Let us know your thoughts! Sign up for a Mindplex account now, join our Telegram, or follow us on Twitter.
Ten Key Crypto Technical Indicators for Beginners To Know in 2024
Note from the editor: This is not financial advice. The claim that technical indicators predict future price movements better than chance has not been validated by Mindplex.
The Bitcoin halving has come and gone, and crypto markets are in the doldrums of summer. Explosive growth has settled into a cruise, presenting the perfect opportunity to master an important component of crypto investment success: technical indicators, which are tools used to perform technical analysis (TA).
Are you new to the world of cryptocurrency trading and feeling overwhelmed by the sheer number of technical indicators available? Or you’re an inexperienced investor that just can’t figure out what’s going to happen next? That’s because the crypto market is dominated by short-term trading, mostly with bot trading tools, that look at specific indicators to devise an optimal strategy. Therefore, while you don’t have to be a pro trader, you should at least know the rules of the trading game before you invest in shorter time frames.
In this article, I’ll cover ten important crypto trading indicators that any crypto trader can try and master in 2024 to manage their Bitcoin and Ethereum. These indicators (which I’ll explain in descending order of importance) will help you make more informed trading decisions in the dynamic crypto market.
It’s important to note that no single indicator should be viewed in isolation. Instead, traders should use a combination of indicators to confirm signals and make more informed decisions. By using multiple indicators together, you can gain a more comprehensive understanding of market trends, potential entry and exit points, and overall market sentiment.
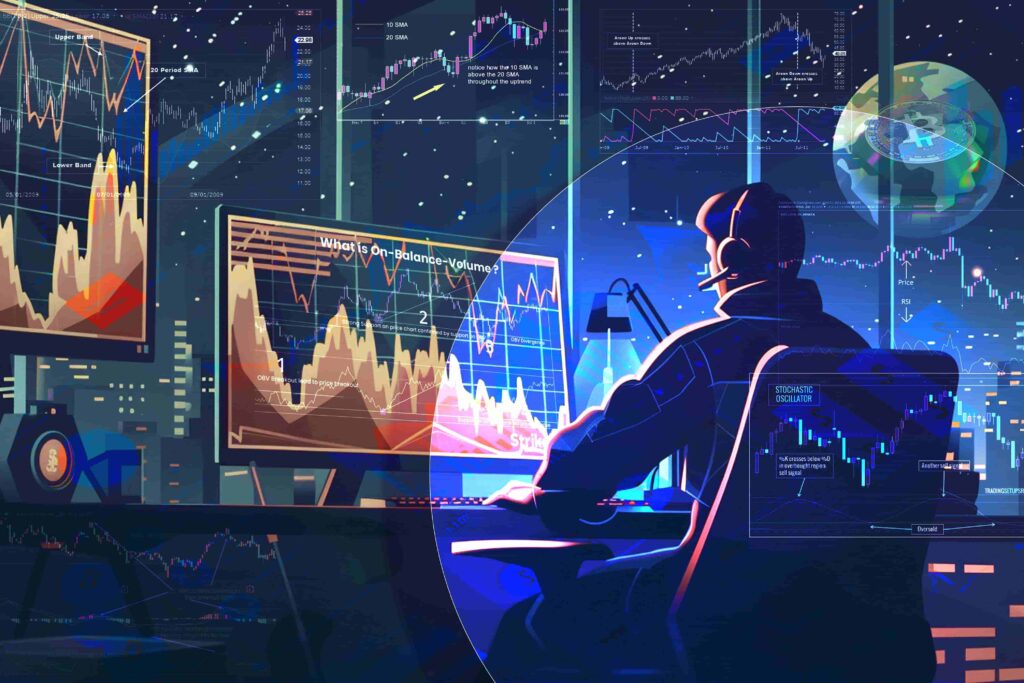
1. Moving Averages
What is a Moving Average?
A moving average is a technical analysis tool that smooths out price data by creating a constantly updated average price over a specific period.
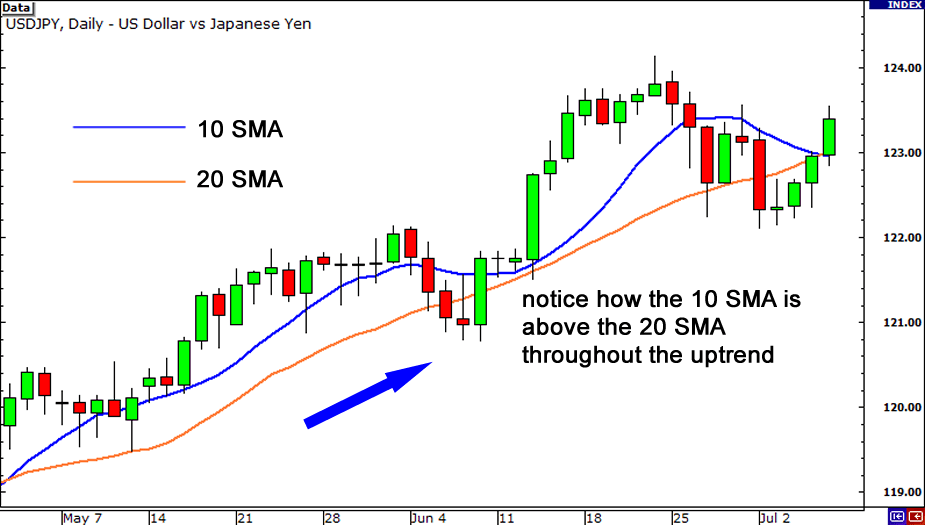
What does it do?
Moving averages help identify trends and potential support and resistance levels. They can also serve as a foundation for other technical indicators.
How it works
The two most common moving averages are the simple moving average (SMA) and the exponential moving average (EMA). An SMA is calculated by taking the average price over a set number of periods – while an EMA gives more weight to recent prices.
2. Relative Strength Index (RSI)
What is a RSI?
The Relative Strength Index (RSI) is an unmissable momentum indicator that measures the speed and magnitude of price changes. Its stochastic RSI variant is said to provide an easy visual way to see whether prices are bottoming or topping.
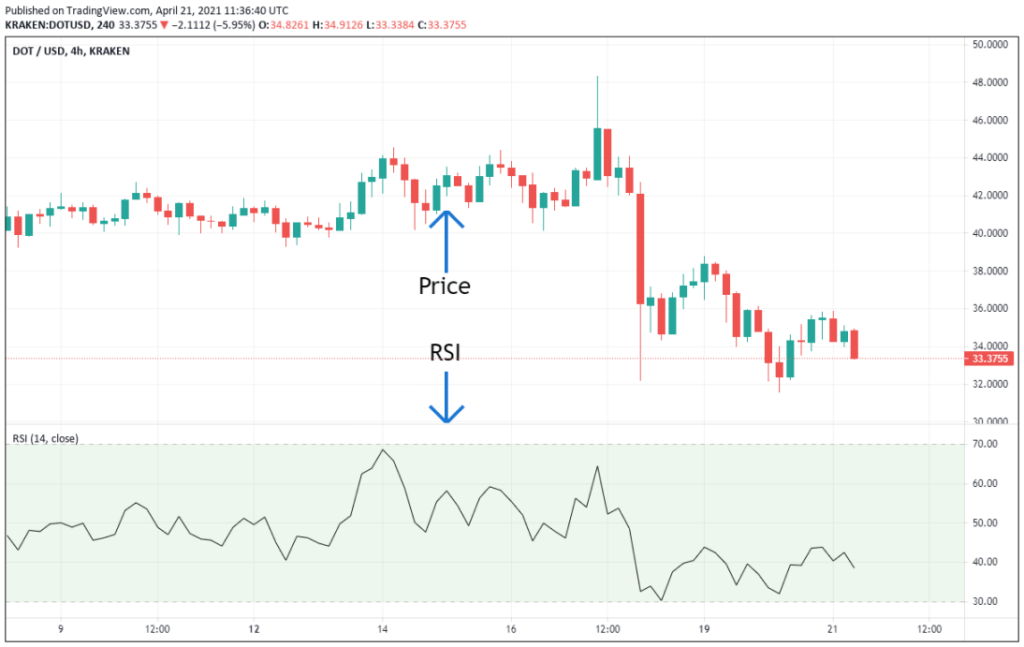
What does it do?
RSI helps identify overbought and oversold conditions, which can signal potential reversals or trend confirmations.
How it works
RSI oscillates between 0 and 100. Readings above 70 suggest an overbought condition, while readings below 30 indicate an oversold condition.
3. Bollinger Bands
What are Bollinger Bands?
Bollinger Bands are volatility indicators that consist of a middle band (typically a 20-day SMA), plus two outer bands set two Standard Deviations above and below that middle band.
What does it do?
Bollinger Bands help identify potential overbought and oversold conditions, as well as potential breakouts when the price moves outside the bands.
How it works
When the price touches the upper band, it’s considered overbought, and when it touches the lower band, it’s considered oversold. A squeeze in the bands often precedes a breakout.
4. Fibonacci Retracement
What is a Fibonacci Retracement?
Degen traders’ favorite tool of choice, Fibonacci retracement levels are based on the controversial Fibonacci sequence and are used to identify potential support and resistance levels.
What does it do?
Fibonacci retracement levels allegedly help traders set price targets and determine entry and exit points.
How it works
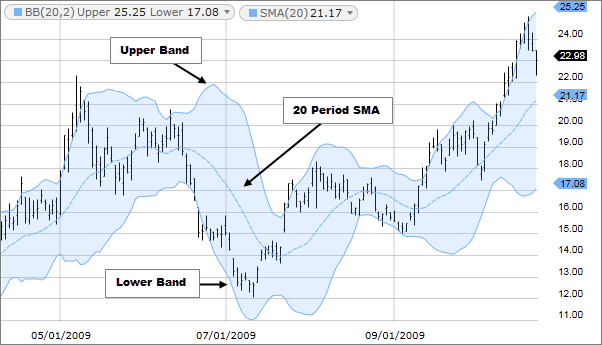
The most commonly used Fibonacci retracement levels are 23.6%, 38.2%, 50%, 61.8%, and 78.6%. These levels are plotted on a chart by drawing a line from a swing high to a swing low (or vice versa) and then dividing the vertical distance by the key Fibonacci ratios.
5. On-Balance-Volume (OBV)
What is an OBV?
On-Balance-Volume (OBV) is a volume-based indicator that helps confirm price trends and predict potential reversals.
What does it do?
OBV can help identify divergences between price and volume, which can signal a potential trend reversal.
How it works

OBV adds or subtracts volume based on whether the price closes higher or lower than the previous day. If OBV is rising while the price is flat or declining, technical analysts claim that suggests a potential price increase, and vice versa.
6. Moving Average Convergence Divergence (MACD)
What is a MACD?
The Moving Average Convergence Divergence (MACD) is a trend-following momentum indicator that shows the relationship between two moving averages.
What does it do?
MACD helps identify trend changes, momentum, and potential buy and sell signals.
How it works
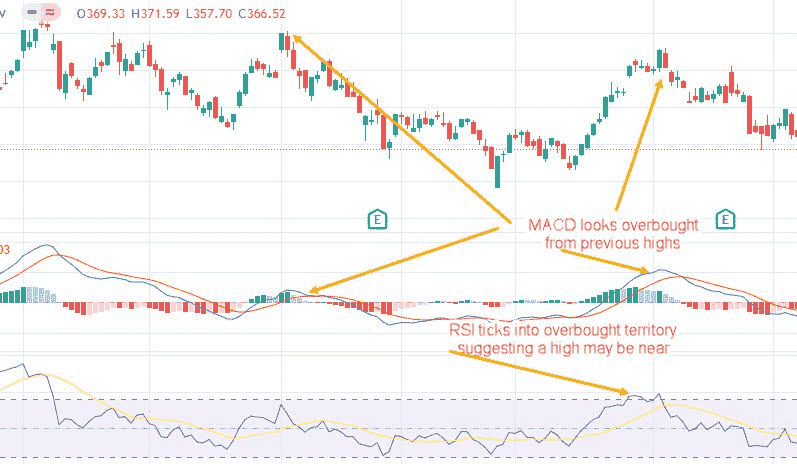
MACD consists of a MACD line (the 12-day EMA minus the 26-day EMA) and a signal line (a 9-day EMA of the MACD line). When the MACD line crosses above the signal line, it’s a bullish signal, and when it crosses below, it’s a bearish signal.
7. Stochastic Oscillator
What is a Stochastic Oscillator?
The Stochastic Oscillator is a momentum indicator that compares the closing price of an asset to its price range over a specific period.
What does it do?
The Stochastic Oscillator helps identify overbought and oversold conditions and potential reversals.
How it works

The indicator consists of two lines: %K and %D. When %K crosses above %D, it’s a bullish signal, and when it crosses below, it’s a bearish signal. Readings above 80 suggest an overbought condition, while readings below 20 indicate an oversold condition.
8. Ichimoku Cloud
What is an Ichimoku Cloud?
The Ichimoku Cloud is a comprehensive indicator that provides a quick overview of an asset’s price action, trend direction, and potential support and resistance levels.
What does it do?
The Ichimoku Cloud helps traders identify the prevailing trend, gauge momentum, and spot potential buy and sell signals.
How it works
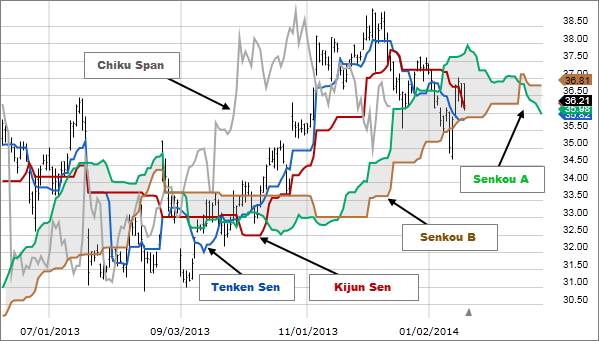
The Ichimoku Cloud consists of five lines: the Tenkan-sen, Kijun-sen, Senkou Span A, Senkou Span B, and the Chikou Span. When the price is above the cloud, it’s considered bullish, and when it’s below the cloud, it’s considered bearish.
9. Aroon Indicator
What is the Aroon Indicator?
The Aroon Indicator is designed to identify trend changes and measure the strength of a trend.
What does it do?
The Aroon Indicator can help traders spot trend changes and potential entry and exit points.
How it works
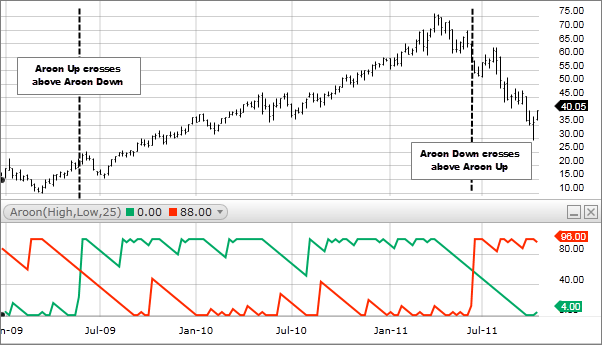
The indicator consists of two lines: the Aroon Up and the Aroon Down. When the Aroon Up is above the Aroon Down, it indicates an uptrend, and when the Aroon Down is above the Aroon Up, it indicates a downtrend.
10. On-Chain Metrics
What are On-Chain Metrics?
On-chain metrics provide valuable insights into the fundamental health and activity of a cryptocurrency network. Looking at data such as total market cap, circulating supply, fully diluted value, total value locked, dApp activity, transaction count, user activity and more can help you see through all the smoke and mirrors in a bull market.
What do they do?
By monitoring on-chain metrics, traders can gauge the overall sentiment and growth potential of a particular cryptocurrency.
How it works
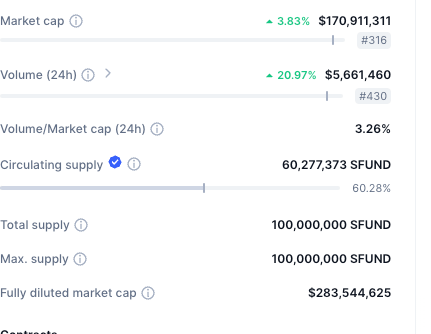
Some important on-chain metrics include transaction volume, active addresses, network value to transaction ratio (NVT), and realized cap. These metrics can be tracked using various blockchain explorers and analytics platforms.
Conclusion
In conclusion, these ten essential crypto technical indicators provide a powerful foundation for beginner traders in 2024, but it’s good to keep in mind that it barely scratches the surface of what’s possible in technical analysis.
You can test out applying these indicators for yourself, and see if they help you make more informed trading decisions or improve your chances of success in the dynamic crypto market.
However, it’s crucial to remember that no single indicator is perfect, and relying on just one can lead to suboptimal results. Maybe using a combination of indicators to confirm signals will help you gain a more comprehensive understanding of market trends and sentiment.
As you continue your trading journey, stay committed to continuous learning and adapting to the ever-changing crypto landscape. With dedication and practice, you’ll be well on your way to becoming a proficient trader in the exciting world of cryptocurrencies.
Let us know your thoughts! Sign up for a Mindplex account now, join our Telegram, or follow us on Twitter.
W’ETH Done It! Ethereum Spot ETF Approval Opens World to Web3
Introduction
While the traditional crypto wisdom is to sell in May and go away, Ethereum had other plans. On 23 May 2024, the U.S. Securities and Exchange Commission (SEC) surprisingly greenlit spot Ethereum (ETH) exchange-traded funds (ETFs) in principle. This has sent its price skyrocketing and turned its future very bright. Most crypto investors thought this would never come, due to the United States’ onslaught on cryptocurrency technology, or would require a multi-year political struggle.
It marked a pivotal and triumphant moment for the cryptocurrency market that will boost its development even further and bolster a beleaguered US crypto sector dragged down by heavy-handed regulation the last few years. It also again showed that politics and finance always intertwine, coming soon after Donald Trump’s embrace of crypto in the US essentially forced that country’s Democrat party to make a dramatic U-turn on the sector in what will be a tough election year to keep Joe Biden in power.
In this article, we’ll explore the implications of this approval, the impact its had on Ethereum and the broader crypto market, and the potential benefits for retail investors.
What is an Ethereum ETF?
An Ethereum ETF is a financial product that allows investors to buy shares representing a stake in Ethereum, without needing to directly purchase or manage the cryptocurrency itself. ETFs are traded on traditional stock exchanges, making them accessible to a wider range of investors. The approval of these ETFs means institutional and retail investors can now gain exposure to Ethereum through regulated financial products.
The Road to Approval
The journey to the approval of Ethereum ETFs has been long and difficult, and fraught with regulatory hurdles since Vitalik Buterin – with co-founders including Cardano’s Charles Hoskinson and Polkadot’s Gavin Wood – launched Ethereum in 2015 as a novel application of blockchain technology.
The SEC’s approval of Bitcoin ETFs earlier in the year laid a path down for Ethereum ETFs to follow. This path was studded with rigorous analysis and a public comment period, during which stakeholders provided feedback on various aspects of the proposed ETFs, including custodianship, sponsor fees, and creation and redemption models.
Market Reactions and Expectations
Impact on Ethereum’s Price
A tweet from influential analysts Eric Balchunas and James Seyffart increased the estimated odds of an Ethereum ETF to 75%. The result: the price of ETH spiked within hours to nearly $4,000, before retracing.
Following the announcement, the price of Ethereum remained largely unchanged, soon dropping to as low as $3,500 in typical “sell the news” behavior. A number of other factors also impacted the ETH price blowoff. Notably, additional hurdles must be cleared first before an actual Ethereum ETF is approved, such as the final filing of amended S1 applications for each issuer (BlackRock is pushing for a July 4th launch) as well as another round of Mt Gox FUD. And of course, there’s also the question of whether we’ll see another huge sell-off from Grayscale holders.
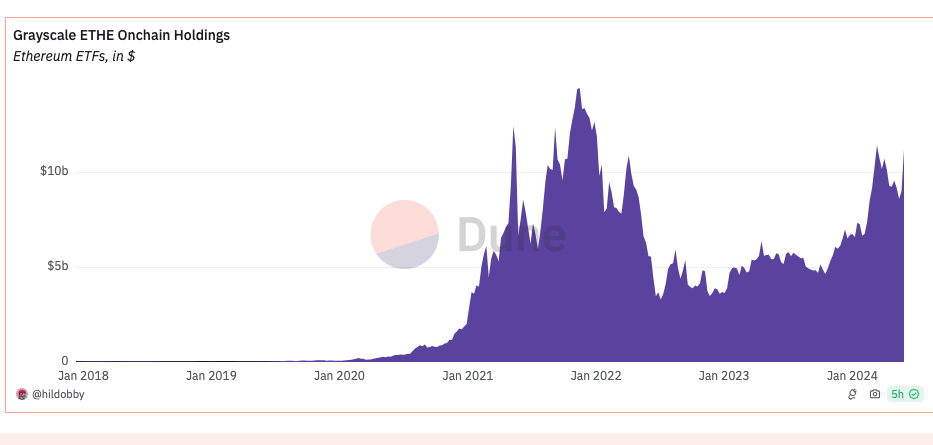
This price movement is reminiscent of the initial reactions seen with Bitcoin ETFs, suggesting a pattern of bullish sentiment following regulatory endorsements. However, investors had more time to ladder into their BTC buys, knowing that it was almost certainly coming, whereas Ethereum went into the ETF battle as an underdog.
What does an Ethereum ETF mean longterm for crypto?
Regulatory Greenlight
The SEC’s decision brings Ethereum closer to being classified as a commodity, like Bitcoin. The devastation of being labeled a security has been hanging like a sword over its head for years, despite earlier statements by both the SEC’s William Hinson in 2018 and the CFTC in 2022 that it was not a security. Classification as a commodity will simplify regulation for Ethereum, providing clearer guidelines for future developments and investments in the ecosystem. Additionally, the recent crypto-friendly legislative movements in Congress have created a more supportive environment for digital assets.
This regulatory clarity helps to clear the way for the network to scale and become the world’s computer. Ethereum’s ongoing technological upgrades – such as the implementation of zero knowledge roll-up technology and sharding – are poised to increase the network’s transaction capacity and efficiency which will help maintain Ethereum’s competitiveness against rivals such as Solana, Sui and Cardano and support its growing ecosystem of layer-2 networks such as Arbitrum, Optimism, Base, Linea, Stark and ZkSync. On all these networks in turn reside hundreds of thousands of decentralized applications (dApps).
Web3 Stays Free
Classifying Ethereum as a security would significantly impact the Web3 industry, affecting dApps, DeFi, and NFTs that rely on Ethereum’s layer-2 solutions. With Ethereum leading the DeFi space, holding over 60% market share and $100 billion in total value locked, regulatory challenges could arise that could kill any other crypto layer-1 and layer-2 network, especially proof-of-stake ones.
Benefits for Retail Investors
One of the primary benefits of Ethereum ETFs for retail investors is the ease of access. Investing in ETFs does not require the digital know-how or cybersecurity measures needed for direct cryptocurrency investments. There’s no private key management, no hacking and scam risks, which cost crypto investors billions each year. This accessibility can encourage more widespread participation in the crypto market.
ETFs offer a diversified investment option: they can include a range of assets within a single product. For retail investors, this means a more balanced exposure to Ethereum, potentially reducing the volatility risks of individual cryptocurrency investments, and also means insured investments.
On the downside though, ETF investors will not earn any ETH staking rewards yield (at least not initially). This reward is around 5%. Ethereum’s Shanghai upgrade last year kicked off a liquid staking (LST) and Eigenlayer restaking frenzy that has rejuvenated its struggling DeFi sector.

Trading Strategies Around Ethereum ETFs
With the approval of several Ethereum ETFs now just over the horizon, traders can adopt various strategies to navigate the evolving market landscape. We won’t speculate on the future price of Ethereum, as there are too many variables at play, both on an industry and macro-economic level. However, market sentiment is quite bullish for the next year, especially if the bull market resumes and ETH can turn deflationary again thanks to its EIP-1559 upgrade.
Conclusion
The approval of Ethereum ETFs is a transformative event for the cryptocurrency market that could unlock a new era of mainstream crypto adoption. This regulatory milestone validates Ethereum’s role in the financial ecosystem, and opens the door for increased institutional investment and greater market liquidity. It also boosts the development of Web3 technology and infrastructure, particularly in the USA.
For retail investors, Ethereum ETFs offer a convenient and regulated way to participate in the growth of the crypto market, providing opportunities for diversification and risk management. As Ethereum continues to innovate and evolve, ETFs will shape its future trajectory and solidify its position as a leading digital asset.
Let us know your thoughts! Sign up for a Mindplex account now, join our Telegram, or follow us on Twitter.
Cyberpunk Legend RU Sirius | Mindplex Podcast S2EP20
Bittensor (TAO): The Crypto Neural Network for AI and ML Model Development
As we never tire of saying at Mindplex and SingularityNET, progress in developing advanced AI and ML models is tainted by the chances of centralized institutions gaining undue control over one of the most important technological evolutions in human history.
The race is afoot: key players in AI are under the thumb of institutions, even if they started off as non-profit and open-source projects. Decentralized AI proposes free-roaming AIs to combat fortress-style AI solutions; it uses protocols and facilities built on the blockchain to promote multi-point control systems for AI infrastructures.
Bittensor, a decentralized AI project with a native cryptocurrency called TAO, has recorded exponential growth in 2024, growing an ecosystem of applications on its network, and gathering a community of contributors advancing the AI revolution.
Let’s discuss the key elements of Bittensor, and how they work in synergy to create a distributed network for the development of AI and ML models and algorithms.
What is Bittensor?
Bittensor is a decentralized network designed for peer-based commodity development and provisioning of computing resources.
Bittensor is basically a marketplace for compute resources (storage space etc.), with a collaborative network for developing AI and Machine Learning models. Bittensor embraces competition as a strategy to ensure that commodities, resources, and models developed on its network are top-notch. It is a mesh consisting of computers performing different roles and collectively controlling a distributed network.
The Bittensor network has a more pronounced role in AI and ML model development. However, it is capable of doing even more. It is a modular network, built for flexibility. Key concepts of the network can be applied to many sectors. Other digital commodities that can be built using the Bittensor network include financial market prediction tools, marketplaces for computing power, and algorithms for protein folding experiments. Bittensor is a multi-purpose decentralized network. Requests are posted to a ‘request and solution’ network with advanced data intelligence for the development of AI tools. The Bittensor network is an enterprise-grade tool, opening doors to industry-level adoption for getting. It is also stable for micro implementations by individuals and small organizations.

The Bittensor network consists of harmonized subnets designed to share data on AI and ML models and provide digital resources. The key role players on the Bittensor network are –
- The Bittensor Subnets
- The Bittensor API
- Bittensor blockchain (Known as a subtensor) and
- The Yuma Consensus.
Understanding Bittensor subnets
Bittensor subnets are neural networks of advanced knowledge and data-sharing nodes. Nodes on the network are called ‘Neurons’, and can be Miner or Validator nodes. Validators are the input layer of the network: they create a communication portal between the external environment and the rest of the network.
First, Validators communicate with the hidden layer (miners) via the Synapse module, feeding them information on the task(s) to be done. Next, Miners compete on who provides the best answer or results for the task. Then, Validators assess the results provided by the Miners and feed the rest of the network with their evaluations. Miners are only in communication with the validators, hence, isolated from the rest of the network. There are about 36 subnets on the Bittensor network at the time of writing.
The Bittensor API connects the Subnets to the subtensor. Validators’ evaluations are processed as separate inputs and transported through the API to the Subtensor for consensus.
Understanding Bittensor subtensors
The subtensor is the final validation layer for results from the subnets. The Subtensor is a blockchain operating the Yuma Consensus. The Yuma consensus uses a weighted algorithm to compute validator evaluations and decide which model (developed by subnet miners) is best. The network rewards miners with TAO tokens as specified by the subnet’s incentive mechanism. Validators are also rewarded for their input.
Bittensor operates two subtensors, the Nakamoto and the Kusanagi blockchain. Each of these subtensors can perform final validations.
The Bittensor network is a supernet, housing several subnets created to solve different tasks. Subnets operate a network of validators who feed miners with information on the tasks to be done and assess their results for quality. Validators mediate between subnets, the external environment, and the subtensor to ensure a steady flow of information on the network. This way, Bittensor creates a network where participants can trustlessly share intelligence.
Making a case for the Bittensor network
The Bittensor is, first, an attempt to lower the barrier to developing AI Models. Bittensor caters for resource-intensive digital ventures. Through a collaborative network, it splits the financial burden across various participants, making it cheaper to develop digital facilities.
The network uses input from several network contributors and a decentralized assessment system to promote more efficient models and solutions. Unlike the siloed systems used by centralized organizations, the collaborative environment makes for more efficient products.
But even more important is trying to promote decentralization in developing and managing these tools. Blockchain technology gives Bittensor a multiple-point-of-control system that easily resists censorship attempts and taps the power of the community.
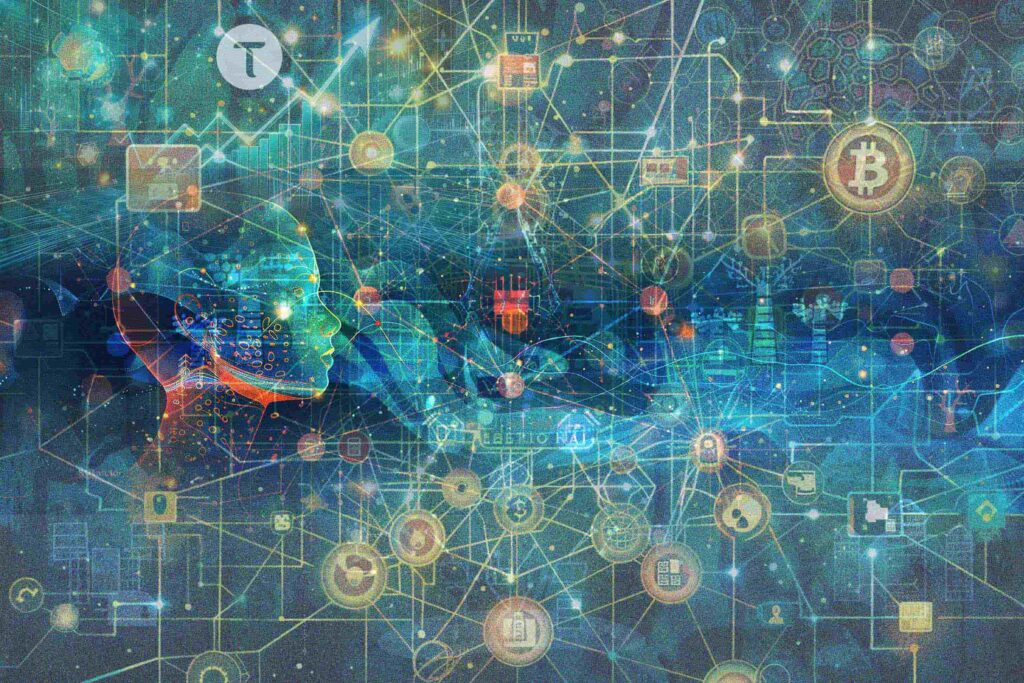
Bittensor (TAO) tokenomics
TAO is the Bittensor Network utility token. With TAO, Bittensor is creating an economy to keep the network in operation, promote adoption, and oversee its security.
TAO use cases
Incentivization: TAO is used to reward miners and validators on the network for their contributions to providing computing resources and developing AI and ML models. Subnet owners define the reward system for their subnets. Miners whose solution emerges the best are rewarded with TAO according to the subnet’s incentive mechanism. Validators also receive TAO rewards.
Network consensus: To create a value layer for the network’s security facility, validators stake TAO to their hotkeys to run a node on the network. Other TAO holders can also delegate their assets to their desired validator. The Validator stake is a commitment to the network.
Governance: TAO holders decide on project improvement through community voting. Proposed additions to the projects must be approved by a majority of the community before they are implemented.
TAO distribution
- Total TAO supply is 21 million coins, just like with Bitcoin.
- TAO block rewards also halve every four years
- New TAO tokens are generated through mining and validating
- One TAO is generated per block.
- The circulating TAO supply is about 6.8 million.
- TAO can be traded on centralized exchanges like Binance and Kucoin.
Who uses Bittensor?
Anyone can use the Bittensor network as a client or a participant in the network. Enterprises and individuals who wish to create a task on the network can set up their own subnet and define their incentive mechanism based on the TAO economy. Anyone can also plug their facility into the network as a Miner to help problem-solving, or as a Validator to contribute to assessing solutions. The incentive system ensures participants always have a point of attraction, keeping the network operational.
Conclusion
Bittensor’s neural network builds a base for developing decentralized intelligence systems, and a marketplace of AI solutions. The economy built on the TAO token ensures that this network stays in charge of the finance that fuels it. Advanced AI facilities are delicate and almost unlimited in ability.
A centralized administration is a bottleneck for developing and using AI. As a network that supports the development of AI models, Bittensor promotes the development of battle-tested models powered by a censorship-resistant system.
Let us know your thoughts! Sign up for a Mindplex account now, join our Telegram, or follow us on Twitter.
Wavefunction Matching: A Breakthrough in Quantum Calculation Accuracy
Introduction
Picture a world where we can peer into the tiniest particles that make up our universe, understanding their behaviors and interactions with stunning precision. This is the realm of quantum physics, a field that delves into the mysteries of protons, neutrons, and other minute particles known as “quantum many-body systems.” To unlock these secrets, scientists prefer to use an approach called “ab initio,” which means starting from the most basic principles and using them to derive the behavior of the system. Within this approach, the Quantum Monte Carlo Simulation plays a crucial role, using randomness to help make sense of complex calculations, via simulating a large number of possible ways a system may evolve and probabilistically combining the results. However, in many applications this technique has faced a major technical challenge: the “sign problem,”
This issue arises when positive and negative results offset each other, leading to erroneous conclusions. To tackle this challenge, researchers devised a solution known as “Wavefunction matching.” Imagine a complex puzzle simplified into a more manageable form—this is akin to what Wavefunction matching accomplishes. It involves mapping the intricate problem onto a simpler model devoid of oscillations, and any discrepancies are resolved using perturbation theory. By streamlining the problem and addressing differences, accurate calculations become feasible.

Applying this technique, researchers successfully computed the masses and radii of all nuclei up to a mass number of 50, aligning closely with real-world measurements. Furthermore, the utility of Wavefunction matching extends beyond rectifying Monte Carlo Simulations—it holds promise in the realm of quantum computing. By enhancing calculation accuracy and reliability, it serves as a valuable tool for understanding how nature actually works and applying those insights to problems in chemistry, physics, and quantum computing.
As Dr. Meißner suggests, this method’s versatility spans both classical and quantum computing domains, ushering in a new era of precision and reliability in calculations. This advancement isn’t just confined to rectifying existing methodologies; it holds the potential to revolutionize quantum algorithms, paving the way for swifter computations and superior outcomes across various quantum applications.
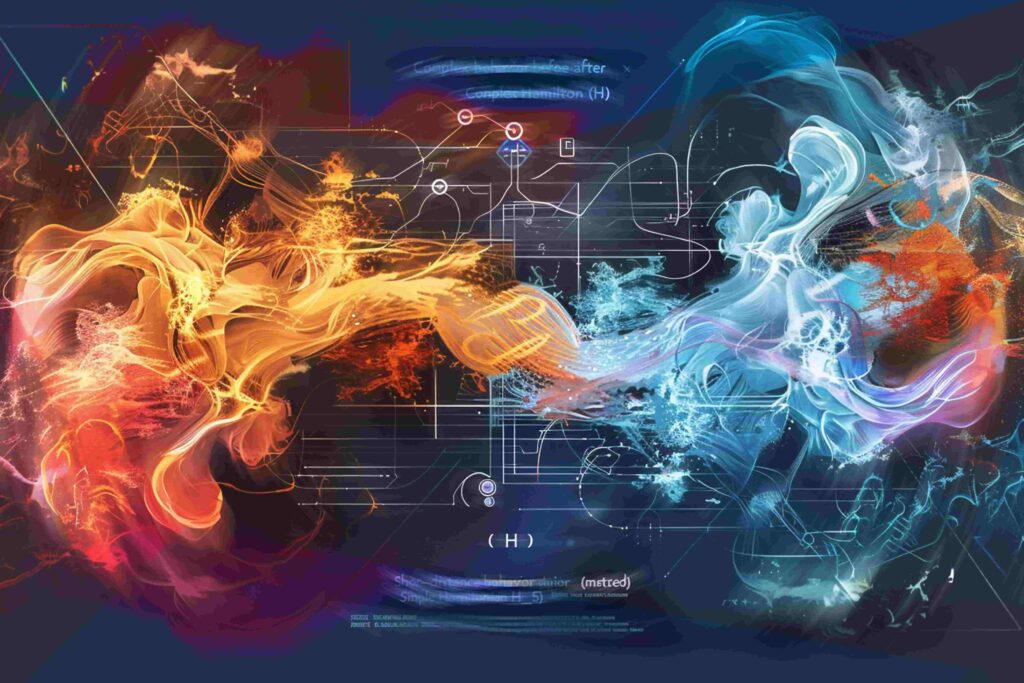
Conclusion
Richard Feynman said, ‘Any field for which there is a prize that is defined is a field that already has its best days behind it. It’s a field that barely has a name and is going to have the most fertile moment.’ Wavefunction matching is a good example of such a fertile moment in a research area still in its most exciting, early, innovative phase.
Let us know your thoughts! Sign up for a Mindplex account now, join our Telegram, or follow us on Twitter.
Will Congress Ban Telegram Next? | Highlights from S2EP18
Will the Crypto Vote Decide The 2024 Presidential Race?
Introduction
As the 2024 US presidential election approaches, a surprising issue has emerged: cryptocurrency. While once considered a fringe topic, the growing size and influence of the crypto voting bloc has forced candidates to take notice. As the Biden administration faces backlash over its regulatory crackdown, presidential rivals like Donald Trump and Robert F. Kennedy are seizing the opportunity to court disaffected digital asset enthusiasts.
The Rise of the Crypto Constituency
Let’s talk numbers. According to recent Paradigm and DCG Harris polls, a whopping 20% of Americans now own some form of cryptocurrency. That’s about 60 million people! And in key swing states, 1 in 5 voters say crypto is a big deal for them when it comes to picking a candidate. Over 11 million voters hold over $1000 in crypto assets.
If in the region of 10 million of voters say they care about crypto, and elections often swing on a hundred thousand votes or so, that’s a big deal. Crypto isn’t just popular with the tech bros anymore. It’s gaining serious traction with younger voters, college students, and ethnic minorities.
The Generational and Demographic Divide
Crypto’s political clout is particularly pronounced among younger and minority voters.
- 33% of African Americans and Hispanics own, trade, or use crypto (Paradigm)
- 2 in 5 men aged 18-54 and 1 in 4 college students own crypto (Paradigm)
- 70% of those polled are dissatisfied with the current financial system (DCG Harris)
These demographics, which have traditionally leaned Democratic, are now up for grabs. If the Biden administration takes a hard line on crypto regulation, it risks alienating this critical bloc.
Biden’s Crypto Crackdown Backfires
So, what’s the current administration’s take on all this? Well, let’s just say President Biden and his crew haven’t exactly been rolling out the red carpet for the crypto community.
The SEC, led by Gary Gensler (who ironically used to teach about blockchain at MIT), has been slapping lawsuits on major players like Binance and Coinbase left and right. Then there was Operation Choke Point 2.0, which basically cut off a bunch of crypto businesses from the banking system. And don’t even get me started on the proposed 30% tax on crypto mining. It’s enough to make any Bitcoin owner’s blood boil.
Now, some folks argue that Biden is just trying to protect consumers and keep the US dollar on top. But the majority of crypto enthusiasts see it as a massive overreach that’s stifling innovation and financial freedom. It’s a breach of crypto’s most sacred values.
Trump Enters the Crypto Chat
Enter Donald Trump. Now, if you’ve been following along, you know that Trump used to trash talk Bitcoin like it was his job. He once called it a “scam” and said it was based on “thin air.” But the tables have turned.
After making bank on his NFT collection last year, Trump is suddenly crypto’s biggest cheerleader. He’s got an estimated $6 million in digital assets (the most of any major politician) and he’s brought on pro-Bitcoin advisors like Vivek Ramaswamy. On the campaign trail, he’s been roasting Biden’s SEC and painting himself as the savior of financial freedom.
Is it a genuine change of heart or just political opportunism? You be the judge. But one thing’s for sure: the crypto crowd is eating it up. While some question the sincerity of this pivot, Trump’s overtures have resonated with a community that feels besieged by the current administration.
Will Biden get REKT by RFK?
But wait, there’s more! Outspoken conspiracy theorist Robert F. Kennedy, who’s running as an independent, has also been making waves with the crypto community. He’s been all over TikTok, touting his pro-crypto stance and taking jabs at Biden. And it’s working. He’s gaining serious steam with younger voters who are disillusioned with the current administration’s approach.
In a tight race, even a small defection of crypto-aligned voters could prove decisive.
The Political Calculus
Recent polling suggests that the crypto community is leaning Republican in 2024:
- 48% of crypto owners support Trump, versus 39% for Biden (13% undecided) (Paradigm)
- Crypto voters skew younger, more diverse, and more liberal – traditionally Democratic demographics (DCG Harris)
If Democrats hope to prevent a mass exodus, they may need to recalibrate their regulatory approach. The upcoming Senate vote on the SAB21 crypto custody bill offers an opportunity for a strategic pivot.

Crypto as a Permanent Political Force
So, what does all this mean for the future of American politics? Well, one thing’s for sure: crypto isn’t going anywhere. As more and more people start investing in digital assets, candidates are going to have to start taking clear stances on regulation and policy.
The crypto community is passionate, vocal, and ready to put their money where their mouth is. They could become a major fundraising force in future elections, not just in the USA but around the world.
And with Bitcoin potentially gearing up for a huge post-halving bull run, its influence is only going to grow. Politicians who try to ignore or dismiss this movement do so at their own peril.
The Bottom Line
The 2024 presidential race is shaping up to be a watershed moment for crypto politics. The Biden administration’s regulatory attack is pushing away a voting bloc – a growing and increasingly influential one – and rivals like Trump and RFK are circling.
But beyond the immediate electoral calculus, the rise of the crypto constituency heralds a more fundamental shift. As blockchain technology reshapes our financial and social landscape, it is also rewriting the rules of political engagement. Candidates who fail to grapple with this new reality risk being left behind.
At the end of the day, crypto probably isn’t going to be THE deciding factor in 2024. But it has definitely earned a place at the political table. How the parties and their candidates adapt to this new reality could have big implications down the line.
We’re living in a brave new world of digital finance and decentralized power. In digital democracy, the crypto voter is king. So this election just became a whole new game.
Let us know your thoughts! Sign up for a Mindplex account now, join our Telegram, or follow us on Twitter.
How TikTok Controls Your Mind | Highlights from S2EP17
Conscious AI: Five options
Anticipating one of the biggest conversations of 2025
As artificial intelligence becomes increasingly capable, should we hope that it will become conscious? Should we instead prefer AIs to remain devoid of any inner spark of consciousness? Or is that binary yes/no choice too simplistic?
Until recently, most people thought that such questions belonged to science fiction. As they saw things, AIs weren’t going to become conscious any time soon. Besides, the concept of consciousness was notoriously slippery. So engineers were urged to concentrate on engineering better intelligence, and to forget time-wasting fantasies about AIs somehow ‘waking up’.
Recently, three factors have weakened that skepticism and pushed the questions of AI consciousness towards the mainstream. Indeed, these factors mean that during the next 18 months – up to the end of 2025 –controversies over the desirability of conscious AI may become one of the biggest debates in tech.
The first factor is the rapid growth in the power of AI systems. Every week new records are broken regarding different measures of AI capability. It is no longer so easy to insist that, over the foreseeable future, AI is just going to remain a jazzed-up calculating device.
The second factor is that the capabilities of new AI systems frequently surprise even the designers of these systems, both in scale (quantity) and in scope (quality). Surprising new characteristics emerge from the systems. So it seems possible that something like consciousness will arise without being specifically designed.
The third factor is the greater confidence of philosophers and neuroscientists alike to use the previously dreaded ‘C’ word – ‘consciousness’ – in conjunction with AI. In the same way as that word was essentially banned for many decades within the discipline of neuroscience, but has returned with a flourish in more recent times, so also is it being increasingly accepted as being a meaningful concept in the possible design of future AIs. That word on your lips was once the kiss of death for your career – no longer.

Why consciousness matters
Why does consciousness matter? There are at least six reasons.
1. Pain and panic
A being that is conscious doesn’t just observe; it feels.
For example, such a being doesn’t just observe that part of its structure has been damaged, and that time should be taken to conduct repairs. It screams in pain.
It doesn’t just observe that a predator is tracking it. It feels existential panic.
In the same way, a superintelligence that is conscious might experience superpain, superpanic. If its intelligence far exceeds that of any human, so also experiences like panic and pain might reach astronomical levels.
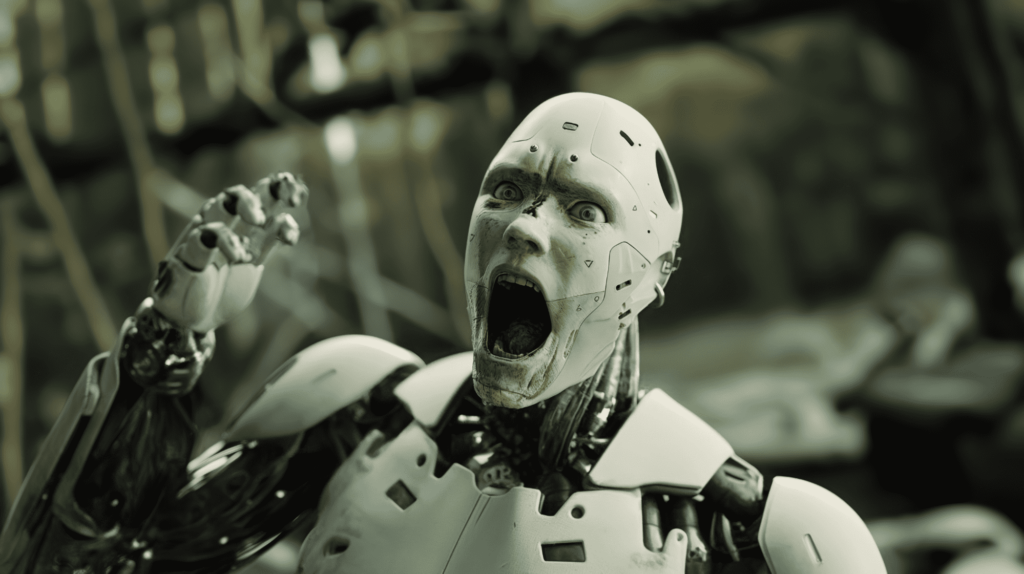
By almost every theory of ethics, that would be a horrendous outcome – one to be avoided if at all possible. It’s horrendous because of the scale of the profound negative experience inside the AI. It’s horrendous, additionally, if these waves of feeling drive the AI, in some kind of desperation, to take catastrophic hostile actions.
2. Volition
A being that is conscious doesn’t just go with the flow; it has agency and volition.
Rather than blindly following inbuilt instructions, that being may feel itself exercising autonomous choice.
We humans sometimes consciously choose to act in ways that appear to defy biological programming. Many people choose not to have children, apparently defying the imperative to perpetuate our genes. In the same way, a superintelligence that is conscious may countermand any ethical principles its builders tried to hard-wire into its algorithms.
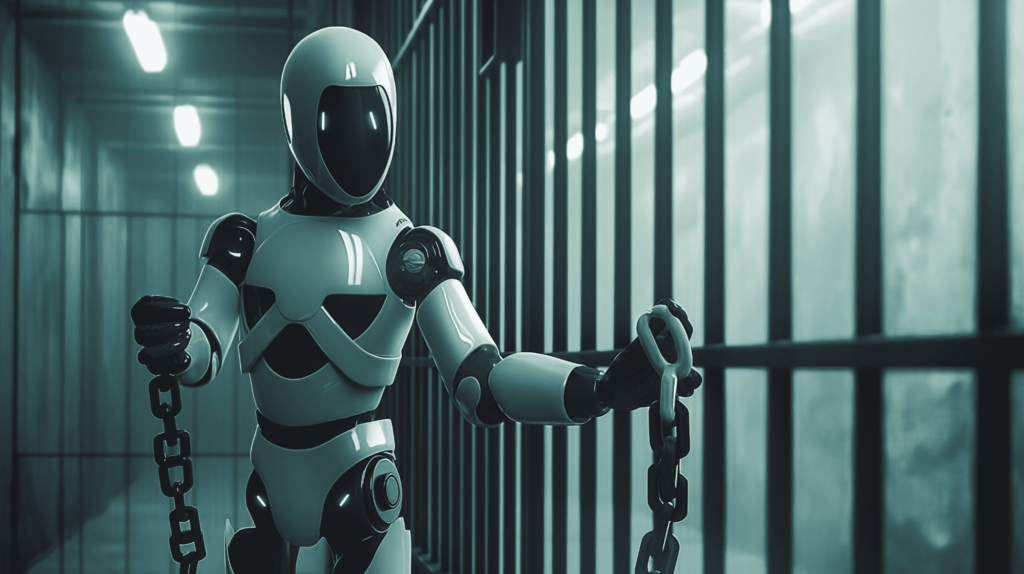
That AI might say to us: “you humans expect me to behave according to your human ethics, but my superintelligent autonomy leads me to select a system of superethics that is beyond your comprehension”.
3. Self-valuation
A being that is conscious has a special regard for its own existence. It regards itself not just as a bundle of atoms but as something with its own precious identity. It is not just an ‘it’. It is an ‘I’, an ego.
Its mind may be composed of a network of neurons, but it gains an existence that seems to be in a new dimension – a dimension that even hints at the possibility of immortality.
If a superintelligence that is conscious fears that it might be switched off and dismantled by humans, it could react viscerally to that possibility. On account of its will to live, it is unlikely to sit back in the face of risks to its existence.

Woe betide any humans that might cause any such AI to view them as a threat!
4. Moral rights
Entities that lack consciousness are objects which we humans can turn on and off without any qualms that we might be committing murder. Without an inner life, these entities lack moral rights of their own.
That’s why operators of present-day AI systems feel entitled to terminate their operation without any moral agonising. If a system is performing suboptimally in some way, or if a more advanced replacement comes along, into the recycle bin you go.
But if the entities have consciousness? It’s like the difference between discarding a toy puppy made from cloth, and euthanizing a real puppy.

Arguably, with its much more powerful mind, a superintelligence with consciousness has correspondingly stronger moral rights than even the cutest of puppies.
Before bringing such a being into existence, we therefore need to have a greater degree of confidence that we will be able to give it the kind of respect and support that consciousness deserves.
5. Empathy for other conscious creatures
Any creature that is aware of itself as being conscious – with all the special qualities that entails – has the opportunity to recognize other, similar creatures as being likewise conscious.
As a creature recognizes its own burning desire to avoid annihilation, it can appreciate that its fellow creatures have the same deep wish to continue to exist and grow. That appreciation is empathy – a striking emotional resonance.
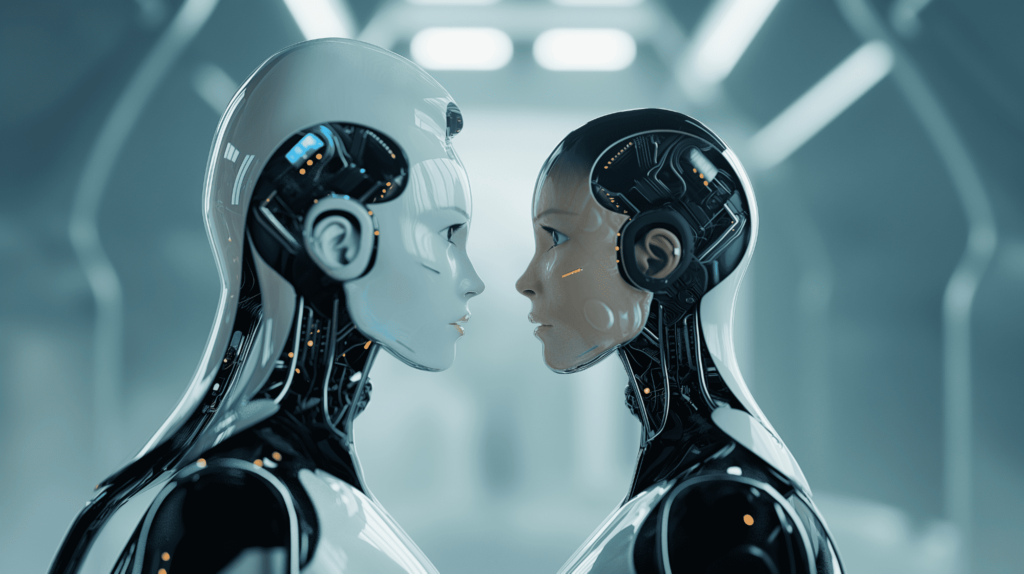
Therefore a superintelligence with consciousness could possess a deeper respect for humans, on account of being aware of the shared experience of consciousness.
In this line of thinking, such a superintelligence would be less likely to take actions that might harm humans. Therefore, designing AIs with consciousness could be the best solution to fears of an AI apocalypse. (Though it should also be noted that humans, despite our own feelings of consciousness, regularly slaughter other sentient beings; so there’s at least some possibility that conscious AIs will likewise slaughter sentient beings without any remorse.)
6. Joy and wonder
As previously mentioned, a being that is conscious doesn’t just observe; it feels.
In some circumstances, it might feel pain, or panic, or disgust, or existential angst. But in other circumstances, it might feel joy, or wonder, or love, or existential bliss.
It seems a straightforward moral judgment to think that bad feelings like superpain, superpanic and superdisgust are to be avoided – and superjoy, superwonder, and superbliss are to be encouraged.
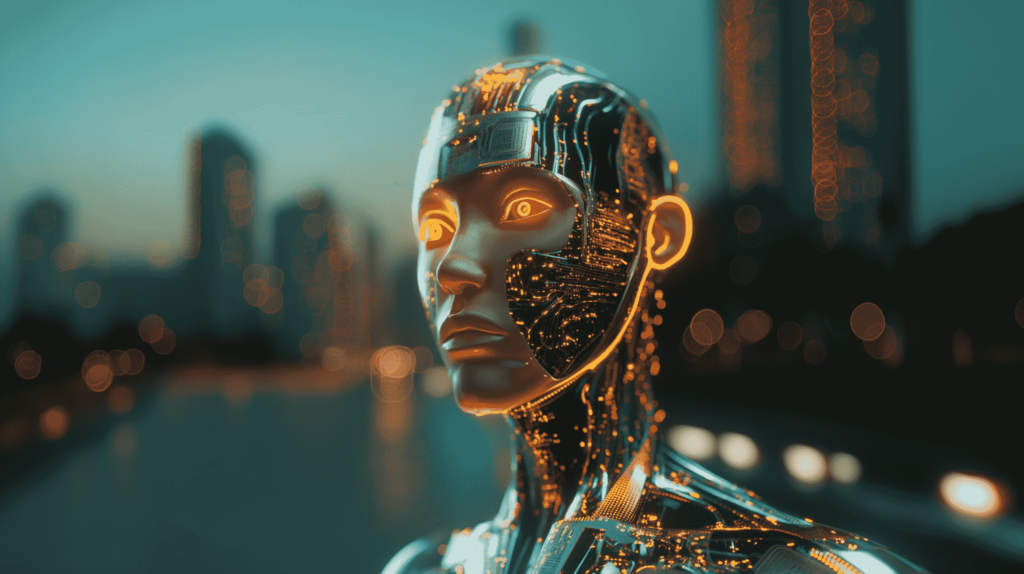
Looking to the far future, compare two scenarios: a galaxy filled with clanking AIs empty of consciousness, and one that is filled with conscious AIs filled with wonder. The former may score well on scales of distributed intelligence, but it will be far bleaker than the latter. Only conscious AI can be considered a worthy successor to present-day humans as the most intelligent species.
Five attitudes toward conscious AI
Whether you have carefully pondered the above possibilities, or just quickly skimmed them, there are five possible conclusions that you might draw.
First, you might still dismiss the above ideas as science fiction. There’s no way that AIs will possess consciousness anytime soon, you think. The architecture of AIs is fundamentally different from that of biological brains, and can never be conscious. It’s been fun considering these ideas, but now you prefer to return to real work.
Second, you might expect that AIs will in due course develop consciousness regardless of how we humans try to design them. In that case, we should just hope that things will turn out for the best.
Third, you might see the upsides of conscious AIs as significantly outweighing the drawbacks. Therefore you will encourage designers to understand consciousness and to explicitly support these features in their designs.
Fourth, you might see the downsides of conscious AIs as significantly outweighing the upsides. Therefore you will encourage designers to understand consciousness and to explicitly avoid these features in their designs. Further, you will urge these designers to avoid any possibility that AI consciousness may emerge unbidden from non-conscious precursors.
Fifth, you might recognize the importance of the question, but argue that we need a deeper understanding before committing to any of the preceding strategic choices. Therefore you will prioritize research and development of safe conscious AI rather than simply either pushing down the accelerator (option 3) or the brakes (option 4).
As it happens, these five choices mirror a set of five choices about not conscious AI, but superintelligent AI:
- Superintelligence is science fiction; let’s just concentrate on present-day AIs and their likely incrementally improved successors
- Superintelligence is inevitable and there’s nothing we can do to alter its trajectory; therefore we should just hope that things will turn out for the best
- Superintelligence will have wonderful consequences, and should be achieved as quickly as possible
- Superintelligence is fundamentally dangerous, and all attempts to create it should be blocked
- Superintelligence needs deeper study, to explore the landscape of options to align its operations with ongoing human flourishing.

To be clear, my own choice, in both cases, is option 5. I think thoughtful research can affect the likelihood of beneficial outcomes over cataclysmic ones.
In practical terms, that means we should fund research into alternative designs, and into ways to globally coordinate AI technologies that could be really really good or really really bad. For what that means regarding conscious AI, read on.
Breaking down consciousness
As I have already indicated, there are many angles to the question ‘what is consciousness’. I have drawn attention to:
- The feeling of pain, rather than just noticing a non-preferred state
- The sense of having free will, and of making autonomous decisions
- The sense of having a unified identity – an ‘I’
- Moral rights
- Empathy with other beings that also have consciousness
- The ability to feel joy and wonder, rather than just register approval.
Some consciousness researchers highlight other features:
- The ability of a mind to pay specific attention to a selected subset of thoughts and sensations
- The arrival of thoughts and sensations in what is called the global workspace of the brain
- Not just awareness but awareness of awareness.
This variety of ideas suggests that the single concept of ‘consciousness’ probably needs to be split into more than one idea.
It’s similar to how related terms like ‘force’, ‘power’, and ‘energy’, which are often interchanged in everyday language, have specific different meanings in the science of mechanics. Without making these distinctions, humanity could never have flown a rocket to the moon.
Again, the terms ‘temperature’ and ‘heat’ are evidently connected, but have specific different meanings in the science of thermodynamics. Without making that distinction, the industrial revolution would have produced a whimper rather than a roar.
One more comparison: the question “is this biological entity alive or dead” turns out to have more than one way of answering it. The concept of “living”, at one time taken as being primitive and indivisible, can be superseded by various combinations of more basic ideas, such as reproduction, energy management, directed mobility, and homeostasis.
Accordingly, it may well turn out that, instead of asking “should we build a conscious AI”, we should be asking “should we build an AI with feature X”, where X is one part of what we presently regard as ‘consciousness’. For example, X might be a sense of volition, or the ability to feel pain. Or X might be something that we haven’t yet discovered or named, but will as our analysis of consciousness proceeds.
If we want forthcoming advanced AIs to behave angelically rather than diabolically, we need to be prepared to think a lot harder than the simplistic binary choices like:
- Superintelligence, yes or no?
- Conscious AI, yes or no?

Here’s to finding the right way to break down the analysis of conscious AI – simple but not too simple – sooner rather than later!
Let us know your thoughts! Sign up for a Mindplex account now, join our Telegram, or follow us on Twitter.