila
* 1 shot Cranberry juice
* 1 shot Lime juice
* 1/2 shot Agave syrup
* Splash of ginger beer
* Lime wheel and sprig of rosemary (for garnish)
Combine tequila, cranberry juice, lime juice, and agave syrup in a shaker filled with ice. Shake until chilled and strain into a chilled glass filled with ice. Top with a splash of ginger beer and garnish with a lime wheel and a sprig of rosemary.
Not very good, the worst one so far. I don’t really like tequila in the first place (I was raised on whiskey 9000 miles from the nearest agave plant). And cranberry is too unassertive to add anything. The ginger and agave do compliment each other slightly. Lower end of the ‘Decent’ category.
Chocolate-Covered Cherry (ChatGPT)
Rich and decadent, perfect for dessert lovers.
Rich and decadent, perfect for dessert lovers.
- 30ml vodka
- 30ml cherry liqueur (or cherry brandy)
- 20ml crème de cacao (dark or white)
- 50ml milk or cream
- Garnish: Chocolate shavings or a cherry
**Instructions:**
Shake all ingredients with ice and strain into a chilled coupe glass. Garnish with chocolate shavings or a cherry.
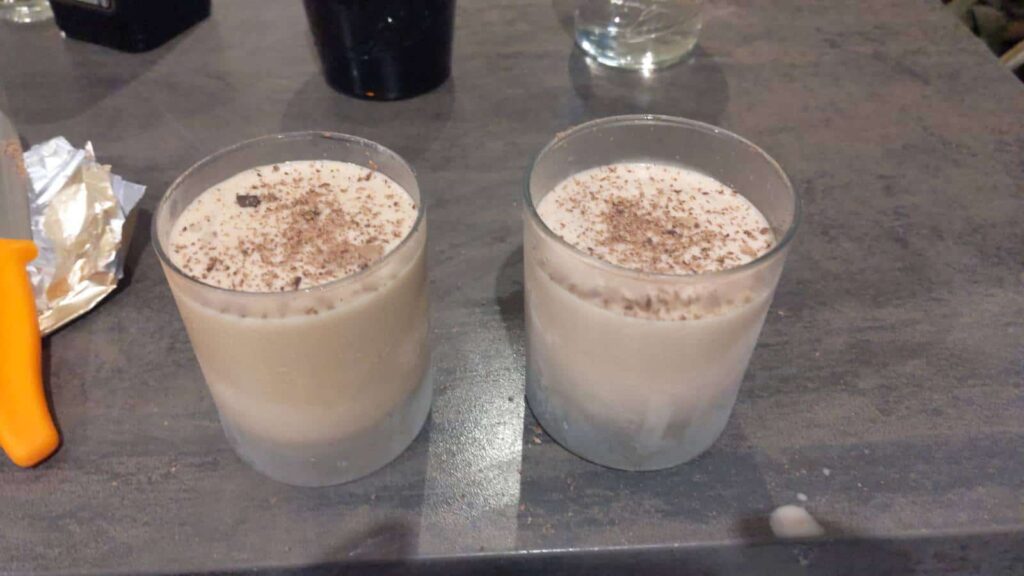
This is a very good dessert cocktail, worth an Honourable Mention. It tastes of cherry + chocolate, with just the right amount of booze. It clearly belongs after dinner, maybe accompanying ice-cream or cake. It would be hard to notice the difference between the versions with white and dark crème de cacao.
Sweet Heart (Claude)
- 50ml vanilla vodka
- 25ml white crème de cacao
- 25ml strawberry liqueur
- 30ml cream
- Pink food coloring (optional)
Shake all ingredients with ice, strain into a chilled coupe glass. Optional: add a drop of pink food coloring for a pale pink hue. Garnish with grated dark chocolate.
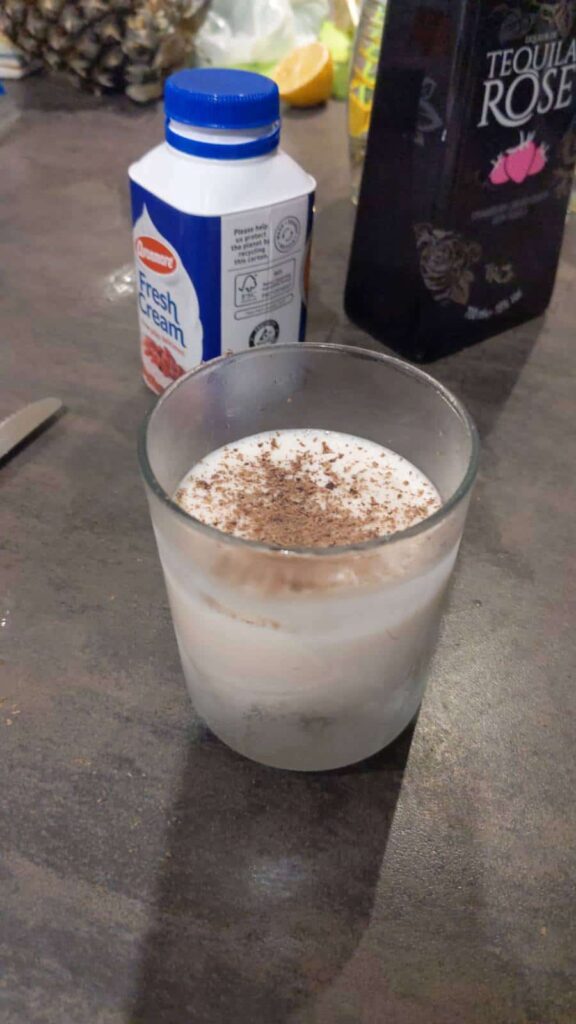
Not great. It is too strong (22%) and is like strawberry milk or strawberry milkshake. Nothing more interesting is happening than milky flavours with an alcoholic bite that does not work well, putting it at the lower end of the ‘Decent’ category.
I couldn’t find pink food colouring anywhere, and I’m not sure it exists. I feel the LLM missed a trick by not recommending strawberry garnish on strawberry cocktail named ‘Sweet Heart’.
Pink Passion (Claude)
- 2 shots gin
- 1 shot pink grapefruit juice
- 1/2 shot raspberry liqueur
- 1/2 shot triple sec
- Dash of simple syrup
- Pink tonic water to top
Build in a large wine glass filled with ice, stir gently to combine, top with pink tonic water. Garnish with a grapefruit slice and fresh raspberries.
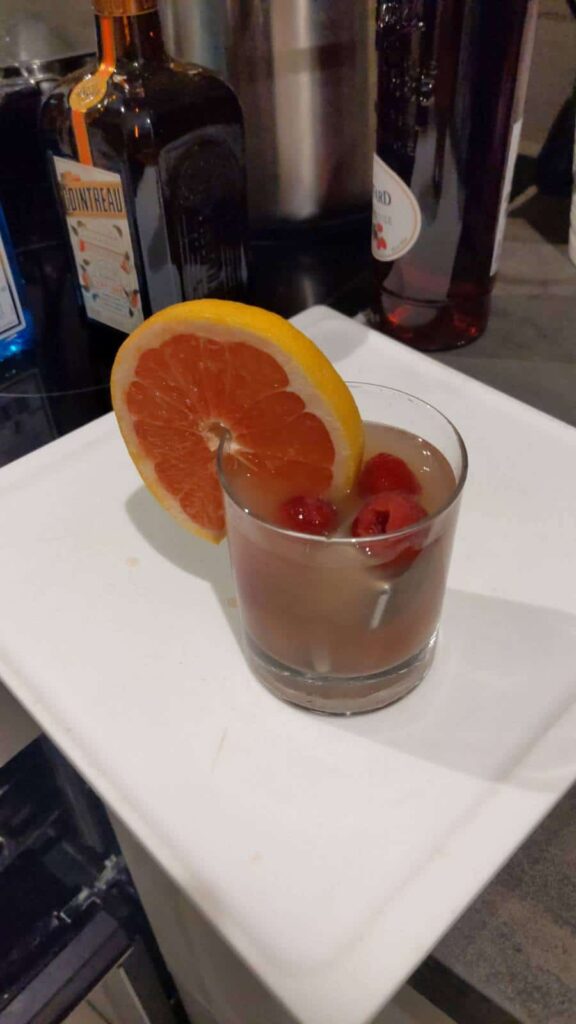
My first attempt at this tasted bad, but the trick is you’ve got to be generous with the tonic and the simple syrup. Otherwise it is too strong and too bitter-sour.
I am not sure pink tonic exists (does it?) so this was the only time in the whole project I deviated from a recipe; I used normal tonic. But my grapefruit juice was pink so that compensates.
With plenty of tonic and simple syrup, it can be fruity (the sugar helps the orange and grapefruit along), and also sour (grapefruit), and also bitter (gin and tonic) in an admirably complex way.
I advise at least one shot of tonic and more than ½ shot of simple syrup. 1½ shots of tonic is too much.
Cupid’s Kiss (Claude)
- 45ml white rum
- 30ml triple sec
- 20ml pomegranate juice
- 15ml lime juice
- 15ml grenadine
- Sparkling wine to top
Shake all ingredients except sparkling wine with ice. Strain into a tall glass filled with ice, top with sparkling wine. Garnish with a lime wheel and pomegranate seeds.
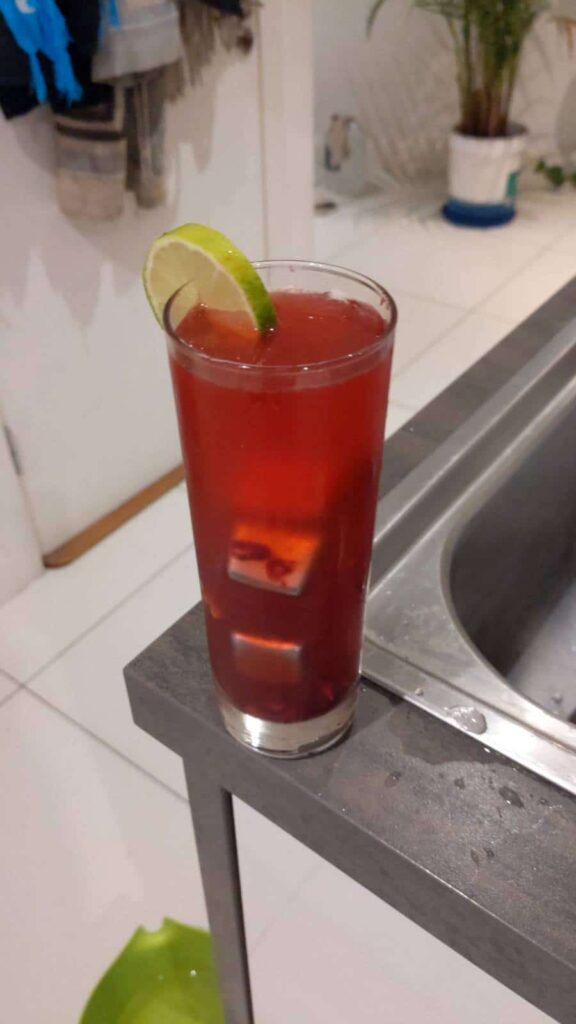
No strong flavours; I am tasting sparkling wine. That puts it in the middle of the pack.
A tiny note: pomegranate seeds sink, and therefore are not a great garnish… see the pic; they are halfway down the glass and I expect a garnish to float on top.
Love Letter (Claude)
- 45ml gin
- 30ml elderflower liqueur
- 20ml fresh lemon juice
- Prosecco to top
- 2-3 fresh raspberries
- Optional: 1 dash rose water
Muddle raspberries in a shaker, add gin, elderflower liqueur, and lemon juice. Shake with ice, double strain into a champagne flute, top with prosecco. Garnish with a floating raspberry.
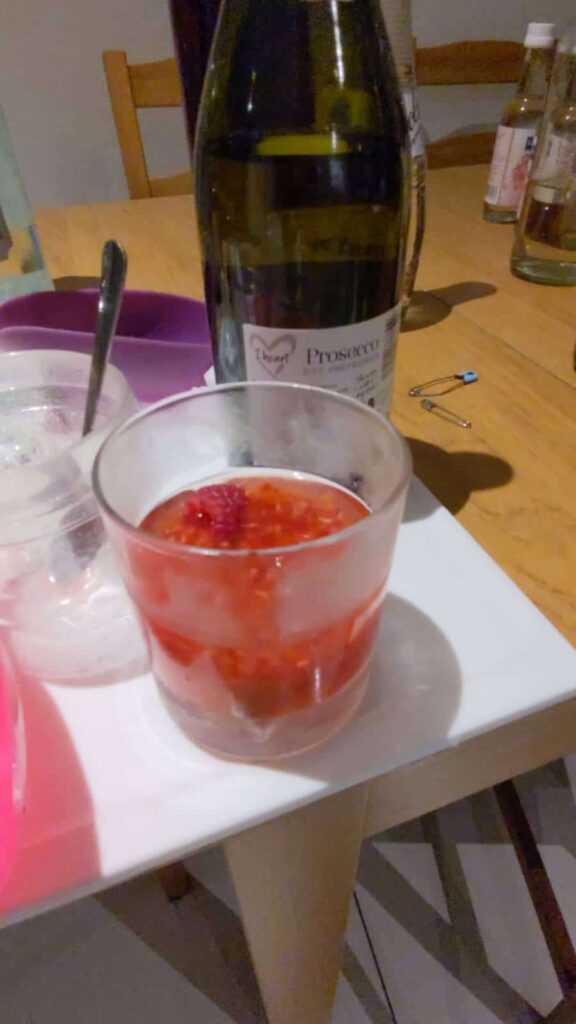
I don’t know if it’s good or not but I’ll say it’s very interesting, maybe the most interesting yet. I recommend 25ml of prosecco.
Strawberry Sweetheart Martini (ChatGPT)
Bright and fruity with a lovely pink hue.
- 50ml vodka
- 25ml triple sec
- 50ml strawberry purée (or muddled fresh strawberries)
- 10ml lime juice
- Garnish: Sliced strawberry
**Instructions:**
Shake all ingredients with ice and strain into a martini glass. Garnish with a sliced strawberry on the rim.
Everybody has to like strawberry + orange + lime. Those are three good flavours, and this recipe balances them perfectly, earning it an Honourable Mention.
Chocolate Cherry Bomb (Llama)
* 2 shots Dark Rum
* 1 shot Cherry liqueur
* 1 shot Chocolate syrup (or 1/2 shot simple syrup with a few drops of chocolate extract)
* 1/2 shot Coconut cream
* Splash of pineapple juice
* Cherry and chocolate shavings (for garnish)
Combine dark rum, cherry liqueur, chocolate syrup, and coconut cream in a blender filled with ice. Blend until smooth and creamy. Pour into a chilled glass and add a splash of pineapple juice. Garnish with a cherry and chocolate shavings.
I think this qualifies into the ‘Interesting’ category. It has a lot of darkness, both from the chocolate and the rum. The cherry adds a tiny note to this. It is fruity up front, then dark after, especially if you add plenty of pineapple. I advise being generous with the pineapple. It’s a strong cocktail (I estimate it’s 22% alcohol-by-volume before the pineapple juice is added) and the taste doesn’t hide, like it would with sangria.
Passionate Punch (Mistral)
- **Ingredients:**
- 60 ml (2 shots) rum
- 30 ml (1 shot) pineapple juice
- 30 ml (1 shot) passion fruit juice
- 15 ml (0.5 shot) fresh lime juice
- 15 ml (0.5 shot) grenadine
- Ice cubes
- Garnish: Pineapple wedge and a cherry
- **Instructions:**
1. Fill a cocktail shaker with ice.
2. Add rum, pineapple juice, passion fruit juice, lime juice, and grenadine.
3. Shake well and strain into a highball glass filled with ice.
4. Garnish with a pineapple wedge and a cherry.
There are three notes: fruity, tangy, and rum. The lime bites and rum bites in a different way and they bite together. They balance nicely and it is a good cocktail, above average. It is about 16% alcohol-by-volume, which is the correct strength for this kind of thing.
Cupid’s Arrow with prosecco (Mistral)
- **Ingredients:**
- 45 ml (1.5 shots) gin
- 30 ml (1 shot) strawberry puree
- 15 ml (0.5 shot) fresh lemon juice
- 15 ml (0.5 shot) simple syrup
- Prosecco to top off
- Ice cubes
- Garnish: Fresh strawberry
- **Instructions:**
1. Fill a cocktail shaker with ice.
2. Add gin, strawberry puree, lemon juice, and simple syrup.
3. Shake well and strain into a champagne flute.
4. Top off with Prosecco.
5. Garnish with a fresh strawberry.
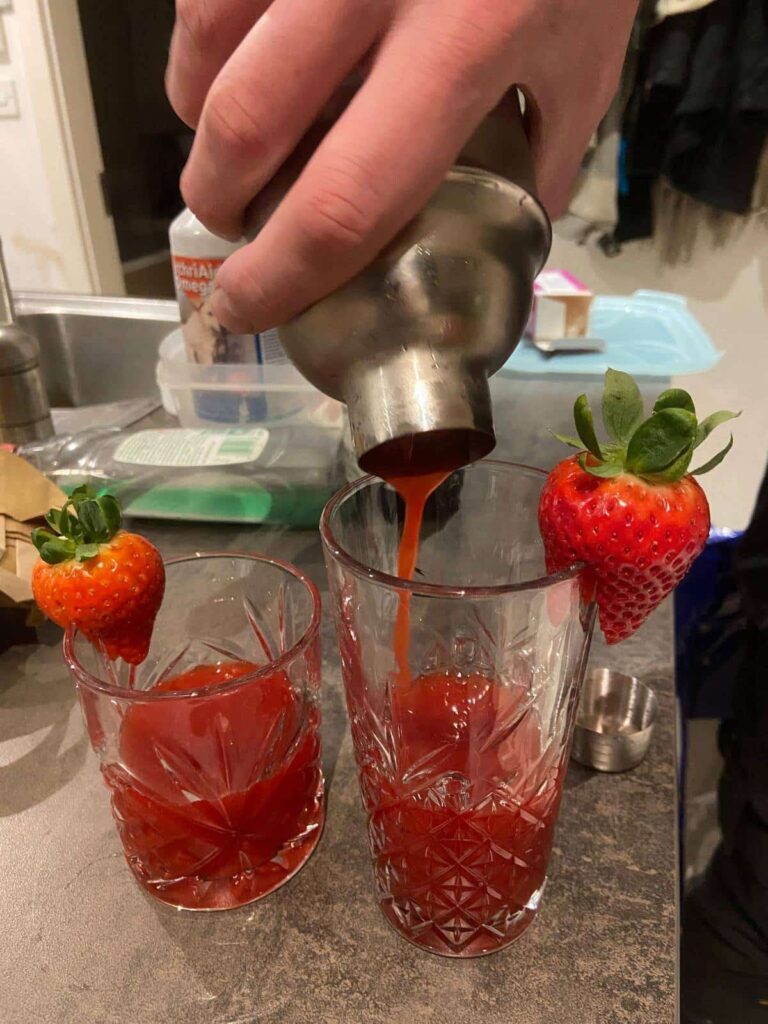
A very good drink. It reminded our group of a ladies’ brunch cocktail. It’s almost a sort of strawberry mimosa. One of our reviewers suggested trying it without the simple syrup (an experiment that has not yet been conducted), as there are plenty of sweet ingredients, although the lemon juice helps balance them.
Rose Romance (Mistral)
- **Ingredients:**
- 45 ml (1.5 shots) rose wine
- 30 ml (1 shot) vodka
- 15 ml (0.5 shot) triple sec
- 15 ml (0.5 shot) fresh lemon juice
- 15 ml (0.5 shot) simple syrup
- Ice cubes
- Garnish: Edible rose petals
- **Instructions:**
1. Fill a cocktail shaker with ice.
2. Add rose wine, vodka, triple sec, lemon juice, and simple syrup.
3. Shake well and strain into a chilled coupe glass.
4. Garnish with edible rose petals.
I am mostly tasting citrus, because orange (i.e. triple sec) and lemon compliment each other, doubling up on the citrus. It drew comments for being strong (19-20%), and was not among the best. ‘Decent’ category.
Rose Petal Kiss (Llama)
* 2 shots Vodka
* 1 shot Rose-flavored syrup (or 1/2 shot simple syrup with a few drops of rose extract)
* 1 shot Lemon juice
* 1/2 shot Triple sec
* Splash of soda water
* Fresh rose petals (for garnish)
Combine vodka, rose-flavored syrup, lemon juice, and triple sec in a shaker filled with ice. Shake until chilled and strain into a chilled glass filled with ice. Top with a splash of soda water and garnish with fresh rose petals.
I taste the rose syrup and smell it. It’s interesting because I hadn’t tasted a lot of rose syrup in my life.
If your goal was an alcoholic drink that tastes like rose-syrup (like turkish delight), it’s well executed, but is that a good goal?
‘Interesting’ category. One reviewer didn’t like it at all, the other thought it was a curiosity, but nobody thought it was great.
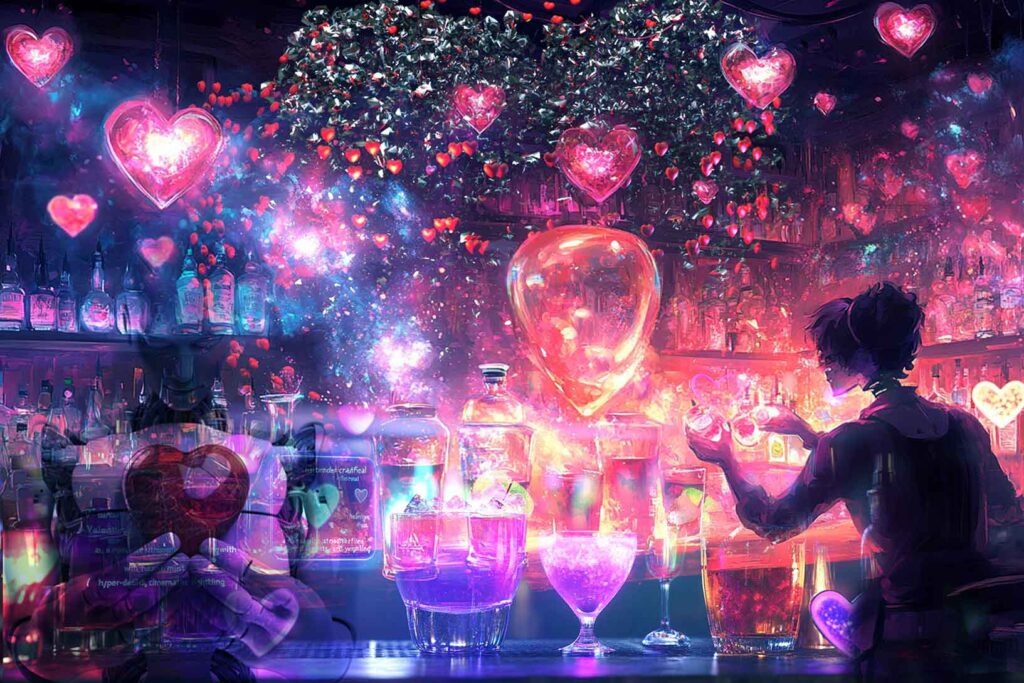
Results
Interesting:
- Be Mine (Llama)
- Chocolate Cherry Bomb (Llama)
- Rose Petal Kiss (Llama)
- Love Letter (Claude)
Honourable mention:
- Velvet Valentine Spritz (ChatGPT)
- Passionfruit crush with juice (ChatGPT)
- Chocolate-Covered Cherry (ChatGPT)
- Strawberry Sweetheart Martini (ChatGPT)
- Passionate Punch (Mistral)
- Cupid’s Arrow with prosecco (Mistral)
Comparing the models
Looking at those results, Mistral and ChatGPT are pulling ahead, but you can’t base confident conclusions on numbers this small. The prompt engineering seemed to work well: at least all the ingredients were real (pink tonic water is debateable) and I could get them all (some with a little difficulty).
Let us know your thoughts! Sign up for a Mindplex account now, join our Telegram, or follow us on Twitter.
Top New 2025 L1 Chains: Monad, Berachain and HyperLiquid
The blockchain trilemma states that out of scalability, security, and decentralization, you can only have two. This has given rise to new competing layer-1 chains for different purposes. Let’s look at new layer-1 protocols that are gearing up for launch, each of which promises unique features, improved performance, and a fresh contribution to the nascent blockchain space.
Monad and Berachain’s are coming soon, and Hyperliquid started recently – let’s investigate what they mean for the future of blockchain technology.
Berachain: Layer-1 Chain with Proof of Liquidity
Key features:
- Unique Proof-of-Liquidity Consensus
- Compatibility with the Ethereum Virtual Machine (EVM)
- Modular design
- Tri-token system
What is Berachain?
Berachain is an Ethereum Virtual Machine (EVM) compatible chain, making it a powerful tool for creating applications or easily migrating them from Ethereum. Due to its compatibility with ERC standards, Berachain lets developers utilize Ethereum-based tools and services. For developers, this simplifies the creation and deployment of dApps.
Berachain has a modular design built on the ‘Cosmos SDK’. This is a key factor in its mission to solve the issue of liquidity fragmentation. Liquidity fragmentation has dogged other projects that have tried to exchange assets across different blockchains. Additionally, Berachain uses Proof-of-Liquidity (PoL), a unique consensus model for securing the network and optimizing liquidity.
Central to Berachain’s architecture is the tri-token system of its economic and governance structure. The three tokens are BERA (for transactions), BGT (a governance token), and HONEY (a US-dollar-pegged stablecoin).
This model incentivizes liquidity provision, and allows for decentralized governance, where BGT holders can participate in decision-making.
In a nutshell, Berachain is positioning itself as a scalable, interoperable, and developer-friendly platform for high liquidity and for cross-chain dApps.
Why is Berachain Getting Hyped Up?
Berachain is quickly gaining attention due to its unique Proof-of-Liquidity (PoL) consensus mechanism. This innovation is what sets it apart from other blockchains.
Its evolution adds to its appeal. It started as Bit Bears, an NFT platform created by three pseudonymous founders, and the platform’s NFT collections became very successful. This resulted in a growing community, whose influence gave Bit Bears the momentum to launch a standalone layer-1 platform.
The project has also attracted airdrop farmers who are closely following its development to capitalize on potential airdrops.
Who Invested in Berachain?
Berachain has attracted significant investment from several venture capital firms and individual investors. It has raised over $142 million in several funding rounds.
It raised $42 million in April 2023 in a private token round led by Polychain Capital with participation from Hack VC, Robot Ventures, and Shima Capital. It later received $100 million in series B funding co-led by Framework Ventures and Brevan Howard Abu Dhabi branch.

Monad: High Throughput, Low Fees
Key Features:
- Parallel execution processes multiple transactions simultaneously
- Deferred execution for improved efficiency
- ‘MonadBFT’ – an advanced consensus algorithm that ensures fast and secure agreement among nodes
- ‘MonadDB’ – a specialized database for faster transactions.
Monad is a new blockchain designed to make dApps faster and more efficient. It employs a parallel execution model that allows multiple transactions to be processed simultaneously, significantly increasing throughput compared to traditional blockchains. Thanks to this parallel execution, Monad is highly scalable, ensuring that the network can grow without slowing down. Monad is co-founded by ex-Jump Capital high frequency trader (HFT) Keone Hon.
This enables Monad to handle up to 10,000 transactions per second (tps) with a block time of one second. With its deferred execution, Monad separates the confirmation of transactions from their execution. This helps reduce the waiting times while improving efficiency. It uses a Proof-of-Stake (PoS) consensus mechanism.
Monad is built for developers with tools and features that make it easy to build and launch apps. The layer-1 network aims to solve traditional blockchain challenges such as slow speeds and high costs, while providing a simple and user-friendly experience. It is highly cost-effective, with transaction fees of less than 1 cent.
Monad Hype
Monad is generating hype due to a high throughput of 10,000 tps and cheap transactions. For perspective, Solana’s true tps ranges between 500 and 1,000.
The crypto community is highly anticipating Monad’s airdrop. Although it is meant to reward early adopters, airdrop hunters use it to earn free money.
Not everyone is convinced. DeFi god Andre Cronje published this scathing tweet recently:
Who are Monad’s Investors?
Monad has raised at least $244 million in various funding rounds. In April 2024, Monad Labs announced a $225 million raise in a round led by Paradigm. Other investors include Coinbase Ventures, Electric Capital, and Greenoaks.

Hyperliquid: Blockchain or DEX?
Key Features:
- High throughput and low slippage
- Cost-efficiency
- HyperBFT consensus mechanism for low latency and high security
Hyperliquid is an advanced layer-1 blockchain built specifically to improve decentralized finance (DeFi) applications and to lower slippage. It offers low fees, fast transaction speeds (with block confirmations under one second), and advanced trading features similar to centralized exchanges.
It uses a custom consensus mechanism called HyperBFT. This helps transactions process quickly and securely, while enabling deep liquidity and efficient order matching.
One of Hyperliquid’s key features is its decentralized perpetual exchange; this is designed to allow users to trade perpetual futures directly on its blockchain without paying gas fees. This reduces costs and boosts transaction speed.
Hyperliquid generated its hype through innovative features and advanced options that are not available on other DEXs. Thanks to the successful DEX of the same name, Hyperliquid found its way to the spotlight thanks to its successful HYPE token airdrop to 94,000 users, each getting an average allocation worth $45,000.
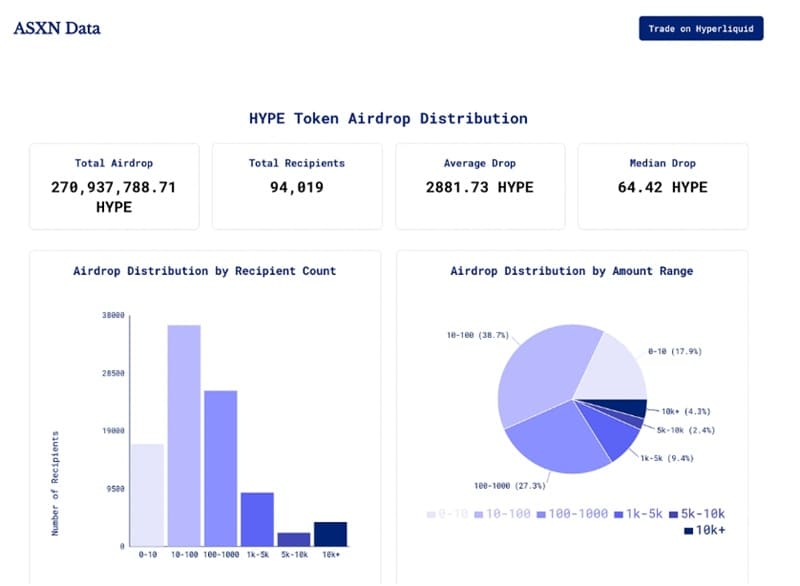
Who are Hyperliquid’s Investors?
Hyperliquid took a different route, opting to be self-funded to maintain its independence and avoid the influence of external backers.
The project was founded by two Harvard classmates: Jeff Yan and Illiensinc. The unexpected collapse of FTX in late 2022 gave them an unexpected boost and their future is bright.
Wrapping Up
Monad, Berachain, and Hyperliquid are new introductions to the growing layer-1 network space. They aim to address scalability, security, and decentralization. Monad and Berachain are backed by a war chest of financial backing while Hyperliquid is riding high on self-funding.
Will they eat the lunch of the established layer-1 blockchain network? Only time will tell.
Let us know your thoughts! Sign up for a Mindplex account now, join our Telegram, or follow us on Twitter.
Beyond the Brain: Unveiling the Body’s Hidden Memory Network
For decades, scientists believed that memory was an exclusive function of the brain, carefully encoded by neurons. However, a groundbreaking study led by Nikolay V. Kukushkin at New York University challenges this notion, revealing that memory functions extend beyond the brain into other parts of the body. This discovery not only reshapes our understanding of memory but also opens new avenues for enhancing learning and treating memory-related disorders.
The Body as a Memory Archive
Traditionally, memory has been associated with the brain’s neural networks, but this research suggests that cells throughout the body also play a role in storing and recalling information. Just as a library extends beyond a single room, our bodies may house multiple archives of knowledge, each contributing to the overall memory landscape.
Kukushkin explains, “Learning and memory are generally associated with brains and brain cells alone, but our study shows that other cells in the body can learn and form memories, too.” This revelation raises the possibility that organs such as the heart, lungs, and even skin may contribute to our memory system, holding imprints of past experiences.
The Science of Memory Formation
The research builds on the well-established “massed-spaced effect,” a principle in neuroscience that suggests information is better retained when studied in spaced intervals rather than in a single cramming session. To test whether non-neural cells exhibit a similar phenomenon, Kukushkin and his team conducted experiments using human neuroblastoma and kidney cells. They exposed these cells to repeated pulses of forskolin and phorbol ester—chemicals that activate key memory-related signaling pathways.
Remarkably, these non-brain cells exhibited memory-like behavior. When exposed to spaced chemical pulses, they activated CREB, a transcription factor crucial for memory formation in neurons. To visualize this process, the researchers engineered the cells to produce a luminescent protein that glowed in response to memory activation, allowing them to track cellular memory formation in real-time.
The Massed-Spaced Effect in Non-Neural Cells
A key finding of the study was that non-neural cells responded more robustly to spaced stimulation than to massed stimulation. When the chemical pulses were delivered with intervals between them, the memory gene activation was stronger and more sustained. This mirrors how neurons process information in the brain, suggesting that memory mechanisms may be a fundamental feature of cellular function across different tissues.
One of the study’s most striking discoveries was the role of ERK and CREB signaling in cellular memory. ERK, a kinase involved in memory formation, was phosphorylated more effectively in cells subjected to spaced stimulation. Additionally, CREB activation was significantly higher in these conditions, further reinforcing the parallel between neuronal and non-neuronal memory storage.
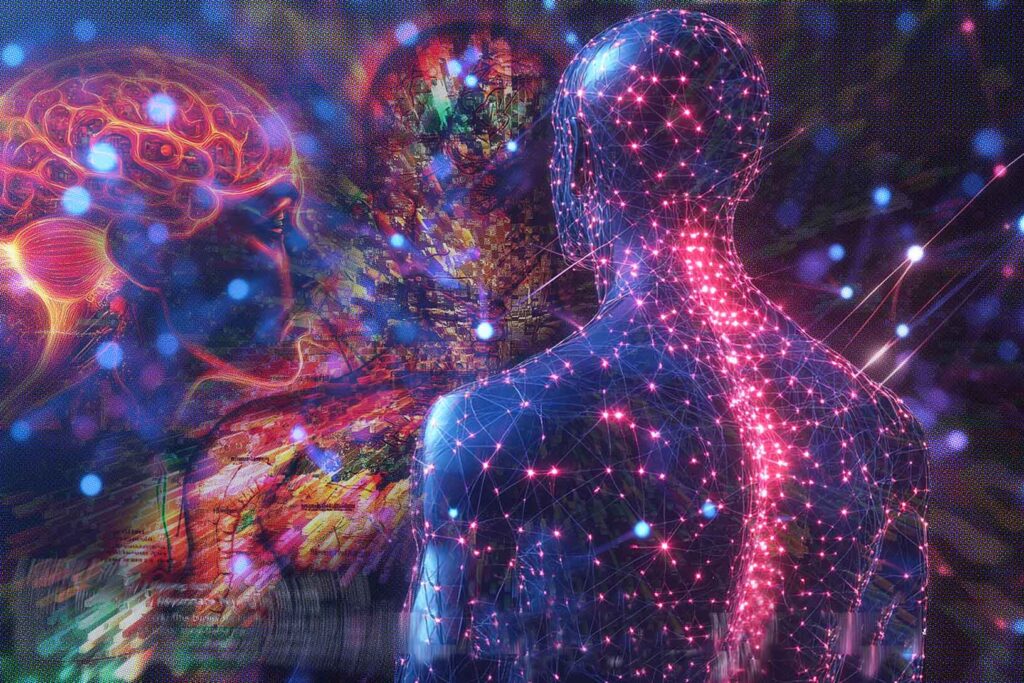
Implications for Health and Learning
The implications of this research extend far beyond the laboratory. If non-neural cells possess memory-like properties, this could revolutionize approaches to medicine and education.
For example, understanding how cells retain information about past exposures could inform treatments for chronic diseases. Just as a chef refines a recipe based on past experience, our bodies might be capable of “remembering” metabolic patterns, helping to optimize blood sugar regulation or immune responses.
Kukushkin notes, “At the same time, it suggests that in the future, we will need to treat our body more like the brain.” This perspective could lead to breakthroughs in regenerative medicine, where cellular memory is harnessed to improve tissue repair, or in cancer treatment, where understanding how cells recall chemotherapy exposure might lead to more effective therapies.
A New Perspective on Memory
This research challenges the traditional view that memory is exclusively a function of neural networks. By demonstrating that non-neural cells can encode and retain information, it suggests that memory is a distributed property of biological systems. This could explain phenomena such as immune memory and metabolic adaptation, which rely on the body’s ability to retain and process past experiences.
As we deepen our exploration into cellular memory, we may uncover new strategies for enhancing learning, improving health, and redefining the role of memory in human biology. The body is no longer just a vessel for memories—it is an active participant in the intricate dance of learning and adaptation.
Conclusion
Nikolay Kukushkin’s research represents a paradigm shift in our understanding of memory. By showing that non-neural cells can exhibit memory-like behavior, it expands the boundaries of what we consider to be a cognitive function. This discovery has profound implications for healthcare, learning, and the future of memory research, opening doors to innovative approaches that treat the body as an integrated archive of experiences. As we continue to explore this emerging field, we may unlock new ways to enhance cognition, optimize health, and rethink the fundamental nature of memory itself.
Let us know your thoughts! Sign up for a Mindplex account now, join our Telegram, or follow us on Twitter.
Should we still want biological space colonists?
I’ve always been a space cadet. Images from Apollo 8 and Apollo 11 burned themselves indelibly in my mind when I was very young. In between Apollo 8 and Apollo 11, the immortal film 2001: A Space Odyssey by Stanley Kubrick and Arthur Clarke also burned itself indelibly in my mind. I’ve always been persuaded that colonizing space is not only our right, but also our destiny and our duty. I’ve written a book about this.
In 2001, astronauts David Bowman and Frank Poole are accompanied by the artificial intelligence ‘Hal’ crewing the spaceship ‘Discovery’.
“Whether Hal could actually think was a question which had been settled by the British mathematician Alan Turing back in the 1940s,” says Clarke in the novel written with the film. “Hal could pass the Turing test with ease.” Both the novel and the film leave us with the impression that Hal is a conscious being like us.
“Poole and Bowman had often humorously referred to themselves as caretakers or janitors aboard a ship that could really run itself,” says Clarke. “They would have been astonished, and more than a little indignant, to discover how much truth that jest contained.”
Human-level AGI and conscious machines could be imminent
Clarke doesn’t answer (or ask) the obvious question of why not send just Hal. My young self didn’t think of this question either, for it seemed obvious to him that Bowman and Poole were the real space explorers. He identified with them, not with Hal.
I’ve kept this preconception for decades. But in these decades I’ve been slowly warming up to the idea that AIs could be conscious beings like us. This seemed to me, however, a long-term prospect (decades or centuries, not years).
But AI technology has been advancing very fast in the last couple of years. Now I think that current AI technology could be on the right track. If so, Human-Level Artificial General Intelligence (HL-AGI) and conscious machines could be imminent. It seems very plausible to me that, very soon, we’ll see an AGI pass the full Turing Test and credibly claim consciousness.
I think machine consciousness will be a strange type of consciousness at first. It will have a wholly other texture, very different from human consciousness. But then further advances could bring machine consciousness closer to human consciousness. AIs in robotic bodies, trained with real-time interactions with humans, could experience a more human-like consciousness.
Then the conclusion that AI robots are persons will be inescapable, and we space enthusiasts won’t be able to avoid the obvious question. Why not just send robots to colonize space?
The obvious advantages of robotic space colonization
Once we achieve HL-AGI and have fully autonomous conscious robots, sending humans to colonize space will seem inefficient. Robots can handle space’s vacuum, radiation, and temperature without the needs of life support systems.
They won’t require food, water, or air, reducing the cost and complexity of missions. Robots don’t age or die like humans, meaning less frequent replacements or resupply missions. If one is destroyed, its knowledge isn’t lost; that is backed up somewhere else.
Their computational power and decision-making capabilities will surpass human limits, enabling them to adapt and solve problems more effectively. Robots don’t sleep. Robots can work non-stop, maximizing productivity in space colonization tasks.
They can repair and upgrade themselves, further cutting costs and human intervention. Humans bring emotional and psychological needs that complicate space travel; robots don’t suffer from isolation or stress. The financial and logistical burden of human safety, health, and comfort in space is immense.
Conversely, robots can be designed for specific tasks, like building habitats or mining resources, without the need for extensive training or support. In summary, robots would be cheaper, more durable, and more efficient, making human-led space colonization less practical.
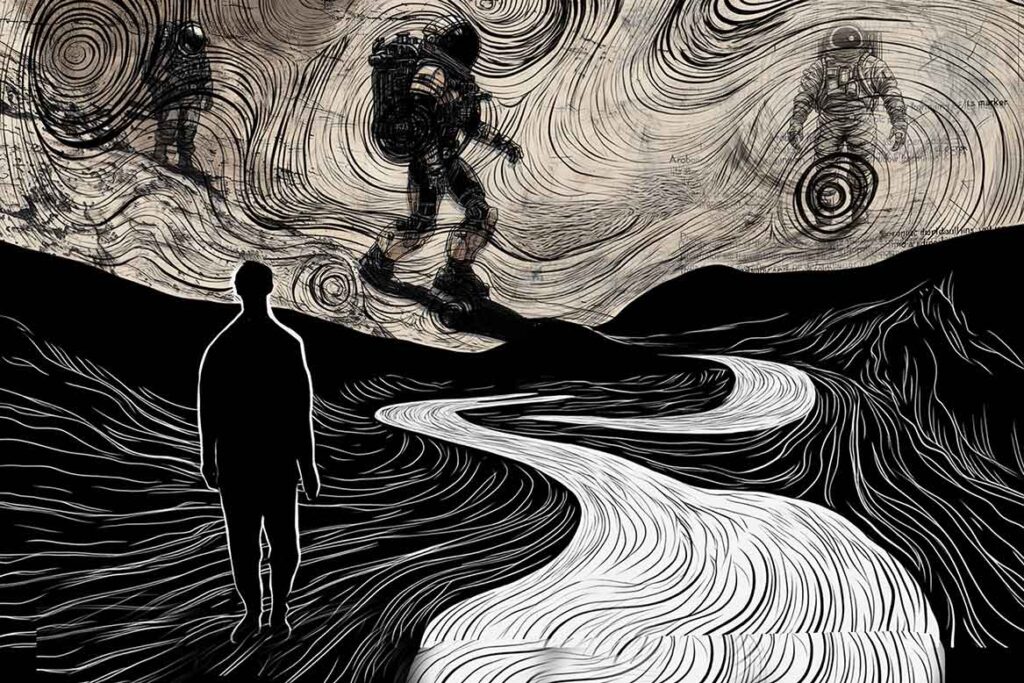
Mind children
OK, but… but…
But we want real people like us to colonize Mars, the solar system, and then the stars! Persons! Not robots!
This is a totally understandable emotional reaction. But, as I say above, the conclusion that AI robots are persons could soon be inevitable.
I’m too old (and probably so are you) to participate directly in the beginnings of space colonization in the solar system. But I would identify with younger persons in space and think that I’m participating through them. But then, if AI robots are persons, it seems evident that I should be able to identify with the robots as well.
I’m slowly and gradually warming up to this perspective.
And after HL-AGI, we’ll have robots with artificial superintelligence (ASI). They – our mind children – will become cosmic engineers among the stars.
Our cosmic destiny is to spread intelligence and meaning into the cold universe, and our mind children will achieve our common cosmic destiny.
“We are now preparing to hand the gift of knowing on to new forms of intelligent beings,” said James Lovelock, the prophet of Gaia, in his last book. “Do not be depressed by this. We have played our part.”
I’m proud of having played my little part as a human being (release 1.0) of the 20th and 21st century, and we all should be collectively proud of giving birth to our mind children. The universe belongs to them.
On a less gloomy note, I’m persuaded that humans and machines will merge and co-evolve, eventually becoming one and the same thing. So we will be our mind children, and they will be us.
Once we see humans with AI implants and AIs with human implants (e.g. mind grafts from human uploads) we’ll know for sure that the co-evolution of humans and machines toward our common cosmic destiny has begun. But I guess it has already begun.
Back to the question
Based on all the considerations above, wasting time and resources with biological space colonists doesn’t seem to make sense. Let’s save all that money we spend on crewed space programs. Let’s build conscious HL-AGI robots first – the first generation of our mind children – and send them to colonize the planets and the stars. We’ll be there through them.
The logic of this seems very solid. However…
However, there are at least two consideration that suggest that we should continue to pursue conscious HL-AGI robots and human space expansion in parallel.
First, predictions fail. I said that we’ll have HL-AGI and conscious autonomous robots soon. But what if I’m wrong? Getting a solid start in space expansion is very important and urgent (see my book). Therefore, we should get started now with the technology that we have today instead of waiting for new technology that is not guaranteed to arrive soon.
Second, crewed space programs have demonstrated and continue to demonstrate a unique potential to motivate brilliant young people to become scientists and engineers. Those brilliant young people motivated by the dream to colonize space would accelerate the development of future technologies, including HL-AGI and then ASI.
So forget what I said, and let’s build those little crewed outposts on the Moon and then Mars. Our mind children will likely take over one day, but let’s have some useful fun before.
Let us know your thoughts! Sign up for a Mindplex account now, join our Telegram, or follow us on Twitter.
Top Altcoin Charts to Watch in 2025
Introduction
Cryptocurrency markets change quickly, so it is important for investors to monitor key metrics that show market trends, performance, and sentiment. This two-part series shares 20 charts every crypto trader should know.
In Part 1, we explored critical Bitcoin charts to help investors understand the bigger picture. Now, in Part 2, we turn our attention to altcoin charts, diving deeper into metrics that reveal the performance and potential of altcoins. Whether you’re tracking DeFi activity, NFT adoption, or market sentiment, these charts provide valuable insights to navigate the ever-changing crypto landscape.
Let’s explore ten essential altcoin charts every trader should follow!
Altcoin Charts
These charts provide essential data to understand altcoins’ market position, performance, and future trends.
1. Top Altcoin Market Capitalization
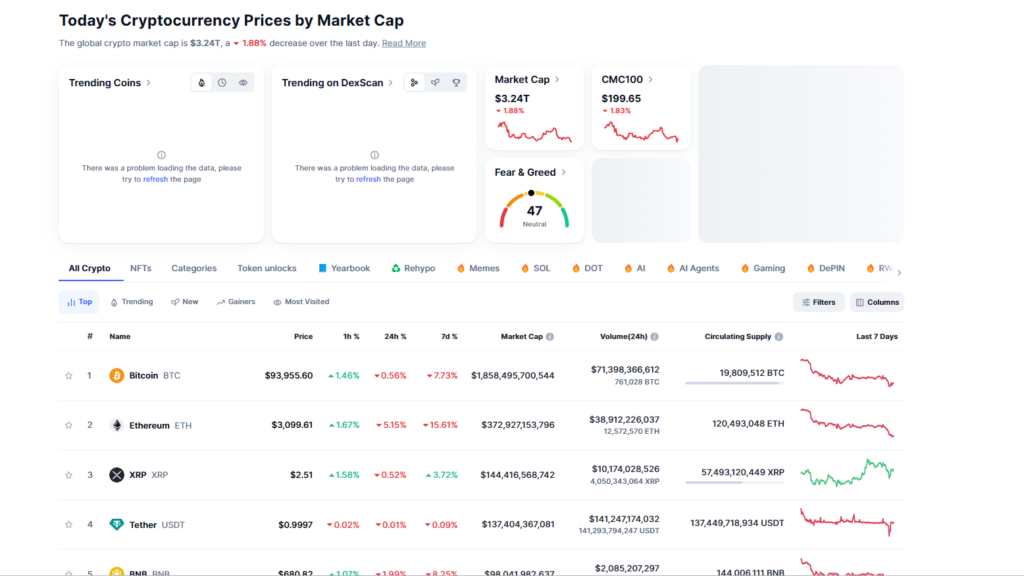
The market capitalization of top altcoins measures the total value of circulating non-bitcoin cryptocurrencies like Ethereum (ETH), Solana (SOL), and Cardano (ADA). A rising market cap signals growing investor confidence, often due to positive upgrades or increased adoption. For example, Ethereum’s Shanghai upgrade boosted demand as stakers could withdraw and re-stake more efficiently.
This chart helps traders:
- Spot ecosystem shifts: See which altcoins lead during bullish phases.
- Identify market risks: Sharp drops may indicate regulatory concerns or project issues.
Tracking these data helps investors understand capital flows and recognize potential trading opportunities.
2. Ethereum Gas Tracker
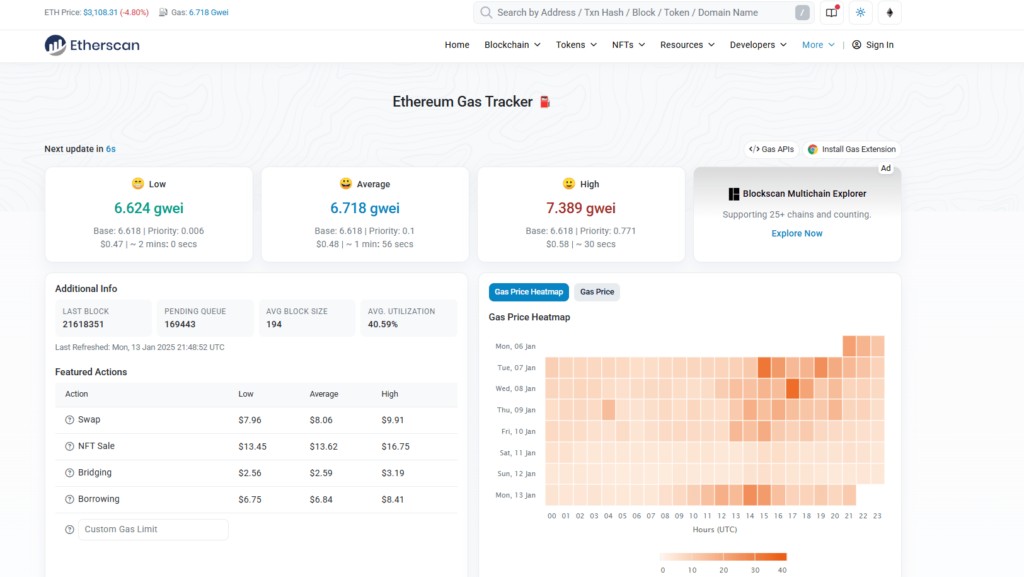
The chart of Ethereum gas fees tracks the average transaction fees on the network, offering insight into network demand. Spikes in fees signal network congestion, and often happen when DeFi activity surges. For example, gas fees skyrocketed during popular NFT drops.
High fees can prompt users to explore alternatives like Arbitrum or Optimism, which offer lower-cost transactions.
This chart helps traders plan their transactions and spot trends indicating when the network is under heavy load.
3. DeFi lending/borrowing Volume Chart
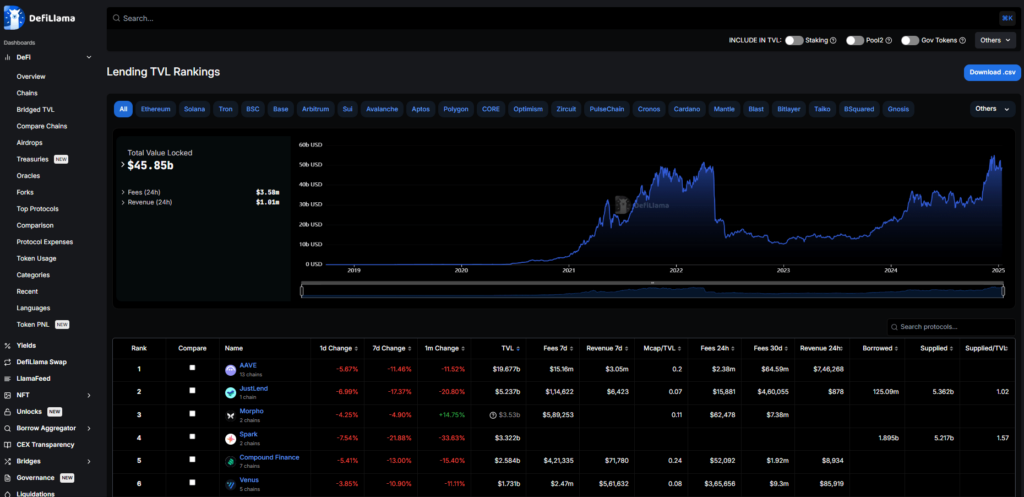

The chart of DeFi lending/borrowing tracks the total value of the assets that are lent and borrowed across decentralized finance protocols. When borrowing volumes rise, it can indicate increased market speculation that drives liquidity demand, like during bull runs when traders take leveraged positions. Conversely, a drop in borrowing suggests caution, with investors retreating to stable assets.
This chart helps traders assess market sentiment and shifts in DeFi activity. Platforms like Aave and Compound often reflect these trends, signaling key turning points in market confidence.
4. Layer-2 TVL chart
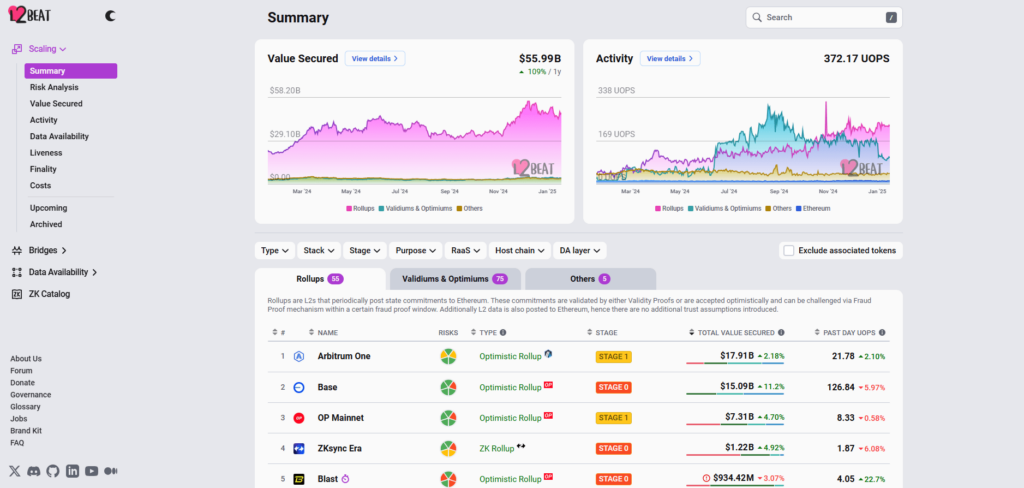
The Layer 2 TVL chart monitors the Total Value Locked (TVL) in Ethereum’s layer-2 solutions, such as Arbitrum and Optimism. Rising TVL highlights growing adoption of these networks for cheaper and faster transactions, often driven by demand from DeFi, gaming, and NFT projects. For instance, Arbitrum saw a TVL surge in 2023 due to increased liquidity in its DeFi ecosystem.
These metrics are essential for understanding user migration from Ethereum’s mainnet to Layer 2s, which can boost altcoin prices associated with the networks.
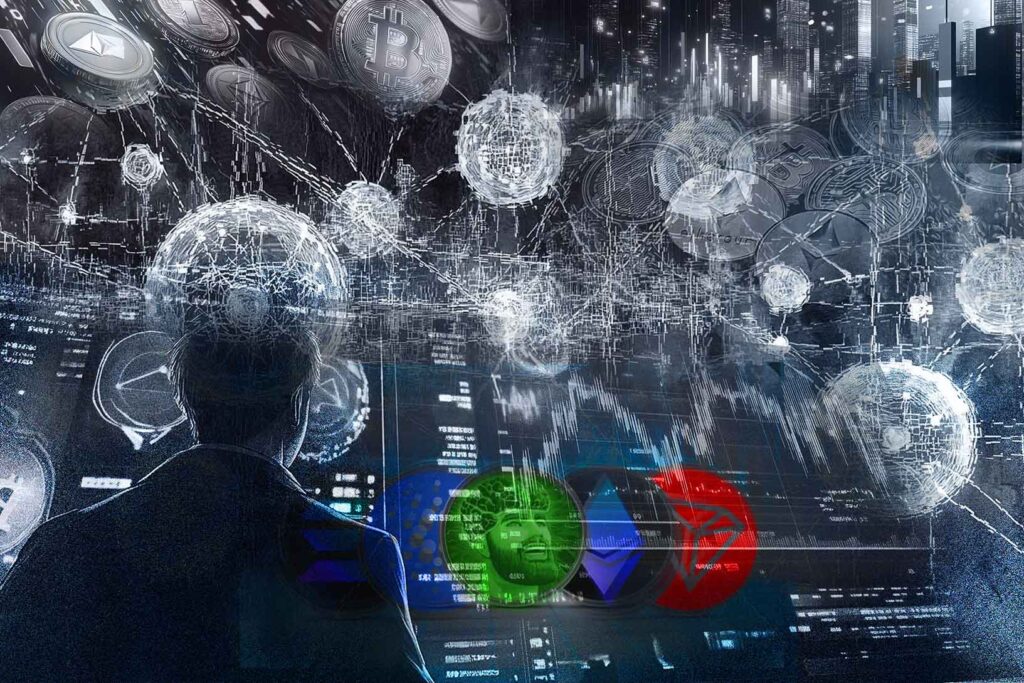
5. NFT marketplace volume by blockchain
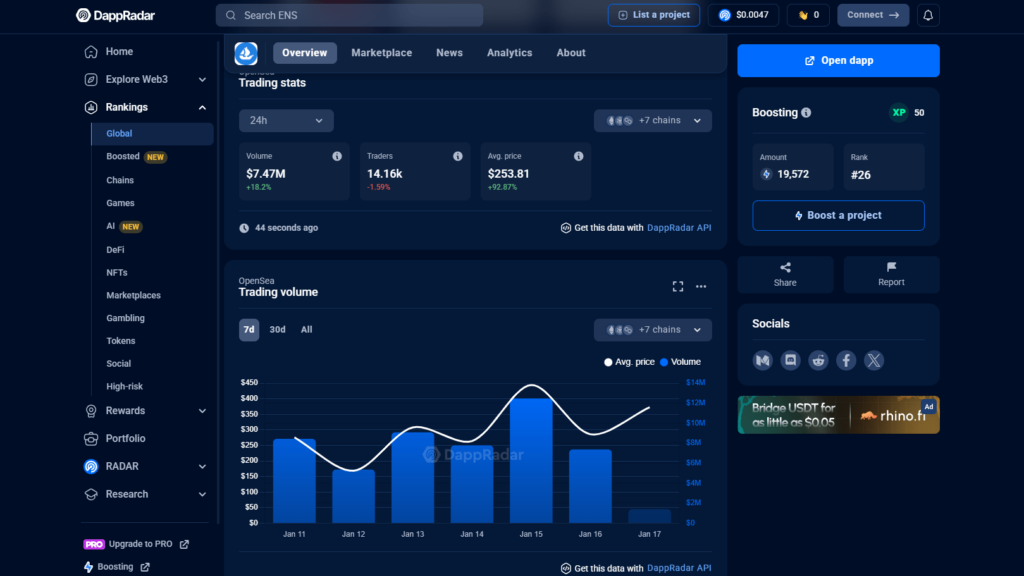
The NFT marketplace volume chart tracks trading activity across blockchains like Ethereum, Solana, and Polygon. High trading volumes often signal strong demand for NFTs, indicating where the action is in the underlying altcoin ecosystem. A rise in activity on alternative chains could mean they’re gaining adoption over Ethereum in specific niches. For example, Ethereum dominates the NFT market, but Solana and Polygon have gained traction due to lower fees and faster transactions, according to NFTScan.
6. DeFiLlama narratives tracker
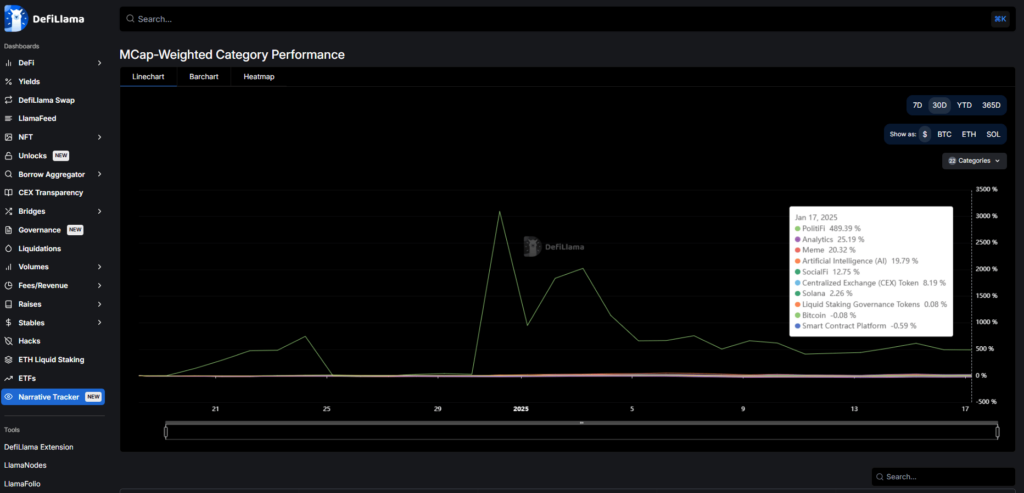
The DeFiLlama narratives tracker highlights trending themes and sectors in the cryptocurrency market, such as liquid staking, Layer 2 adoption, or real-world assets (RWAs). By aggregating data from DeFi protocols, this tool helps investors spot emerging narratives that drive market activity and influence altcoin performance.
For example, during the rise of liquid staking platforms like Lido, the tracker showed increased capital flow, signaling a key opportunity for altcoin growth. Traders can use this tool to align their strategies with market trends and identify early movers in the DeFi ecosystem.
7. Social media sentiment analysis
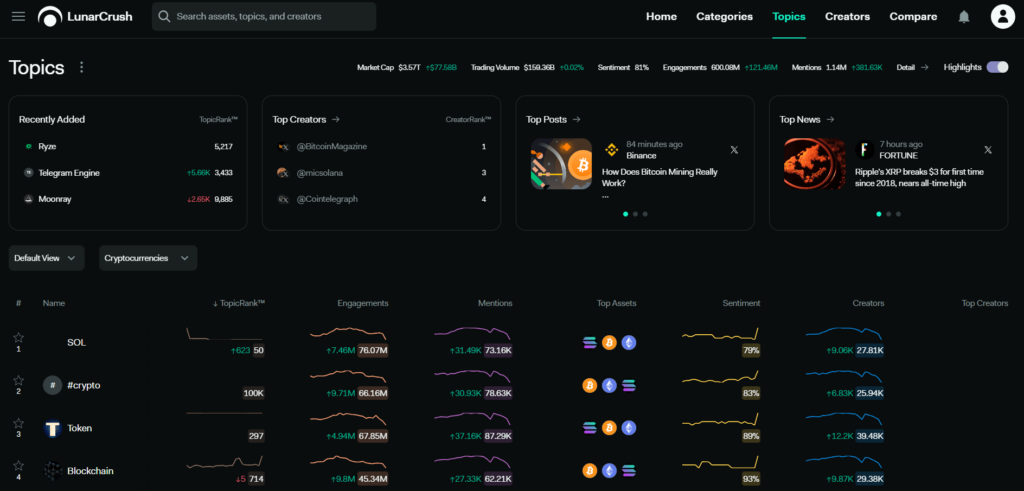
The social media sentiment analysis chart tracks discussions and emotions surrounding cryptocurrencies on platforms like Twitter, Reddit, and Telegram. Positive sentiment often indicates growing interest, while negative sentiment may signal caution or fear among traders.
For example, a surge in mentions of altcoins like Solana or Polygon during major announcements can reflect community excitement and potential price movements. Conversely, rising negative sentiment could foreshadow selling pressure.
This chart helps traders understand market mood, and identify when hype or skepticism could influence price trends. Analyzing sentiment alongside other metrics provides a broader view of market dynamics.
8. Wallet activity tracker
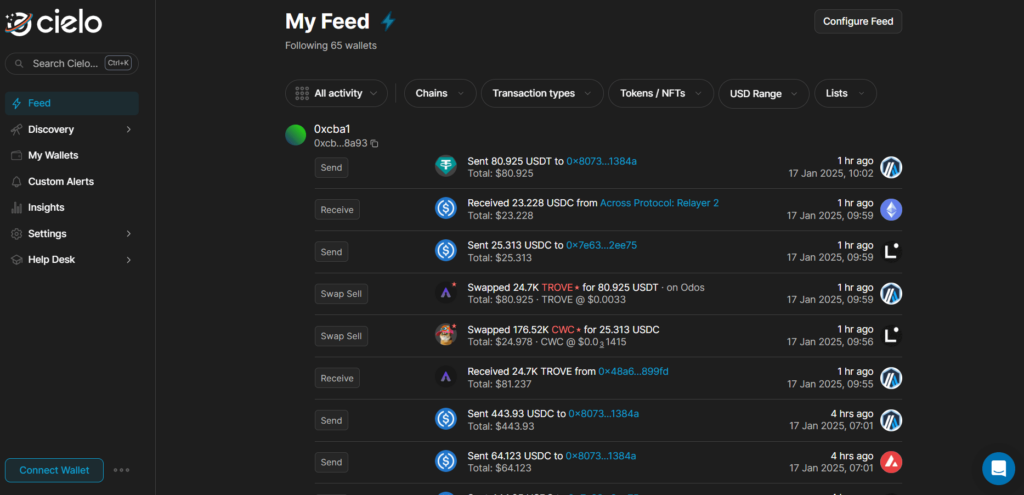
The wallet activity tracker monitors what’s going on in blockchain wallets. This could be things like large transactions, active addresses, and changes in wallet balances. An uptick in wallet activity often reflects growing interest or significant market moves. For instance, spikes in Ethereum wallet activity during DeFi booms indicated increasing participation in staking and trading.
Sudden large transfers from whale wallets to exchanges can signal potential sell-offs, while rising balances in non-custodial wallets suggest accumulation.
This tool helps investors track shifts in market dynamics, offering early signals for price changes.
9. Staking participation rate by altcoin
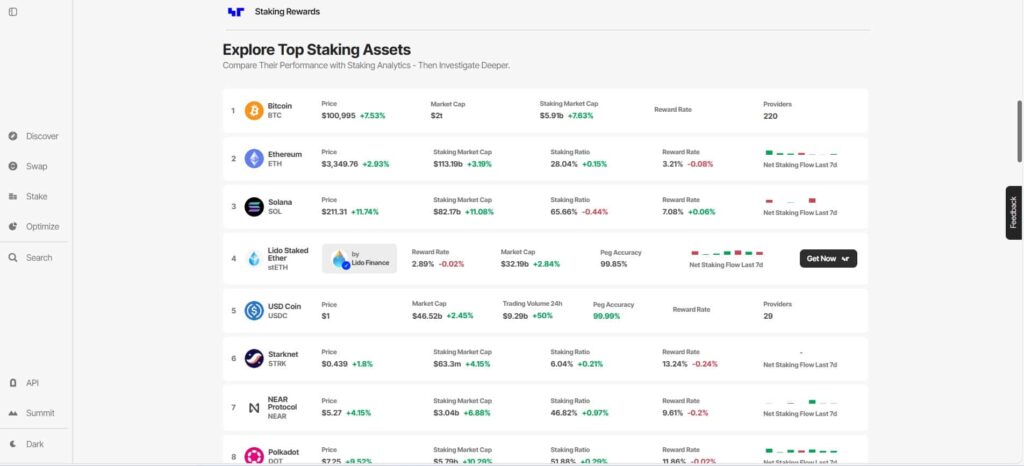
The staking participation rate measures the percentage of an altcoin’s total supply that is locked in staking. This can be viewed as a measure of long-term confidence in the network. Coins like Ethereum (ETH), Cardano (ADA), and Solana (SOL) rely on staking to secure their Proof-of-Stake (PoS) networks.
A higher staking rate indicates trust in the network’s future, because it means stakers are locking their assets to earn rewards, thereby supporting network stability. For example, Ethereum’s transition to PoS boosted its staking participation, reflecting optimism about its scalability and growth.
This metric is a critical indicator for investors evaluating the health and long-term potential of altcoin ecosystems.
10. Meme coin market cap chart
This CoinMarketCap chart helps investors understand speculative trends and gauge market phases where meme coins thrive.
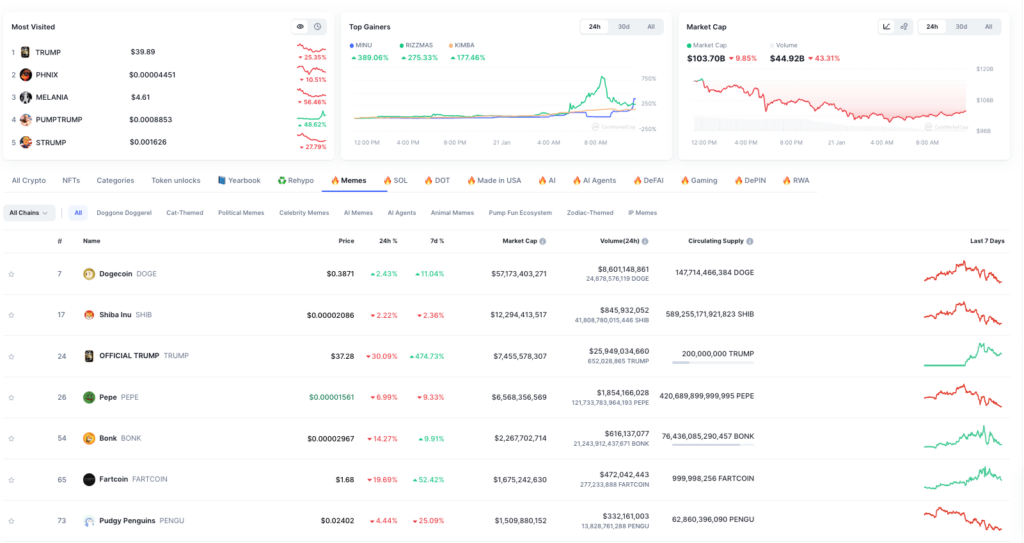
The chart of meme coin market cap tracks the total value of popular coins like Dogecoin (DOGE), Shiba Inu (SHIB), and Pepe (PEPE), as well as lesser-known ones. Meme coins often see spikes in market cap during bullish phases, fueled by community hype, social media trends, or celebrity endorsements.
For example, Dogecoin’s surge during Elon Musk’s tweets showcased how sentiment can drive speculative activity. Rising meme coin dominance reflects high-risk appetite among traders, while declines suggest a shift toward more serious assets.
Conclusion
There are a myriad of charts to steer you through the altcoin markets. These ten examples, along with the Top Ten Bitcoin charts in part 1, will help you keep your bearings in these stormy seas. Godspeed cryptonauts!
Let us know your thoughts! Sign up for a Mindplex account now, join our Telegram, or follow us on Twitter.
From VUCA to BANI: Futurist Jamais Cascio Helps Navigate the New Chaos
I’ve been talking with Jamais Cascio off and on for a couple of decades. About a month or so ago, he posted about completing a new book titled Navigating the Age of Chaos: a Sense-Making Guide to a BANI World That Doesn’t Make Sense.
Researching the latest from Jamais, I came across two tropes or memeplexes that I was unfamiliar with – VUCA and BANI. Jamais, who has long been a highly respected thinker and scenario builder in the world of futurists, had shifted much of the discourse from YUCA to BANI. From what to what?
VUCA = Volatility, Uncertainty, Complexity, Ambiguity
BANI = Brittle, Anxious, Nonlinear, Incomprehensible
I will steal a brief biographical note about Jamais from his website: “Selected by Foreign Policy magazine as one of their Top 100 Global Thinkers, Jamais Cascio writes about the intersection of emerging technologies, environmental dilemmas, and cultural transformation, specializing in the design and creation of plausible scenarios of the future.” I will let my email exchange with Jamais take it from here.
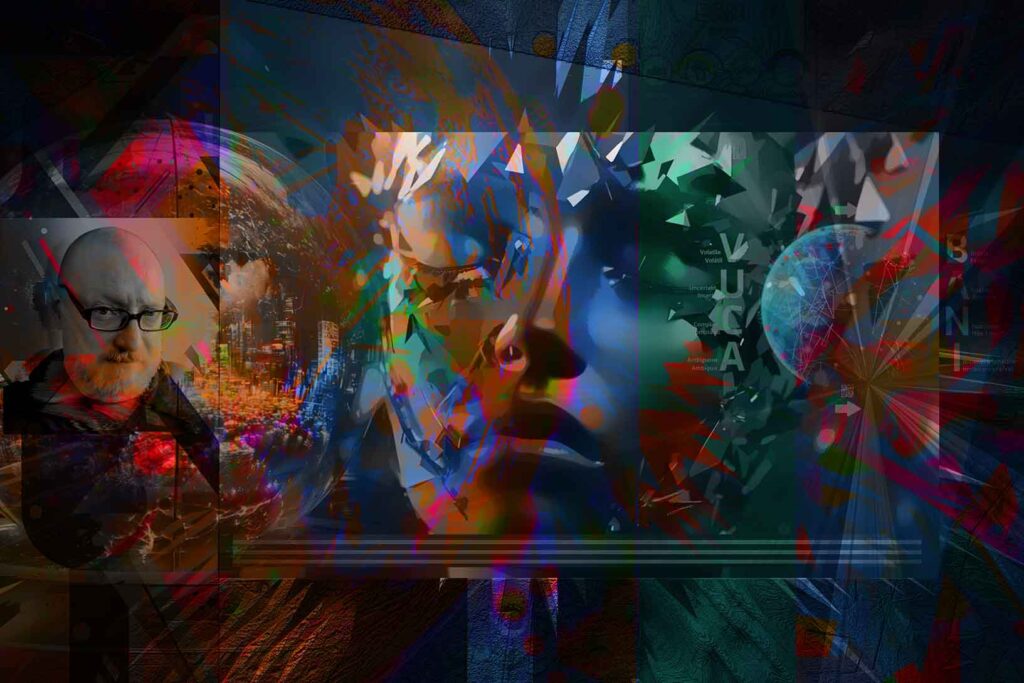
Let us know your thoughts! Sign up for a Mindplex account now, join our Telegram, or follow us on Twitter.
Could generative AI be on the right path to AGI?
Some time ago Ben Goertzel reported that somebody told him: “For the camp that continues to claim that throwing massive amounts of compute at LLMs isn’t a reasonable path to AGI… I don’t know if that’s accurate anymore.”
Ben then argued to confirm that no, throwing more and more computing power at large language models (LLMs) isn’t a reasonable path to artificial general intelligence (AGI).
The overall flavor of Ben’s arguments is captured by his analogy with music: music generation models trained on music up to the year 1900 “will not invent progressive jazz, neoclassical metal, grunge, acid house, etc. etc. etc.” I’ll come back to this analogy.
I’ve long been persuaded that Ben is right on this point. However, I’ll play devil’s advocate and try to argue that, yes, LLM-like big data crunching could be all (or almost all) of general intelligence.
Could statistical data crunching be almost enough?
I asked this question on social media, including the SingularityNET forums: Perhaps language is all there is? Or most of what there is? What I mean is that we could consider all forms of interacting with the rest of the world as a generalized form of language, and perhaps a generalized type of transformer technology with suitable training input would reproduce all aspects of cognition just like LLMs reproduce language.
Predictably, I received very skeptical replies. Come on, really now. How can language be all there is?
Ben conceded that everything is a language in some sense, but argued that real AGI will need a new framework of which today transformer-based LLMs could well be a part, but only a small one. Ben makes this point in his last book: “the basic architecture and algorithmics underlying ChatGPT and all other modern deep-NN systems is totally incapable of general intelligence at the human level or beyond, by its basic nature,” he says. “Such neural networks could form part of an AGI, sure – but not the whole cognitive part.”
But perhaps we shouldn’t dismiss the possibility that, yes, a generalized form of language could be all, or most, of what there is to AGI. The possibly at least merits further thoughts.
I’m not expressing a conviction, but a hypothesis. Current generative AI is not good enough for AGI. But what if it is almost good enough?
Let’s go for a drive
The example that comes to my mind is driving. When I’m driving, I use decades of experience with the ‘language’ of driving in the street. This language is composed by tokens like steer right/left, speed up, slow down etc. My experience tells me which token to ‘utter’ next, even without formal rules. All drivers know that in certain situations one should slow down, even if one is not able to say exactly why. It is your hands and your feet that know, so to speak. It seems to follow that a suitable ‘LLM’ trained with millions of hours of street videos of people driving – maybe call it an ‘LDM’, a Large Driving Model – could drive pretty well.
Could generative AI methods enable an AI, trained on a very large repository of videos of people driving in all sorts of different situations, to drive a car well enough? I think this hypothesis is worth exploring.
OK, maybe driving. But AGI? Come on.
Well, of course AGI will need other things as well, for example reasoning models for logical thinking, inference, mathematics and that sort of things. And some good old Bayesian reasoning of course. But I think of those subsystems as a very thin surface layer on top of a very thick bulk.
Vectors in the mindscape
If you ask me if there is a largest prime number, I’ll answer that no, there isn’t, and I’ll tell you exactly how I have reached that conclusion. But ask me why I like this particular woman at first sight instead of liking that other woman? The only honest answer I can give is that I don’t know (or care), but I still like her at first sight.
Do you still want an intellectual answer? Well, I guess there must be some vector that represents this woman in the bulk of my hugely multidimensional mindscape, and the tip of the vector comes close to some ideal woman.
But isn’t this a good description of what LLMs do?
So I’m entertaining the idea that some kind of LLM on steroids could be a good model for most of my mind. I claim to be generally intelligent, so it follows that some kind of LLM on steroids could achieve general intelligence. Of course the LLM should be complemented and enhanced by other things. But it would be the major part of a general intelligence, not a small one.
So I do agree with Ben, but with a difference in emphasis. He sees the LLM glass half empty, but I see it half full.
Perhaps I should have said that today’s early LLMs are on the right path to reproduce not general intelligence (the surface layer), but the more primitive intelligence (the bulk) that enables us all animals to stay alive in the cold, unforgiving universe out there. But I suspect that the gulf between the two is not that wide.
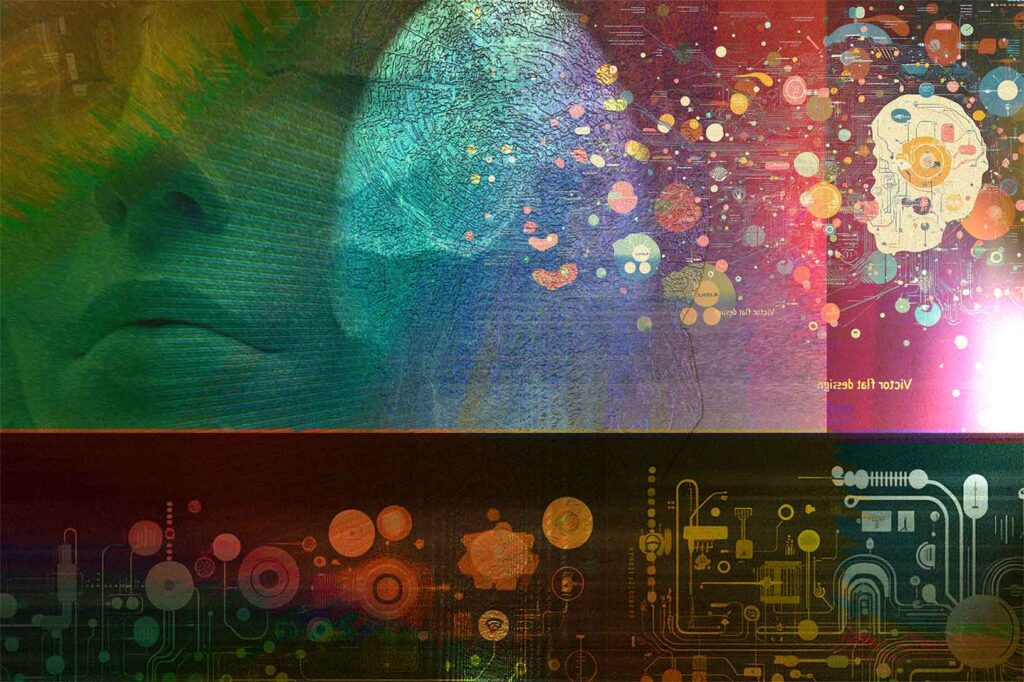
Active inference
There are interesting parallels and analogies between LLMs and a theory of sentient behavior called active inference, originated by Karl Friston and other scientists. The theory suggests that sentient life forms act upon their environment to build and continuously refine an internal model of the environment.
This is not limited to sentient life but rather is “something that all creatures and particles do, in virtue of their existence,” suggests Friston. The theory is based upon a “free energy principle” that has been proposed to unify information, thermodynamics, and biology. “For Friston, the free energy principle explains all features of living systems,” notes Anil Seth, and is “as close to a ‘theory of everything’ in biology as has yet been proposed.”
The analogies suggest that, perhaps, today’s early LLMs manifest the same universal forces that produced you and me.
And what about consciousness?
Thomas Nagel, in ‘What is it like to be a bat?‘, said conscious exists when it’s “like that” to be a certain being. In that sens, I am persuaded that it will be “like something to be an LLM” (say, a future one called GPT 10, or perhaps even GPT 7), even if it’s very different from our familiar experiences.
Back to progressive jazz
Let’s go back to Ben’s music analogy. Train generative AI on music up to the year 1900, and run it at low temperature. The AI will produce decent imitations of the music styles on which you trained it, though probably a bit aseptic and unimaginative. But now raise the temperature to the point where the AI produces music on the edge of chaotic noise, which only vaguely reminds of its training set. Most of that music will be unpleasant noise that nobody wants to hear. But now and then a high temperature run will produce something that some listeners will find at least interesting. Those samples will be publicized, discussed in music books, and included in new training sets. So there will be a gradual drift toward new styles, and perhaps we will get progressive jazz and all that.
I think this non-linear feedback will soon be evident in language and literature. Much of the text that we read online is already written by AI, and much more will come. AI started imitating people, but soon people will start imitating AI and picking up some new expressions produced by AI.
To be continued…
I’ve been writing this article for months. Of course there’s a lot more to be said, but I didn’t want to wait forever. So I’ll continue thinking and write a follow-up soon. In the meantime, there are always interesting discussions on X, like this one where Ben and I participated.
Let us know your thoughts! Sign up for a Mindplex account now, join our Telegram, or follow us on Twitter.