“I am the Law!” says Judge Dredd in the deeply satirical comics of the same name. Judge Dredd is a satirical pastiche of a law system gone wrong (despite Hollywood’s tendency to portray him as a hero in two excellent movies). It shows a world where far too much power is coagulated into a single figure who acts as judge, jury and executioner – a system of runaway justice which does as much to propagate the dystopian society in which it exists as it does to tame it.
We all know no one person should have that much power, that to err is human, and that in matters of law and order we need robust and sufficient oversight to iron out the blindspots of the individual human psyche. We have entire systems, necessarily bureaucratic and baroque, to ensure justice is served properly through our shared system of values, no matter how inefficient, arduous and expensive it may be. An inefficiency that, sadly, leads many to escape justice altogether, as overwhelmed police and law courts simply can’t keep up.
Painful Parables
Yet what about the use of AI to help us dispense justice? Recent proponents have argued that AI can help iron out human bias, process police work quicker, solve cold cases and perhaps even predict potential crime before it happens. They argue by drastically increasing the efficiency of police work, we increase its efficacy. It sounds great to some, utterly terrifying, to others – a short hop from an authoritarian nightmare.
In the Judge Dredd comics, Judges are supported by AI systems that help them determine what crimes have been committed. Minority Report, by Phillip K. Dick (also made into an outstanding movie), uses an AI system to process human visions to determine who is guilty by sheer predestination, locking them up before a crime has even occurred. In Psycho-pass, an exceptional cyberpunk anime, an AI system supervises human mental activity and distils it into a ‘Crime Coefficient’ which is then used to bring perps to ‘justice’ based on probability alone.
As readers and viewers, we abhor these insane AI-driven systems of justice, we see them as cautionary tales from impossible futures to teach us not what to do to build a better society. We may even dismiss them as silly sci-fi tales, parables that would never happen in our world.
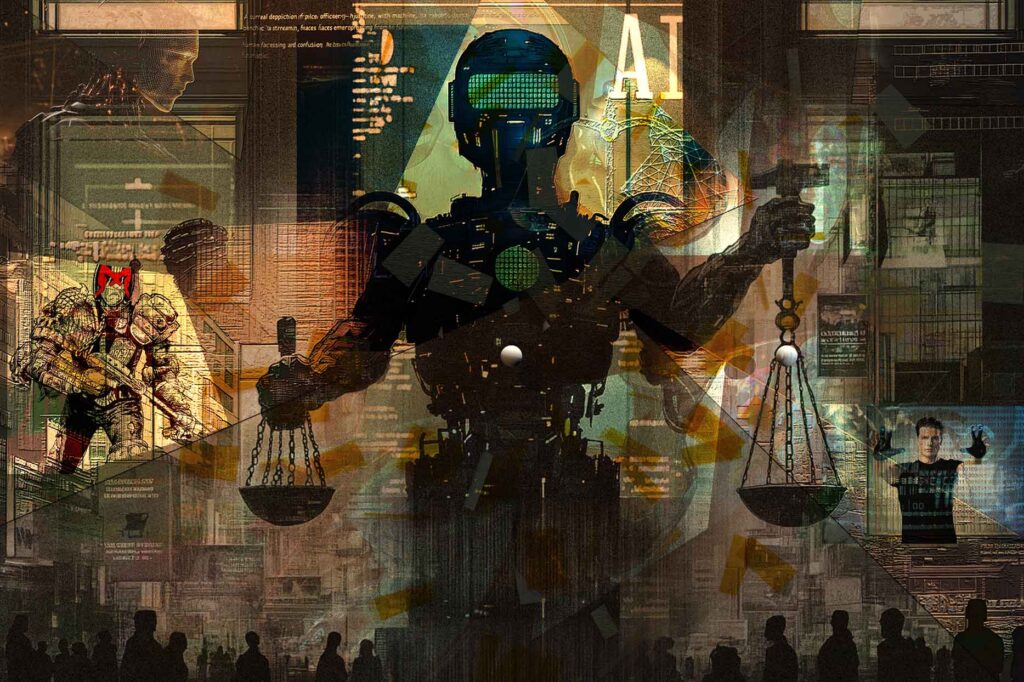
The Use of AI in Law Enforcement
Except, it’s starting to happen. It’s come with the appropriate insistence on frameworks and regulations, but AI is now beginning to be used by police forces to help them with their work. A tool that can do ‘81 years of police work in 30 hours’ is being trialled by UK police, helping them identify potential leads buried in mounds of evidence. AI is relentless, and its ability to sift through acres of documentation is its likely most compelling use-case to date. Putting it to work collating evidence from thousands of documents does seem like an efficient use of the system – but the implications do remain terrifying.
One example of this is seen in the use of AI to write police reports by US officers. That’s insane. In a world where criminal convictions can hang on literally one word in a statement, using a generative AI to create them based on the noted jottings of a police officer is throwing open the door to possible miscarriages of justice. There is a time and a place for AI, and in matters of justice where exact recollections matter, using an AI to write the document of record on events can’t be acceptable.
We still don’t know exactly how these LLMs arrive at their conclusions. AI researchers at the top companies can’t ‘work backwards’ through output, it doesn’t work like that. It’s a dangerously slippery slope to start using AI to generate the reports that are the foundation of much of our legal system. Studies show it barely saves time anyway, and issue with how these bots are trained means instead of eroding bias, they may fortify it.
Sentenced to Life
It won’t stop though. Implementations of AI initiatives in policing are already widespread in the UK. For work that is so process-driven and numbingly painstaking, the attractions of using AI to speed everything up is too alluring. The data which feed these AIs must be carefully chosen, for they will surely enshrine bias that has lived in police documentation for generations. The Met police has been accused of being institutionally sexist, racist and homophobic – you think an AI trained on their historical practices is going to be a paragon of egalitarian virtue?
The law works by slow degrees. Its cumbersome nature is an antidote to the disaster of false justice. Sci-fi about the horror of AI-driven police systems are important warnings of the perils of too many shortcuts. Like every aspect of society, there may well be a place for AI in helping keep our society safe, but we must tread very carefully indeed, for an exponential singularity in this sector could soon see all of us locked up for crimes we never knew we’d commit, on the reasoning of AI models we don’t truly understand.
Let us know your thoughts! Sign up for a Mindplex account now, join our Telegram, or follow us on Twitter.