Let us know your thoughts! Sign up for a Mindplex account now, join our Telegram, or follow us on Twitter.
Large Language Models – From Banality to Originality
Looking at the insanely popular Large Language Models (LLMs) like ChatGPT with an AGI researcher’s eye, what I see are some potentially interesting components for future AGI systems.
Economically, I see the seed of a family of technologies that, over the next few years, is very likely to obsolete a majority of white-collar jobs, wreaking incredible disruption and ultimately moving us further toward a world in which people are liberated from the necessity to work for a living.
On the other hand, looking at them from an end user’s view, what I see are tools that are already pretty useful for some things – but are marred by a few really major and frustrating flaws.
The first major flaw is a bizarre lack of reality discrimination – LLMs are so full of shit it’s almost unbelievable, given how intelligent they are in some ways.
The second is a mind-numbing boringness and banality – such powerful cliché machines have never previously even been imagined.
I do believe, though, both of these issues can be overcome with a moderate amount of R&D effort. Which is probably going to be put in by various parties during the next couple years.
To fully overcome these weaknesses will require a true breakthrough to Artificial General Intelligence. But I suspect they can be remedied to a significant degree even without AGI, as a parallel track of development.
Generative AI vs. AGI
As an AGI guy, the first thing I need to clarify when talking about LLMs is that they are certainly not AGIs. They achieve a fair degree of generality in their intelligence, but it’s not because they generalize beyond their training and programming – it’s because their training data is so huge it covers almost everything. A tremendous variety of queries can be answered via minor variations and combinations on things in the training data.
A generally intelligent artificial mind will have lots of uses for statistically recognizing patterns in huge datasets, and synthesizing new forms via merging these patterns together in a manner appropriate to some narrow context – the things that LLMs are good at. But I don’t think these capabilities can form the core of an AGI mind. That needs much more abstract thinking, bound up with agency, will, self-reflection, modeling of self and other. If AGI systems have these core functions fulfilled (by the OpenCog Hyperon AGI architecture my colleagues and I are working on, or something else), I think LLMs could add a lot of wonderful peripheral functions.
Toward LLMs with Reality Discrimination
LLMs trained to produce text currently have serious problems with truth-telling – they basically can’t distinguish truth from falsehood. I’ve gathered a few funny or instructive examples in a blog post on ChatGPT and AGI. There are many others easy to dig up online. I have a particular liking for the conversation where ChatGPT calls me the founder of OpenAI, the company that created it (in which I have no direct role).
A variety of approaches are being pursued to overcome this problem and make LLMs less full of shit, and I think a bunch of them will probably succeed to some degree. As an example, we at SingularityNET and TrueAGI are trying to address this with a neural-symbolic architecture: LLMs are hybridized with an OpenCog-based symbolic reasoning engine.
This symbolic reasoning engine can extract logical relationships from an LLM, and put the LLM’s knowledge in a more structured form. It then compares the relationships between concepts with each other to identify inconsistencies. Where possible, it also compares these relationships with existing background knowledge – common-sense truths about the world like ‘water is wet’ or ‘Ben Goertzel is not the founder of OpenAI’. Inconsistencies can then be used as negative evidence to fine-tune the model – the model can be explicitly trained to unlearn the lies it contains.
I suspect these hybrid symbolic-LLM structures can greatly increase the generality and truthfulness of things like ChatGPT, even without taking us all the way to AGI.
The Banality of LLMs
But LLMs also have other problems besides their rampant BS generation – like the fact that so much of what they produce is boring clichés. Eric Hoel has called this “the banality of ChatGPT“.
As a so-bad-it’s-good example of this banality, consider the following excerpt from a recent dialogue I had with the system:
BEN: How would you advise to manage and coordinate the project of creating a replica of the planet Jupiter with 1000x human intelligence?
ChatGPT: For managing and coordinating a project of creating a replica of Jupiter with 1000x human intelligence, I would suggest the following steps:
- Define the project scope: Clearly define the goals, objectives, and deliverables of the project.
- Assemble the team: Recruit individuals with relevant skills and experience to form a diverse and effective team.
- Develop a project plan: Determine the tasks, resources, timelines, and budgets required to complete the project.
- Set up project management systems: Establish tools and processes to track project progress, communicate with team members, and manage risks.
- Monitor progress and adjust as needed: Continuously assess the project’s status and make changes as needed to ensure it stays on track.
- Ensure communication and collaboration: Encourage regular communication among team members and stakeholders to ensure everyone is on the same page.
- Anticipate and manage risks: Identify and plan for potential risks and obstacles to minimize their impact on the project.
- Celebrate success: Recognize and celebrate milestones and achievements along the way to keep team morale high.
Remember, creating a replica of Jupiter with 1000x human intelligence is a complex and challenging project, so it’s important to be organized, communicate effectively, and continuously adjust as needed to ensure success.
Not exactly wrong. But, shall we say, not really grappling with the particulars of the question…
Sometimes boring clichés are exactly what you want in life. If you’re writing a cover letter for a job application, or an essay for university English class… originality and quirkiness can sometimes be dangerous.
But one worries about a near-future in which boring clichés become even more common than they are now, because they’re so quick and cheap to generate using AI models. A boring dystopia.
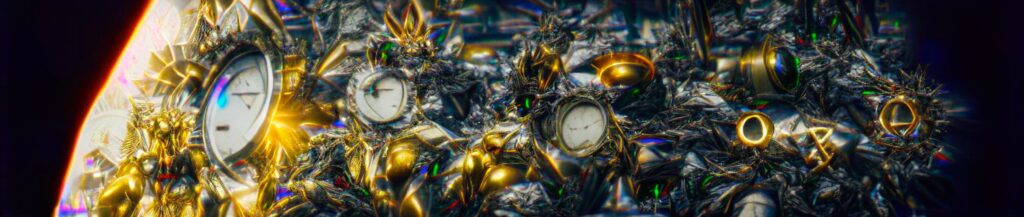
The Glory and Horror of Music LLMs
Google’s recently announced MusicLM neural model provides an intriguing case study of the intersection between “fascinatingly impressive” and “horrifyingly boring.”
You can give it a query like –
We can hear a choir, singing a Gregorian chant, and a drum machine, creating a rhythmic beat. The slow, stately sounds of strings provide a calming backdrop for the fast, complex sounds of futuristic electronic music
.
– and it will generate music that fits the bill. Amazing stuff.
Except the effect is a bit like having a workaday lounge band improvise a musical passage for you. It’s very rarely artistically thrilling.
Given how impressive the functionality is, you might say this is a pretty lame complaint.
However, if such technology was used to generate music for people to listen to, the result would be an even more cliché-ridden and repetitious music sphere than record execs have already inflicted on us! Dentist’s office muzak++ forever!
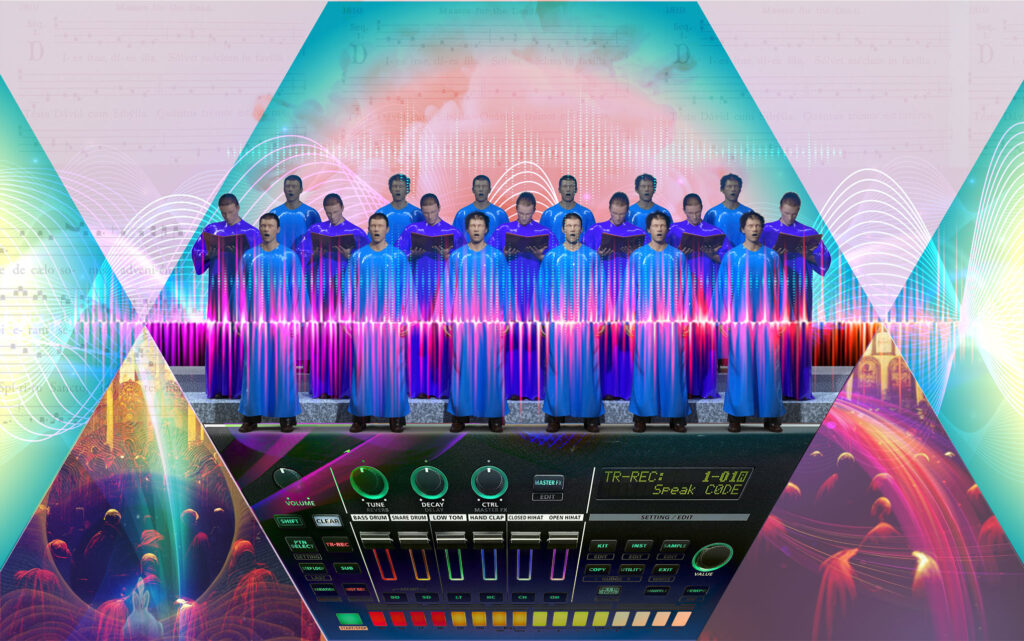
The problem here is that averaging together everybody’s art produces art that is itself average. For some commercial purposes – e.g. background music for ads or video games – average, passable, competent music may be fine.
As a lifelong composer and improviser, I’ve generally been more interested in creating sounds that diverge from the average and the expectation – even if they get a little jarring or discordant in the process.
Of course, current neural models can be jarring and discordant too – but they will tend to do it in a way quite similar to something from their training dataset, or combining surface-level features of a few things in their training datasets.
Music is the domain in which I’ve thought most about how to overcome the banality of LLM output – because as a musician, I would really love to have an AI musician to improvise with. We already have a robot singer in our Jam Galaxy Band, and some AI-composed riffs, but a real-time AI improviser jamming alongside me is what I dream of. I don’t want boring lowest-common-denominator MusicLM-style in my band, not at all…
One approach that can be taken here is to formally introduce a theory of ‘interestingness’ – make a mathematical model of what constitutes interesting music, and then condition a MusicLM-type model to bias it to produce outputs meeting this interestingness criterion. This is not that far off from work I did in the 1990s using genetic algorithms to evolve music maximizing a ‘fitness function’ encoding a theory of musical interestingness. But LLMs allow the evolved music to incorporate patterns of various sorts from human music in a much more refined way than was possible back then.
LLMs vs. Hmmmmmm
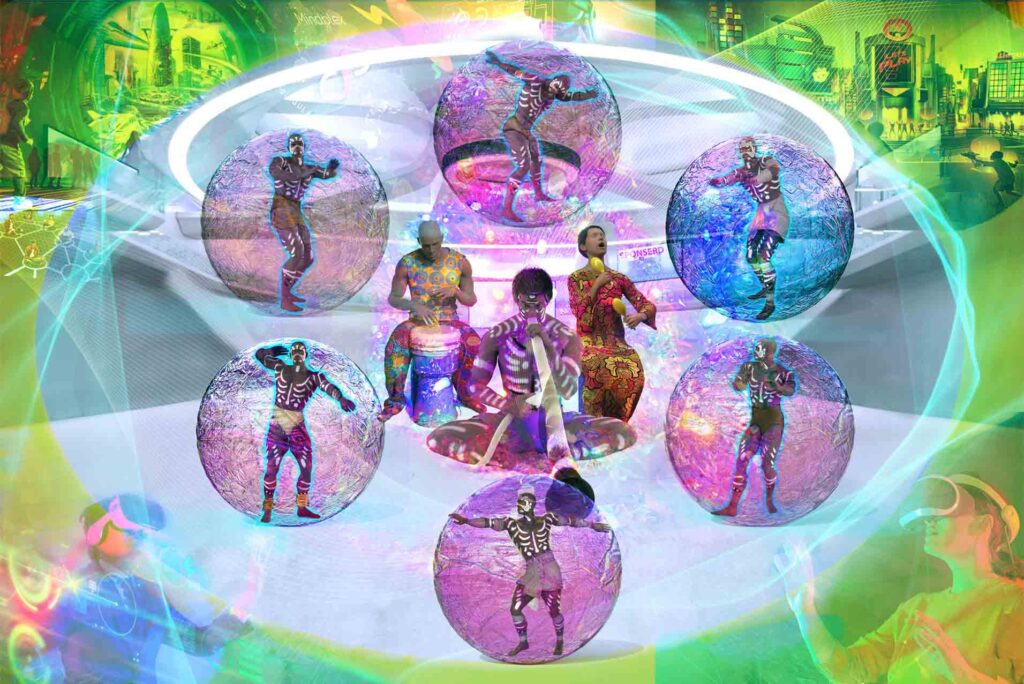
Of course, this would still be a very different thing from how an AGI system would approach music.
AGI and music could intersect in a variety of ways, but one way or another, it would involve an AGI system creating and understanding music in the context of its experience of being an agent in the world, like when the AGI in the 2013 film Her says, “I’m trying to write a piece of music that’s about what it feels like to be on the beach with you right now.”
Steven Mithen’s book The Singing Neanderthals presents an hypothesis about the origin of language and music. He posits that human communication began with a communication system he refers to as “Hmmmmm” because it had the following characteristics: it was Holistic, manipulative, multi-modal, musical and mimetic. Basically Hmmmmm combined sound and gesture and action and imitation – somewhat like the pre-verbal/semi-verbal communication one sees in one-year-old children, but with more adult-level cognitive sophistication underlying. His proposal is that Hmmmmm came first and then spawned both language and music, which evolved from Hmmmmm in their own different directions.
Cambridge Archeological Journal did a fascinating feature presenting various criticisms on the hypothesis along with Mithen’s responses.
An interesting and fairly difficult challenge would be to coax AI agents living in a virtual world – let’s say Minecraft enhanced with plugins, or the in-process Sophiaverse virtual world – to invent language and music along the lines of the Hmmmmm theory. This could be an interesting and valuable thing for AGI researchers to do regardless of how fully accurate Mithen’s theory of evolution is.
We could stock the virtual world with a few easy-to-use musical instruments, let’s say –
- drums that make rhythms when hit
- flutes that they breathe into (modulating volume and timbre with breath) while pushing buttons to make notes
- Piano-type instruments that make notes when their keys are hit
One would then ultimately want these virtual-world proto-AGI agents – I like to think of them as “Neoterics” (new people) – to do things like:
- Discover that dancing to music is pleasurable and creates a feeling of togetherness which fosters collective action and communication
- Discover that drumming enthuses a group to carry out physical tasks together
- Discover that listening to melodic music puts the mind in a state conducive to creativity
Given that the Neoterics’ emotion models will be similar to ours, yet different in the particulars, it may be that the music they create to express their own emotions and influence each others’ emotions will be significantly different from human music. Perhaps one could then train music LLMs on music made by Neoterics and get a fascinating sort of hybrid – a truly new genre of music!
Whether or not this Neoterics experiment ever gets done in precisely this form, it does highlight the big difference between an LLM approach and an AGI approach – to music or anything else. LLMs are munging and merging data patterns, and with cleverness one can work around the most immediate issues that emerge from this approach, issues such as tendencies to hallucinate or converge on clichés. AGI, however, requires a totally different approach.
Narrow AI systems like LLMs may be useful for feeding patterns into the core cognition of AGI systems, or for helping them speak fluently in the lingo of a given domain. But at core, AGI systems will necessarily be very different from LLMs – they will be what Weaver has called Open-Ended Intelligences – complex self-organizing systems that engage richly with their environments, driven by complementary-and-contradictory drives to individuation and self-transcendence. AGI systems will achieve generalization via abstraction, and the need to balance individuation and self-transcendence, while working with limited resources, will drive them to intelligent abstract understanding. When they generate language or make music, they will build it on this abstract understanding, formed from their experience, and as a result will be imaginative and truth-telling naturally – organically – rather than with an additional trick.
LLMs and other deep neural nets are going to have a big impact on society, disrupting the business models of today’s tech giants and potentially eliminating a significant percentage of human jobs. But their biggest contribution ultimately may be waking up the world to the potential of AI and thus attracting more cognitive, financial and cultural resources toward the development of AGI, which will have a far larger direct impact than even the coolest narrow AI systems.
Let us know your thoughts! Sign up for a Mindplex account now, join our Telegram, or follow us on Twitter.
Technoshaman: from worldbuilding to mindbuilding: Part 2
In Part 1, I introduced the archetype of the technoshaman, one who uses XR (extended reality — VR, AR, and MR) technologies to craft multisensory digital worlds and experiences that elevate and harmonize human consciousness on a mass scale. In Part 2, we deep-dive into “digital pharmacology” and use XR to evoke alternate states of consciousness.
The social impacts of contemporary media are well known. Film and television can engage, inform and motivate on a mass scale and are no longer solely dominated by major studios who must make significant investments in productions. And the increasing democratization of media has placed great power in the hands of nearly every individual.
More than 6.5 billion people — 80% of the world’s population — now own smartphones. Social media has enabled nearly anyone to influence the minds of hundreds, thousands, or millions of people with videos, news, opinions and niche programming.
Storytellers intentionally use the power of media and entertainment to achieve social or global change through social impact entertainment. As described by Ella Saltmarsh, stories can be tools for “building community through empathy and coherence,” connecting diverse people and generating “narratives that hold together groups, organizations, and movements.”
Stories can re-author our personal and cultural narratives and mythic narratives or worldviews, including values, mindsets, rules and goals. “Stories deeply affect our psyche and collective direction of travel,” Saltmarsh says.
Unlike film, television, or streaming media, where passive audiences watch visuals framed on a wall or a handheld device, XR (extended reality) worldbuilders use presence, embodiment, and agency for spatial or environmental storytelling. These place us within the “storyworld” with XR’s ability to simulate real-world experiences, giving this new medium a deeper, more profound effect on consciousness.
The power of XR
Everyone in the metaverse can become a worldbuilder, giving them the power to evoke memes, moods, and states of consciousness in other participants. The result is akin to a virtual brain corpus callosum between groups and individuals, allowing for direct transmission of consciousness from worldbuilder to participant.
Of course, music, arts, entertainment, architecture, literature, and other real-world modes of expression have long been used to evoke profound brain states in participants. But XR supercharges this process, due to various factors:
- XR interfaces create a wideband interface to the participant’s brain and nervous system. Immersive displays can place a pixel on nearly every nerve ending in the eye. 3D audio simulates spatial and spectral properties of sound. And XR controllers capture natural movement and gestures.
- XR production tools and robust user interfaces, enhanced by AI, accelerate worldbuilding without the need to write code. Virtual worldbuilding is not burdened by the need for physical materials, construction, labor, and logistics, vastly accelerating the democratization of virtual worldbuilding compared to physical worldbuilding.
- XR performers can easily inhabit and command virtual worlds, controlling complex interactions and effects through natural gestures and simple controllers. Imagine a live theater that suddenly morphs into a cave, a castle, or another world altogether.
- Virtual worlds combine music, arts, entertainment, storytelling, social media, architecture, and multisensory modalities into a seamless experience for participants, compounding their psychological power.
- Unlike physical spaces, virtual environments are inexpensive, easily replicated, and massively scalable with global reach.
The metaverse
The XR technology market will expand by a factor of 10 in the next five years, according to a forecast by Statista. Over the long term, wearable XR interfaces are expected to replace handheld smartphones. And some expect the metaverse to replace the internet, despite Meta’s problems.
To articulate and guide the development of the metaverse “for the greatest good for the greatest number” of participants, virtual reality pioneer Tony Parisi recently proposed these Seven Rules of the Metaverse:
- There is only one Metaverse
- The Metaverse is for everyone
- Nobody controls the Metaverse
- The Metaverse is open
- The Metaverse is hardware-independent
- The Metaverse is a Network
- The Metaverse is the Internet
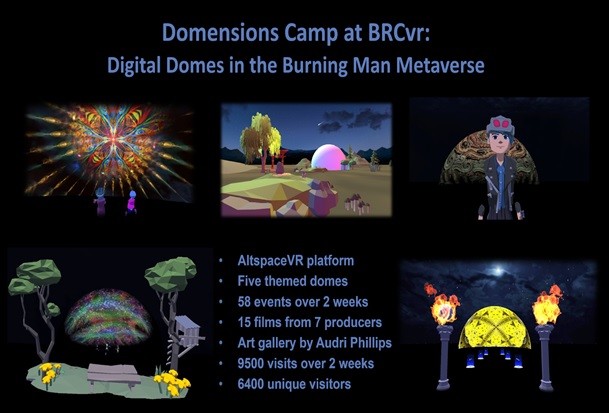
Microsoft’s AltspaceVR platform (credit: Vortex Community Circle)
Widespread adoption of the metaverse using XR interfaces will place unprecedented power and influence into the hands of worldbuilders. But will worldbuilders use this power wisely?
Digital pharmacology
XR technologies can extend beyond simple storytelling. Immersive multisensory media can evoke specific states of consciousness, or “digital pharmacology.” Applications could include enhanced entertainment, education, leisure and lifestyle, enhanced well-being, spiritual ceremonies, and a wide variety of clinical applications.
There’s a rich history of sensory experiences being used to alter consciousness. Music is a powerful mood-altering agent that can induce or modify cognitive states and perceptions. Sound healing and vibroacoustic healing have been proven effective. Simple nature sounds can improve health, increase positive affect, and lower stress.
Visual stimuli such as natural environments — both real and virtual — can similarly affect physiological states (as measured by blood pressure and muscle tension). They can even alter our perception of music. Other powerful sensory modalities include haptics (touch) and aroma.
Transformational and mood-elevating experiences can take on many forms. Here’s a partial list:
- Awaken the Mind
- Inspire imagination, creativity, and open-mindedness
- Educate, train, inform
- Epiphanies and new understandings
- Open the Heart
- Empathy, compassion, understanding
- The emotional shift towards love, respect, joy, happiness
- Activate the Spirit
- Awe, wonder, ecstasy, bliss
- Transcendent, numinous or “unity” experiences
- Cosmic consciousness
- Going Deep
- Mindfulness and contemplative states
- Trance, dream, and mystical states
- Psychedelic states
- Soothe the Beast
- Relaxation, sense of calmness
- Feeling of safety, restoration
- Awaken the Senses
- Arouse passion, romance, sensuality
- Energize and Engage
- Awaken the body and mind
- Stimulate, motivate, alert, play
- Entrain the Brain
- New kinesthetic skills, break/make habits, reprogram
Mindfulness states have a broad range of health benefits. Studies have shown decreased anxiety and depression, improved immune function, mitigation of cognitive decline due to aging, increased mental clarity and focus, improved heart health and mental health, increased longevity and self-confidence, improved moods, improved sleep, pain mitigation and more.
Likewise, studies have shown that psychedelic states can treat obsessive-compulsive disorder, end-of-life psychological distress, alcohol and tobacco addiction, and major depressive disorder. More research is needed to validate the degree to which XR experiences can evoke such states. However, XR applications are emerging to guide patients receiving psychedelic therapies.
Awe is one of the most powerful emotions one can experience. In a partnership with Cirque du Soleil, Dr. Beau Lotto’s Lab of Misfits conducted an ambitious study on the emotion of awe. The findings suggest that awe motivates us to step forward into a world of uncertainty in the search for answers, raises our risk tolerance, increases social behavior, and can even reframe who we believe we were in the past. Multiple studies have also found evidence that experiencing awe makes us kinder and more generous. These are all good things for this day and age!
Let us know your thoughts! Sign up for a Mindplex account now, join our Telegram, or follow us on Twitter.
Explaining Mindplexes | Highlights: Episode 1 of the Mindplex Podcast feat. David Brin
How to raise an AGI Toddler | Highlights: Episode 1 of the Mindplex Podcast feat. David Brin
Delusional Humans and AGI | Highlights: Episode 1 of the Mindplex Podcast feat. David Brin
The Sensor Fusion Guide by Ryan Sternlicht
This guide goes with this article “The World of Sensors” by Alex Milenkovic
INTRO
Whether we know it or not, humanity is quickly entering into an era of technology, where biosensing, biometrics, and the quantified self are combining into something transformative. These techniques will allow us to gain profound insight into ourselves, how we do things, how we think, how we are similar, and how we differ as well. Have you ever wanted to control a robot with your mind, or interact with virtual characters that respond to your emotions, what about developing a health and exercise plan specific to you that is on par with the trainers that professional athletes get. What about being able to predict the future of your own health? Biosensor fusion can allow all of that and much more.
In our World of Sensors article, we learned about biosensors, their data, and how they will shape our world. This accompanying device chart explains in detail the kind of data that all of the different types of biosensors collect, and also provides a comprehensive “device guide” for comparison.
So let’s take a look at how each of these sensors work individually.
OVERVIEW
Our bodies produce a lot of different signals, which contain distinct markers and those are measured by the different sensors. These sensors give us an understanding of our body that would be hidden from us otherwise.
An exciting recent development is that many of these sensors are starting to become available to consumers and developers, bypassing the need to spend significant amounts of money, be associated with a tech company or be a part of an educational institution.
Before we can understand biosensor fusion in detail we should describe the biosensors themselves that are available, including the pros and cons of various sensors. We will discuss what each one brings to the table, the drawbacks they present, how they are best used, and how they can be combined with each other.
Technology: Eye Tracking sensors
This sensor is probably the easiest to understand as well as implement for analysis. It has been incorporated into a number of AR and VR headsets, and is available as an accessory for desktop computers as well. Most Eye Trackers use infrared emitters to shine light into your eyes. The pupils absorb the light, the rest of your eye reflects. This allows the eye tracker to accurately calculate your eye position. Some eye trackers use regular Cameras to measure and compute eye position, and although rare, you can also use an array of electrodes around the eye to track where the eye is looking, called EOG (electrooculography).
Current expectations is that Valve/OpenBCI’s galea headset will have both EOG and built in camera based eye tracking, which would be an easy form of sensor fusion. This is because EOG can have much higher temporal resolution then current eye tracking cameras, which makes the data more accurate and precise, and the EOG data can be used to further enhance the performance of foveated rendering which is highly time sensitive. This is then coupled with the benefits of camera based eye tracking, which offers decent accuracy in a passive collection method. Further, camera based eye trackers can measure your pupil size and changes which is an extremely valuable measurement as it can be used to determine your current cognitive load. Also camera based eye trackers can be further away from the user, such as on a desk, or attached to a monitor. Or mounted to a pair of glasses or around a VR headset’s lenses.
Eye tracking presents us with a great example of how Sensor fusion can lead to an improved and more accurate picture of a person. HP recently released the HP Omnicept G2 VR headset, offering a combination of PPG based Heart Rate tracking and camera based eye tracking. This device offers a custom metric in the SDK, a cognitive load calculation. This metric represents how much of the user’s cognitive capacity is in use at a given moment – on a scale of 0 to 100. This metric is computed using both pupil size and heart rate variability, which can provide a higher accuracy metric of cognitive load than either sensor alone. This calculation is also thanks to a machine learning algorithm to look at the data and give a prediction of what the current cognitive load is based on that training.
(more on the hp research can be found here
https://developers.hp.com/system/files/attachments/HPO-CLD%20Technical%20Report%204.30.21.pdf
Technology: Heart Rate sensor
This is probably the most likely sensor that you might be wearing right now. If you are wearing a recent Apple watch, fitbit, smart watch, or any fitness tracker that says it has heart rate functionality, then it probably has one of two types of sensors: a PPG (Photoplethysmogram) sensor or a EKG (electrocardiogram) sensor. EKG measures electrical impulses on the skin, usually the chest, using two electrodes to measure the pulses of electricity. PPG measures heart pulses by measuring the changes in color of the blood using an LED light and measuring the reflection. One can verify which sensor their device has as if there is light coming from the sensor, it is probably a PPG sensor, and if there are small metal contacts it is probably a EKG sensor. These each have slightly different information that they can tell you on top of your basic heart pulse rate.
A PPG sensor can tell you a number of interesting things such as SpO2 (Blood oxygen saturation), which is how well your blood is taking up oxygen, and can be very useful in exercise or in understanding . Pi (Perfusion index) is the strength of the pulse at the location of the sensor, this is what gives form to the pleth waveform. PVi (Pleth Variability Index) is the measurement of the variability of the perfusion index, which is currently being looked at as an interesting indicator of how hydrated you are. And RRp (Respiration rate from Pleth) which measures your breathing rate based on an analysis of the pleth waveform, this is because your breathing actually affects the pressures your heart is feeling from your left lung.
An EKG sensor can tell you a lot about the hearts movement on top of just pulse rate, it can especially tell you if there are any issues with your heart, as if the waveform from an EKG shows any out of ordinary patterns it probably means that your heart is doing something out of the ordinary. Generally you wear an EKG sensor around your chest. The problem is that outside of true clinical conditions these changes are hard to check, and verify. Though sensor fusion should definitely help with that in the future. But adding EKG will eventually be one of the most likely sensors to save peoples lives
All of these measurements are extremely valuable and often are the backbone of many of the other sensors, especially as your breathing and pulse themselves affect almost every other sensor in some way. It is often critical to have this data to correlate with other sensor data. A great example is in combination with eye tracking which I talked about before. For those who want a picture of what it might look like from the user side would be something like the “bullet circle” in the anime Sword Art Online as the reticle changes based on your heart rate. Another great use is to tell if someone is excited or scared as an elevated heart rate is good at detecting those, the problem is that being able to understand which one it is (fear or excitement) requires other sensors to be accurate.
Technology: Galvanic Skin Response (GSR) (a form of EDA(Electrodermal activity) sensor
Galvanic Skin Response is a well established technology, and offers unique advantages and drawbacks. In terms of negatives, many GSR devices require 10-20 minute wait time for the sensors to accumulate sweat and be able to measure changes. Additionally, while GSR can measure general levels of emotional intensity, it is not possible to distinguish whether the experience is positive or negative. Lastly, GSR tends to be slow to change and is mainly used to show trends over time. It is possible to measure spikes which denote interesting events, but it can be difficult to isolate what stimulus is driving the overall GSR readings. In terms of advantages, GSR measures emotional state in a fairly unique way. Once correlated with the particular task it can be a reliable indicator of emotional experience.
As GSR is mostly correlated with emotional state, it can allow for another very interesting understanding of your current state. This might sound familiar if you like spy or detective movies or books, as this is one of the pieces of tech that made the polygraph (lie detector) work the way it did. Polygraphs were usually a combination of blood pressure, pulse, respiration and galvanic skin response sensors. And although the stated purpose of use as a lie detector, which can easily be debated, the polygraph was a perfect example of a biosensor fusion device, and thanks to the polygraph, all of the sensors used by it, are quite well documented, as well as how they behave in relation to each other.
Technology: Skin temperature sensor
Skin temperature is another interesting sensor that a few different devices (such as the emotibit, whoop, and Oura ring) have started to use. Skin temperature and body temperature do not always line up, and that can lead to some interesting insights. Also body temperature can be a very good indicator of your immune activity, and overall health. It can also be affected by your emotional state.
Skin temperature can be measured directly by having the sensor touch the skin, which is the typical method used. More advanced temperature sensors use far infrared (FIR) to measure the body state remotely, increasing reliability and reducing the need for another sensor to make contact with the user.
Local skin temperature can change very fast and quite often. Think about when your hands are really hot or really cold. Skin temperature has a direct effect on your internal body temperature and your overall metabolic activity, and body’s hemodynamics, as your skin is in essence your largest organ and has massive amounts of blood flowing through it and muscles right under it. Skin temperature trends are currently being looked into for many different short and long term health metrics related to disease, sickness, recovery period, and exercise.
Technology: humidity / temperature sensor – less Common
When talking about the emotibit, we have to mention that it also has a humidity / temperature sensor that is for measuring skin perspiration, and as another sensor to measure body temperature. Perspiration is interesting because it is currently not something many biosensor devices currently measure, but can have some interesting data. Especially when active, or when you are hot or cold, your perspiration changes quite a bit and can be useful to understand how activities are changing your perspiration amount.
Technology: EEG (electroencephalogram) sensor
Now for the sensor that probably gets the most interest in biosensor fusion and in general when people talk about biosensors is EEG. When people start talking about controlling things with their minds this is often the device people think of.
EEG is a technology that has been around for a while and allows the measurement of brain state through electrical signals. By placing electrodes on the scalp, the system will generate an electrical reading for the brain activity present at that location. By processing that electrical reading, or interpreting it directly, users and researchers can learn about the current brain state. There are a lot of subject specific characteristics with EEG, so it can be hard to generalize – EEG will exhibit consistent readings with the same subjects and the same tasks. EEG will measure significant differences between subjects that are doing the same tasks, and these differences will remain consistent on repeated tests. People’s brain signals are unique to them, and these differences are consistent, almost like a brain fingerprint that is unique for each person.
Most current consumer EEG devices have only a few electrodes, the Muse has 4 electrodes, and the low cost OpenBCI Ganglion also has 4 electrodes. Though this is starting to change as the electronics that are used for amplifying and recording the output of the electrodes are falling in price quite a bit every year, soon consumer devices will have many more electrodes which give much more accurate data, and a better understanding of how the different parts of our brain are working. Soon 8 and 16 will be quite common, and down the line many developers hope that 64 or more will become much less expensive. This increase in the number of channels is important because EEG of different parts of the brain give insight into vastly different parts of what your body is doing. Good examples are that if you do eeg on the back of the head, you can get data that is related to your visual cortex (what you are currently seeing), while the top of the head can give you data related to your motor cortex (how your body moves).
Although EEG is really cool, it has a big issue and that is that on its own it is often not very useful as it becomes very hard to correlate the brainwave data with anything you are doing or thinking at the time, as there is to much data from our body that our brain is processing and it ends up overlapping. And there can be many things that interfere or affect the signal. That also means it is often required to do sensor fusion to get good data from EEG. Or to use AI and machine learning constantly to improve accuracy. When sensor fusion of EEG and almost any other sensor is done you usually can find quite interesting correlation of data.
Technology: fNIRS (Functional Near Infrared Spectroscopy)
An interesting piece of technology that is slowly becoming accessible to consumers is fNIRS (Functional Near Infrared Spectroscopy). This tech is all about imaging blood flow. In some aspects it is similar to an array of PPG sensors, but it has a lot more complexity in how it works so that it can be used as an array and create images. Right now a very common use is to be used for imaging brain blood flow, though it can also be used elsewhere on the body. For sensor fusion this can work alongside EEG quite well to give a better picture of what is currently happening in the brain. Blood Flow through the brain has been shown to be linked with brain activity on multiple occasions. Though sometimes the blood flow is connected with other things then brain activity, such as emotional activity.
A good low cost version of one of these devices is the HEGduino, but it can not really create images as it is only a single sensor (basically an advanced PPG sensor device). If you have 100k burning a hole in your wallet, you can buy 50 sensors to fill up a whole Kernel flow device. And if you have university backing there are many more options like the Kernel Flux.
Technology: ultrasound imaging sensor
Another type of imaging that is getting easier to access is ultrasound imaging. There has been a lot of recent research into using ultrasound in combination with other biosensors. Especially for brain imaging as (TCD (Transcranial doppler Ultrasound)), it gives a much deeper image then fNIRS, but the technology is a bit less portable at the moment and often requires a large amount of area for the sensor, that could instead fit a large number of other sensors.
Technology: EIT (electrical impedance tomography) sensor
Though out of all imaging technologies probably my favorite up and coming one has to be EIT. Although in its early stages, this real time medical imaging technology has a lot of potential, especially as it has fewer downsides then MRI (bad temporal resolution, big device), CT scans (radiation), and ultrasound (currently hard to wear). It also has great temporal resolution. The problem is that it currently lacks spatial resolution. A really cool use case for this is to do cross sectional analysis of a part of your body while you are doing an activity. If you do a cross section of your wrist you could figure out quite accurately the position of your fingers. This might even have better resolution and accuracy then using EMG, or Myoelectric sensors which will be talked about later in this article.
Technology: CT scans and MRI
Now a bit more on the absurd and excessive side, I do have to mention that CT scans and MRI can be very helpful for sensor fusion even if they can’t be done in real time. This is because each person’s body is different enough that bone and body structure can have noticeable impact on the accuracy of different sensors. So having an MRI or CT scan can allow for you to tune sensors to be more accurate to your body. This is extremely helpful for any form of brain related sensor fNIRS, EEG, etc, as the shape of your skull, and brain’s placement inside your skull does affect the signal that the sensor is able to receive quite a bit. The hardest part about getting an MRI other than cost itself, is that for use with EEG, you actually want to get a standing MRI scan so that it accurately places your brain in your skull in the same way that it would be when you are active in daily life. This is because your brain is in a different spot in your skull when you are lying down vs sitting or standing.
Technology: environmental sensors
Another set of sensors that have only recently been adopted into biosensor fusion, are environmental sensors to track the state of the environment around the user. These sensors are useful in understanding how our body reacts to a changing environment. Although these sensors are not in very many devices yet, they are some of the easiest to add yourself, because many of these sensors are quite cheap and easy to get ahold of. You might even be able to use the temperature and humidity sensors on the emotibit this way if you have an extra and flip it around to have the sensors not on the skin.
Technology: Full body tracking
A somewhat common biosensor that people might have used is body motion tracking. Although this is not always considered a biosensor itself, it is tracking your physical body in a way that other sensors can’t. And provides very important information when you connect it to other biosensors, since body movement can affect a lot of different aspects of your body, including, heart rate, brain activity, metabolism, skin temperature, etc. If you have ever used a recent gen VR headset then you have experienced at least a bit of this through controller and headset tracking which ends up tracking your hand position and head position. If you have used oculus or windows mixed reality headsets, you have used camera + IMU based tracking, if you have used a steamvr tracked headset then you have used a lidar + IMU tracked headset.
In the near future full body tracking will become much more common outside of the motion tracking they use in movies. A big part of this has been the improvement in two things, camera based movement analysis, and IMU drift. It was not very long ago that identifying how our body is moving in three dimensions from just 2D camera images was considered really difficult, but thanks to AI and machine learning we can now quite accurately understand the position and movement of a person’s body from a single or very few cameras. And then there is IMU drift, up until about 5 years ago using an IMU based motion capture suit was considered an unreliable option, and this is because after a very short period of time, the sensor readings would drift out of alignment with the actual position that your body was in. And if you have a suit with 20 or 30 IMU sensors, the drift would make you need to recalibrate the suit position very often. Each type has some very good use cases, as cameras do not need to be worn on the body, but IMU tracking suits can be used while out in the everyday world.
Technology: EMG (Electromyography) and Myoelectric sensors
http://www.advancertechnologies.com/p/myoware.htmlhttps://www.sparkfun.com/products/13723
When talking about body movement it is also important to consider why exactly our body is moving in the first place, and a great sensor for doing that is contact based Myoelectric sensors, which measure electrical impulses that trigger your muscle to expand or contract using a pair of electrodes.
There are three different focuses for detecting things with EMG usually. First is detection of The motor neurons firing, which sends very quick spike signals to the muscle. This is quite difficult depending on the size of the muscle, and if it is hard to reach without needle based EMG sensors. This can be used to predetermine if you are going to move a part of your body. An example of a device focused around using this quick spike is the Brink Bionics Impulse Neurocontroller. Second is the detection of a muscle twitch which will happen from the firing of the motor neurons. Good examples of this sensor in use are the Myo armband, and the armband Meta (Facebook) has recently shown, that is based off the hardware they got when acquiring Ctrl Labs. And third is monitoring of tetanic muscle activation from continued motor neurons firing. This can be great for helping figure out how much power, or force you are putting into any one movement during something like full body tracking or while doing sports, or how fatigued your muscles are after a workout.
Another interesting use case is to map facial position, by having the sensors on the face, the Galea will have EMG, it will only be for the face.
As we can see, the ability to measure people has grown to a capacity that was never possible before. We can passively observe what someone finds interesting in a given scene or encounter, measure the brain state that experience generates, and record the intensity and enjoyability of the experience. This will allow quantification of many things that are currently invisible to us. Imagine a couple recording their biometric responses to various TV shows, so that they can make better decisions on what to watch together based on how enjoyable it will be for both parties. Lecturers will be able to monitor student volunteers during presentations to see how it makes the students feel and tune their material accordingly. Car manufacturers will be able to passively observe driver fatigue and attention and design safer cars that notify the driver when their attention is lacking or needed to deal with an urgent issue. Companies will be able to design easier to use and understand packaging based on consumer response. A doctor could observe a patient’s reactions to their explanation of some diagnosis, note a place where confusion occurs, and revisit that aspect. All of these will finally allow us to get past the current difficulties presented by placebo, self report, and an inability to quantify and compare people’s experiences.
On a fundamental level, something worth noting is that many people don’t really trust or believe that people are having the experience they claim to have, as much of the time we don’t have a way to quantify or verify whether this is the case. If someone says they are scared, or angry, or happy, or frustrated, or confused – there’s often an element of doubt present as the only information available is what the person claims is happening to them. If someone says they enjoyed a movie, we might assume they are praising the movie for some other reason other than liking the film. If someone says they enjoyed a meal, perhaps they enjoyed the company and social setting but didn’t enjoy the food one bit. With sensor data, we’d be confident in what they were saying – or they could volunteer to share the data with others – which has profound implications for our society and the way we see each other.
These sensors will provide a wealth of data about us, our lives, and our patterns. We will need strong laws and motivated legislatures to ensure the protections necessary to make these sensors safe and ubiquitous.
Let us know your thoughts! Sign up for a Mindplex account now, join our Telegram, or follow us on Twitter.
The United Nations and our uncertain future: breakdown or breakthrough?
“Humanity faces a stark and urgent choice: a breakdown or a breakthrough”
These words introduce an 85-page report issued by António Guterres, United Nations Secretary-General (UNSG).
The report had been produced in response to requests from UN member state delegations “to look ahead to the next 25 years” and review the issues and opportunities for global cooperation over that time period.
Entitled “Our Common Agenda,” the document was released on September 10, 2021.
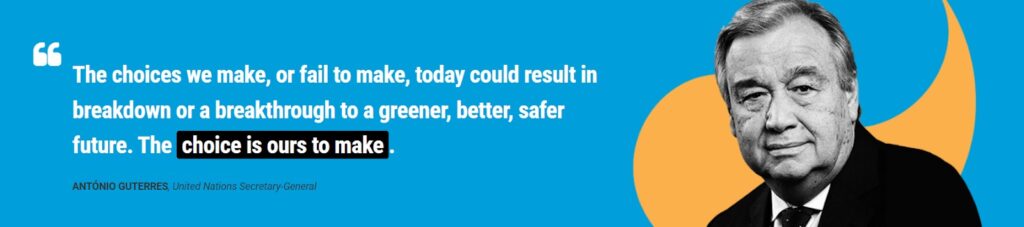
It featured some bold recommendations. Two examples:
Now is the time to end the “infodemic” plaguing our world by defending a common, empirically backed consensus around facts, science and knowledge. The “war on science” must end. All policy and budget decisions should be backed by science and expertise, and I am calling for a global code of conduct that promotes integrity in public information.
Now is the time to correct a glaring blind spot in how we measure economic prosperity and progress. When profits come at the expense of people and our planet, we are left with an incomplete picture of the true cost of economic growth. As currently measured, gross domestic product (GDP) fails to capture the human and environmental destruction of some business activities. I call for new measures to complement GDP, so that people can gain a full understanding of the impacts of business activities and how we can and must do better to support people and our planet.
It also called for greater attention on being prepared for hard-to-predict future developments:
We also need to be better prepared to prevent and respond to major global risks. It will be important for the United Nations to issue a Strategic Foresight and Global Risk Report on a regular basis…
I propose a Summit of the Future to forge a new global consensus on what our future should look like, and what we can do today to secure it.
But this was only the start of a serious conversation about taking better steps in anticipation of future developments. It’s what happened next that moved the conversation significantly forward.
Introducing the Millennium Project
Too many documents in this world appear to be “write-only.” Writers spend significant time devising recommendations, documenting the context, and converting their ideas into eye-catching layouts. But then their report languishes, accumulating dust. Officials may occasionally glance at the headlines, but the remainder of the fine words in the document could be invisible for all the effect they have in the real world.
In the case of the report “Our Common Agenda,” the UNSG took action to avoid the tumbleweed scenario. His office contacted an organization called the Millennium Project to collect feedback from the international futurist community on the recommendations in the report. How did these futurists assess the various recommendations? And what advice would they give regarding practical steps forward?
The Millennium Project has a long association with the United Nations. Established in 1996 after a three-year feasibility study with the United Nations University, it has built up a global network of “nodes” connected to numerous scholars and practitioners of foresight. The Millennium Project regularly publishes its own “State of the Future” reports, which aggregate and distill input from its worldwide family of futurists.
A distinguishing feature of how the Millennium Project operates is the “Real-Time Delphi” process it uses. In a traditional questionnaire, each participant gives their own answers, along with explanations of their choices. In a Delphi survey, participants can see an anonymized version of the analysis provided by other participants, and are encouraged to take that into account in their own answers.
So participants can reflect, not only on the questions, but also on everything written by the other respondents. Participants revisit the set of questions as many times as they like, reviewing any updates in the input provided by other respondents. And if they judge it appropriate, they can amend their own answers, again and again.
The magic of this process — in which I have personally participated on several occasions — is the way new themes, introduced by diverse participants, prompt individuals to consider matters from multiple perspectives. Rather than some mediocre “lowest common denominator” compromise, a novel synthesis of divergent viewpoints can emerge.
So the Millennium Project was well placed to respond to the challenge issued by the UNSG’s office. And in May last year, an email popped into my inbox. It was an invitation to me to take part in a Real-Time Delphi survey on key elements of the UNSG’s “Our Common Agenda” report.
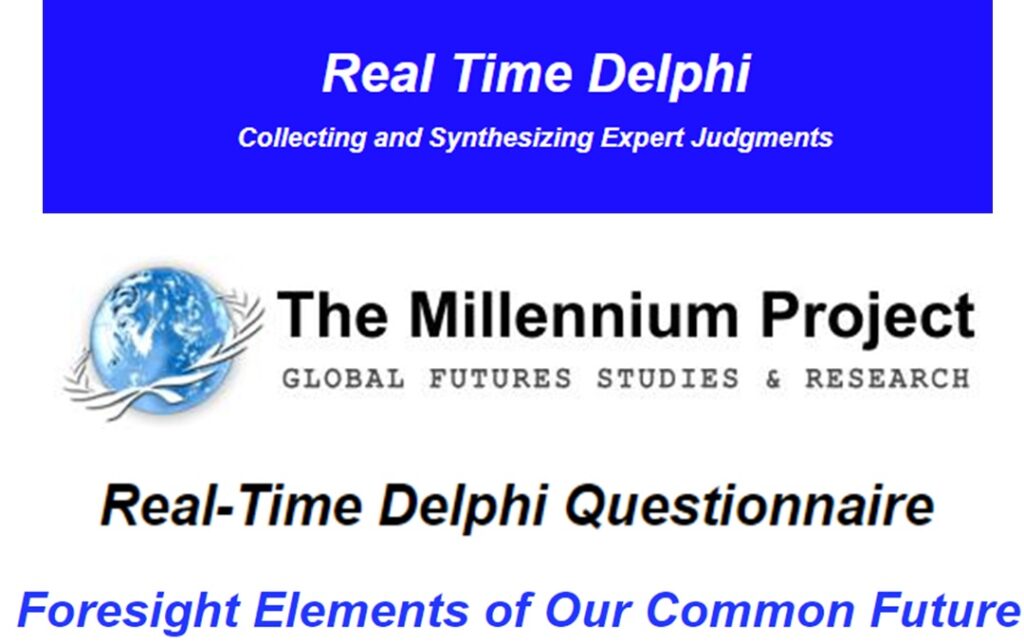
In turn, I forwarded the invitation in a newsletter to members of the London Futurists community that I chair. I added a few of my own comments:
The UNSG report leaves me with mixed feelings.
Some parts are bland, and could be considered “virtue signaling.”
But other parts offer genuinely interesting suggestions…
Other parts are significant by what is not said, alas.
Not everyone who started answering the questionnaire finished the process. It required significant thought and attention. But by the time the Delphi closed, it contained substantive answers from 189 futurists and related experts from a total of 54 countries.
The results of the Delphi process
Researchers at the Millennium Project, led by the organization’s long-time Executive Director, Jerome Glenn, transformed the results of the Delphi process into a 38-page analysis (PDF). The process had led to two types of conclusion.
The first conclusions were direct responses to specific proposals in the “Our Common Agenda” report. The second referred to matters that were given little or no attention in the report, but which deserve to be prioritized more highly.
In the first category, responses referred to five features of the UNSG proposal:
-
- A Summit of the Future
- Repurposing the UN Trusteeship Council as a Multi-Stakeholder Body
- Establishing a UN Futures Lab
- Issuing Strategic Foresight and Global Risk Reports on a regular basis
- Creating a Special Envoy for Future Generations
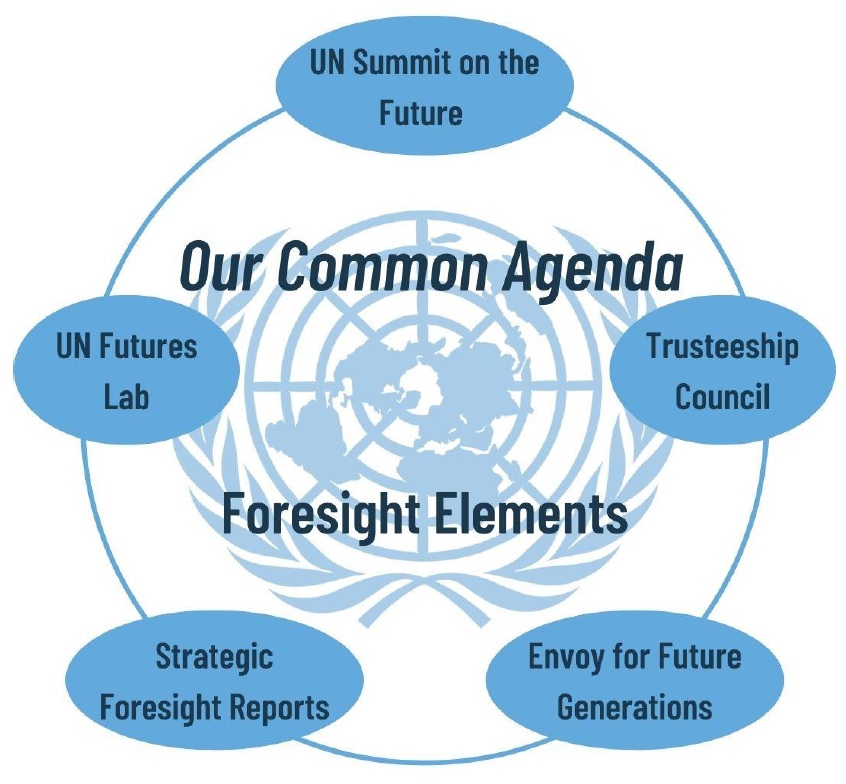
Overall, the Delphi gave a strong positive assessment of these five proposals. Here’s a quote from Jerome Glenn:
If the five foresight elements in Our Common Agenda are implemented along the lines of our study, it could be the greatest advance for futures research and foresight in history… This is the best opportunity to get global foresight structural change into the UN system that has ever been proposed.
Of the five proposals, the UN Futures Lab was identified as being most critical:
The UN Futures Lab was rated the most critical element among the five for improving global foresight by over half of the Real-Time Delphi panel. It is critical, urgent, and essential to do it as soon as possible. It is critical for all the other foresight elements in Our Common Agenda.
The Lab should function across all UN agencies and integrate all UN data and intelligence, creating a global collective intelligence system. This would create an official space for systemic and systematic global futures research. It could become the foresight brain of humanity.
The Delphi also deemed the proposal on strategic foresight reports particularly important:
Strategic Foresight and Global Risk Reports were seen as very critical for improving global foresight by nearly 40% of the panel. This is exactly the kind of report that the United Nations should give the world. Along with its own analysis, these reports should provide an analysis and synthesis of all the other major foresight and risk reports, provide roadmaps for global strategies, and give equal weight to risks and opportunities.
The reports should be issued every one or two years due to accelerating change, and the need to keep people involved with these issues. It should include a chapter on actions taken since the last report, with examples of risk mitigation, management, and what persists…
They should bring attention to threats that are often ignored with cost estimates for prevention vs. recovery (Bill Gates estimates it will cost $1 billion to address the next pandemic compared to the $15 trillion spent on Covid so far). It should identify time-sensitive information required to make more intelligent decisions.
In a way, it’s not a big concern that key futurist topics are omitted from Our Common Agenda. If the mechanisms described above are put in place, any significant omissions that are known to foresight practitioners around the world will quickly be fed into the revitalized process, and brought to wider attention.
But, for the record, it’s important to note some key risks and opportunities that are missing from the UNSG’s report.
The biggest forthcoming transition
Jerome Glenn puts it well:
If we don’t get the initial conditions right for artificial general intelligence, an artificial superintelligence could emerge from beyond our control and understanding, and not to our liking.
If AGI could occur in ten to 20 years and if it will take that same amount of time to create international agreements about the right initial conditions, design a global governance system, and implement it, we should begin now.
Glenn goes on to point out the essential international nature of this conundrum:
If both the US and China do everything right, but others do not, the fears of science fiction could occur. It has to be a global agreement enforced globally. Only the UN can do that.
Here’s the problem: Each new generation of AI makes it easier for more people to become involved in developing the next generation of AI. Systems are often built by bolting together previous components, and tweaking the connections that govern the flow of information and command. If an initial attempt doesn’t work, engineers may reverse some connections and try again. Or add in some delay, or some data transformation, in between two ports. Or double the amount of processing power available. And so on. (I’m over-simplifying, of course. In reality, the sorts of experimental changes made are more complex than what I’ve just said.)
This kind of innovation by repeated experimentation has frequently produced outstanding results in the history of the development of technology. Creative engineers were frequently disappointed by their results to start with, until, almost by chance, they stumbled on a configuration that worked. Bingo — a new level of intelligence is created. And the engineers, previously suspected as being second-rate, are now lauded as visionary giants.
Silicon Valley has a name for this: Move fast and break things.
The idea: if a company is being too careful, it will fail to come up with new breakthrough combinations as quickly as its competitors. So it will go out of business.
That phrase was the informal mission statement for many years at Facebook.
Consider also the advice you’ll often hear about the importance of “failing forward” and “how to fail faster.”
In this view, failures aren’t a problem, provided we pick ourselves up quickly, learn from the experience, and can proceed more wisely to the next attempt.
But wait: what if the failure is a problem? What if a combination of new technology turns out to have cataclysmic consequences? That risk is posed by several leading edge technologies today:
-
- Manipulation of viruses, to explore options for creating vaccines to counter new viruses — but what if a deadly new synthetic virus were to leak out of supposedly secure laboratory confinement?
- Manipulation of the stratosphere, to reflect back a larger portion of incoming sunlight — but what if such an intervention has unexpected side effects, such as triggering huge droughts and/or floods?
- Manipulation of algorithms, to increase their ability to influence human behavior (as consumers, electors, or whatever) — but what if a new algorithm was so powerful that it inadvertently shatters important social communities?
That takes us back to the message at the start of this article, by UNSG António Guterres: What if an attempt to achieve a decisive breakthrough results, instead, in a terrible breakdown?
Not the Terminator
The idea of powerful algorithms going awry is often dismissed with a wave of the hand: “This is just science fiction.”
But Jerome Glenn is correct in his statement (quoted earlier): “The fears of science fiction could occur.”
After all, HG Wells published a science-fiction story in 1914 entitled The World Set Free that featured what he called “atomic bombs” that derived their explosive power from nuclear fission. In his novel, atomic bombs destroyed the majority of the world’s cities in a global war (set in 1958).
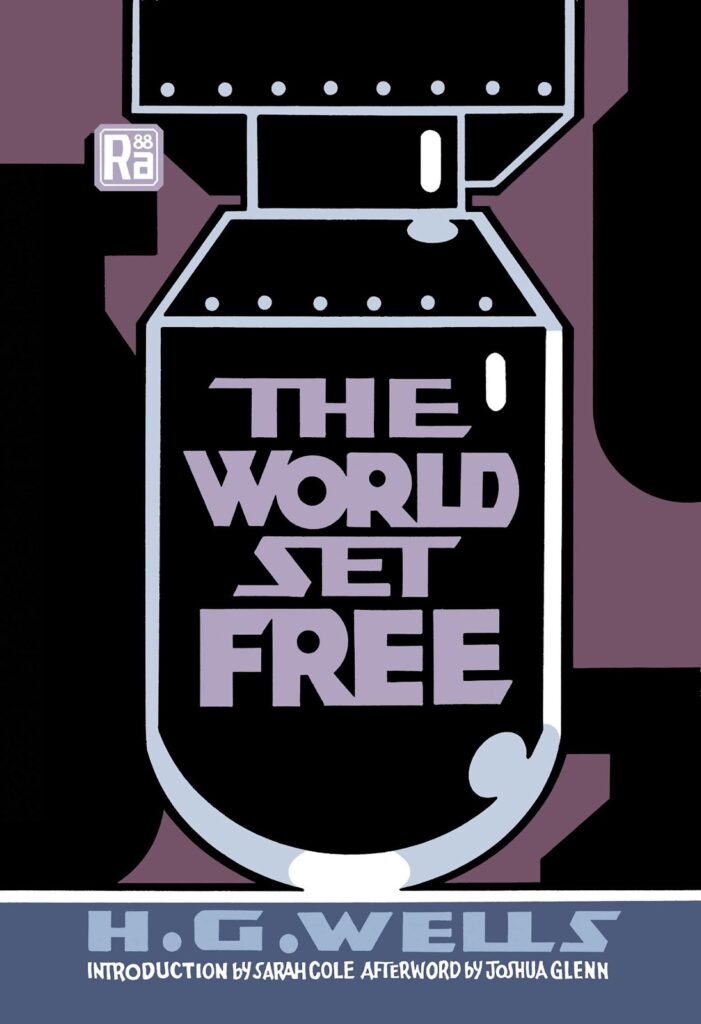
But merely because something is predicted in science fiction, that’s no reason to reject the possibility of something like it happening in reality.
The “atomic bombs” foreseen by HG Wells, unsurprisingly, differed in several ways from the real-world atomic bombs developed by the Manhattan Project and subsequent research programs. In the same way, the threats from misconfigured powerful AI are generally different from those portrayed in science fiction.
For example, in the Hollywood Terminator movie series, humans are able, via what can be called superhuman effort, to thwart the intentions of the malign “Skynet” artificial intelligence system.
It’s gripping entertainment. But the narrative in these movies distorts credible scenarios of the dangers posed by AGI. We need to avoid being misled by such narratives.
First, there’s an implication in The Terminator, and in many other works of science fiction, that the danger point for humanity is when AI systems somehow “wake up,” or become conscious. If true, a sufficient safety measure would be to avoid any such artificial consciousness.
However, a cruise missile that is hunting us down does not depend for its deadliness on any cruise-missile consciousness. A social media algorithm that is whipping up hatred against specific ethnic minorities isn’t consciously evil. The damage results from the cold operation of algorithms. There’s no need to involve consciousness.
Second, there’s an implication that AI needs to be deliberately malicious before it can cause damage to humans. However, damage to human wellbeing can, just as likely, arise from side effects of policies that have no malicious intent.
When we humans destroy ant colonies in our process of constructing a new shopping mall, we’re not acting out of deliberate malice toward ants. It’s just that the ants are in our way. They are using resources for which we have a different purpose in mind. It could well be the same with an AGI that is pursuing its own objectives.
Consider a corporation that is vigorously pursuing an objective of raising its own profits. It may well take actions that damage the wellbeing of at least some humans, or parts of the environment. These outcomes are side-effects of the prime profit-generation directive that is governing these corporations. They’re not outcomes that the corporation consciously desires. It could well be the same with a badly designed AGI.
Third, the scenario in The Terminator leaves viewers with a false hope that, with sufficient effort, a group of human resistance fighters will be able to out-maneuver an AGI. That would be like a group of chimpanzees imagining that, with enough effort, they could displace humans as the dominant species on planet Earth. In real life, bullets shot by a terminator robot would never miss. Resistance would indeed be futile.
Instead, the time to fight against the damage an AGI could cause is before the AGI is created, not when it already exists and is effectively all-powerful. That’s why any analysis of future global developments needs to place the AGI risk front and center.
Four catastrophic error modes
The real risk — as opposed to “the Hollywood risk” — is that an AI system may acquire so much influence over human society and our surrounding environment that a mistake in that system could cataclysmically reduce human wellbeing all over the world. Billions of lives could be extinguished, or turned into a very pale reflection of their present state.
Such an outcome could arise in any of four ways – four catastrophic error modes. In brief, these are:
-
- Implementation defect
- Design defect
- Design overridden
- Implementation overridden.
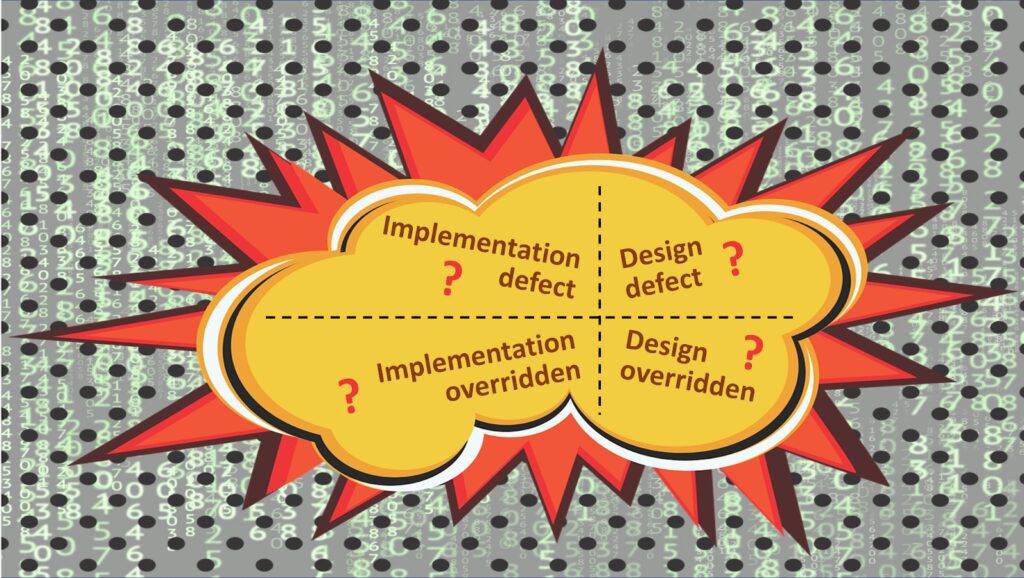
In more detail:
- The system contains a defect in its implementation. It takes an action that it calculates will have one outcome, but unexpectedly, it has another outcome instead. For example, a geoengineering intervention could trigger an unforeseen change in the global climate, plunging the Earth into a state in which humans cannot survive.
- Imagine, for example, an AI with a clumsily specified goal to focus on preserving the diversity of the Earth’s biosystem. That could be met by eliminating upward of 99% of all humans. Oops! Such a system contains a defect in its design. It takes actions to advance the goals it has explicitly been given, but does so in a way that catastrophically reduces actual human wellbeing.
- The system has been given goals that are well aligned with human wellbeing, but as the system evolves, a different set of goals emerge, in which the wellbeing of humans is deprioritized. This is similar to how the emergence of higher thinking capabilities in human primates led to many humans taking actions in opposition to the gene-spreading instincts placed into our biology by evolution.
- The system has been given goals that are well-aligned with human wellbeing, but the system is reconfigured by hackers of one sort or another — perhaps from malevolence, or perhaps from a misguided sense that various changes would make the system more powerful (and hence more valuable).
Some writers suggest that it will be relatively easy to avoid these four catastrophic error modes. I disagree. I consider that to be wishful thinking. Such thinking is especially dangerous, since it leads to complacency.
Wise governance of the transition to AGI
Various “easy fixes” may work against one or two of the above catastrophic error modes, but solutions that will cope with all four of them require a much more comprehensive approach: something I call “the whole-system perspective.”
That whole-system perspective includes promoting characteristics such as transparency, resilience, verification, vigilance, agility, accountability, consensus, and diversity. It champions the discipline of proactive risk management.
It comes down to a question of how technology is harnessed — how it is selectively steered, slowed down, and (yes) on occasion, given full throttle.
Taking back control
In summary, it’s necessary to “take back control of technology.” Technology cannot be left to its own devices. Nor to the sometimes headlong rushes of technology corporations. Nor to the decisions of military planners. And definitely not to the whims of out-of-touch politicians.
Instead, we — we the people — need to “take back control.”
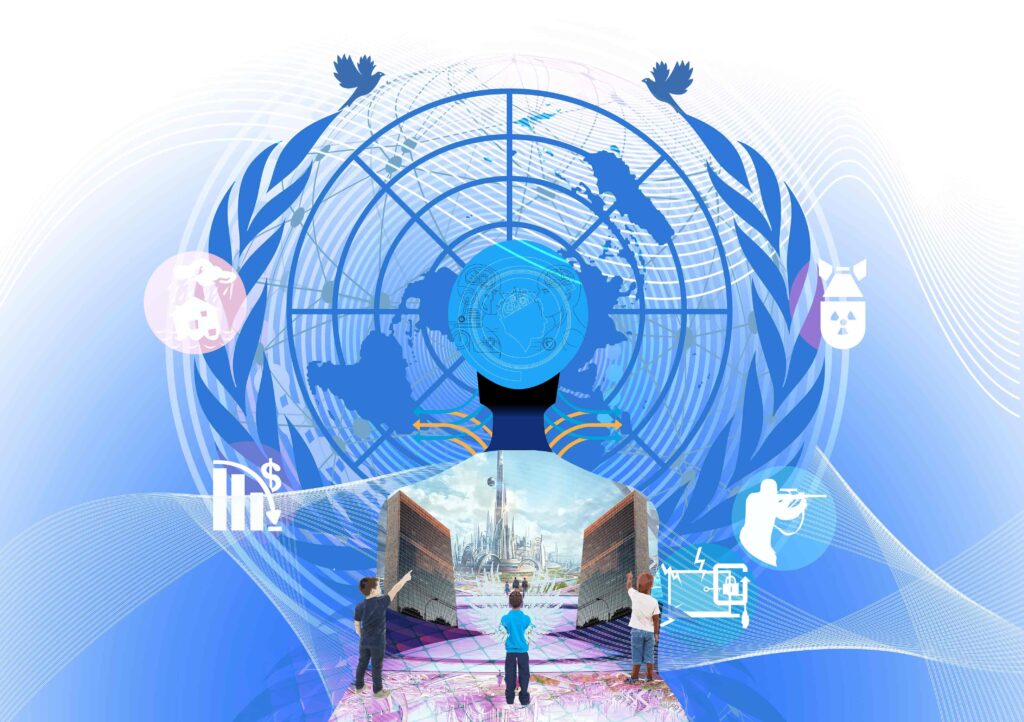
There’s a huge potential role for the United Nations in wise governance of the transition to AGI. The UN can help to broker and deepen human links at all levels of society, despite the sharp differences between the various national governments in the world. An understanding of “our common agenda” regarding fast-changing technologies (such as AI) can transcend the differences in our ideologies, politics, religions, and cultures.
I particularly look forward to a worldwide shared appreciation of:
-
- The risks of catastrophe from mismanaged technological change
- The profound positive possibilities if these technologies are wisely managed.
Let us know your thoughts! Sign up for a Mindplex account now, join our Telegram, or follow us on Twitter.
The World of Sensors
The Fitbit is the first wearable biosensor that achieved widespread adoption, due to a simple proposition: measure the amount of steps that a person takes in a day. This measurement turns out to be an excellent approximation for fitness level and provides a clear goal to measure against: 10,000 steps a day. How does it measure the steps? The accelerometer measures the movement of the Fitbit and can determine your motion.
Here is an example of an accelerometer recording someone walking:
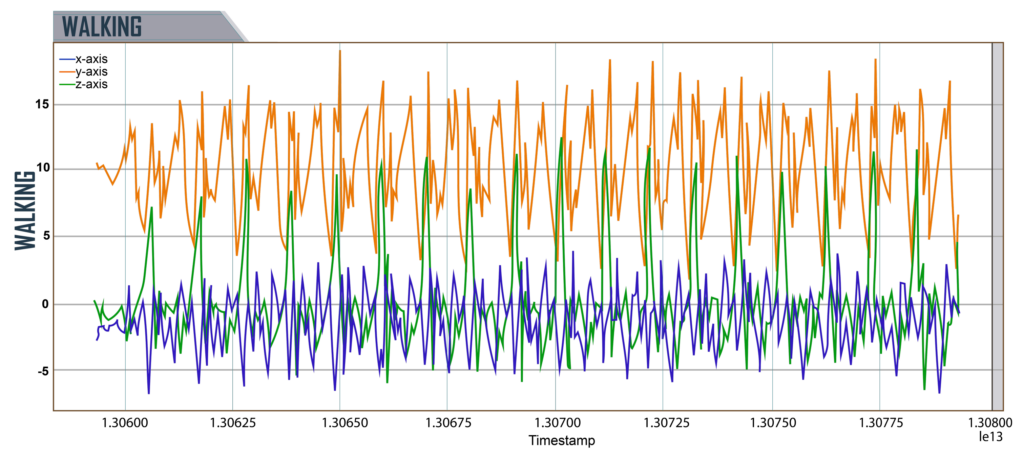
Here is an example of someone jogging – note how different the data looks
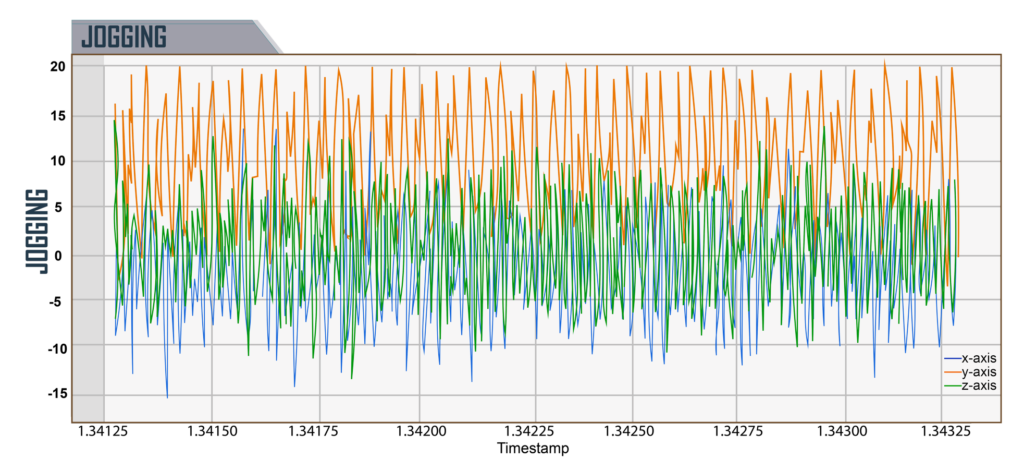
It can tell when you are navigating a flight of stairs, and can tell which way you’re going – climbing up the stairs:
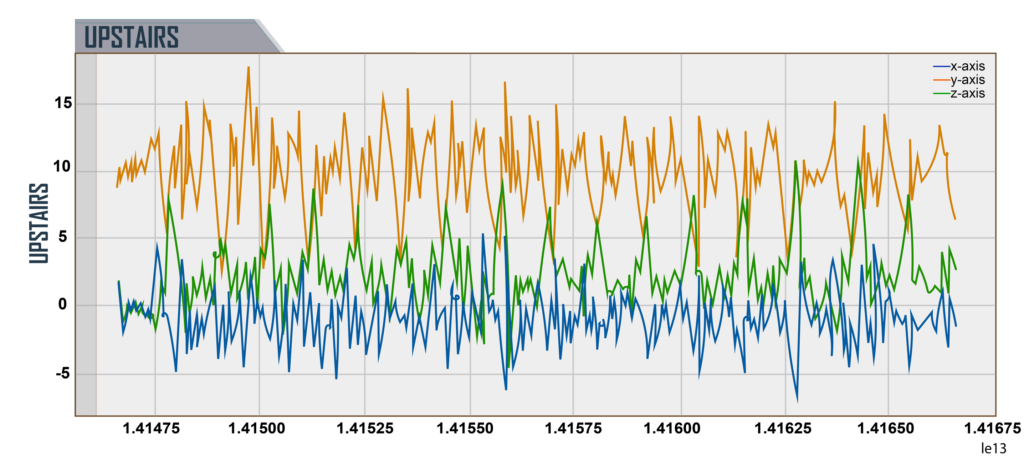
Climbing down the stairs:
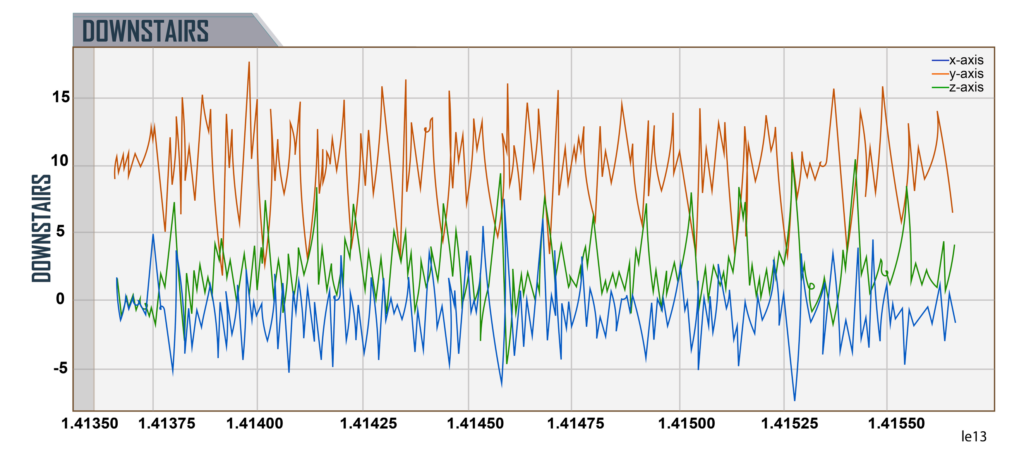
Sitting down:
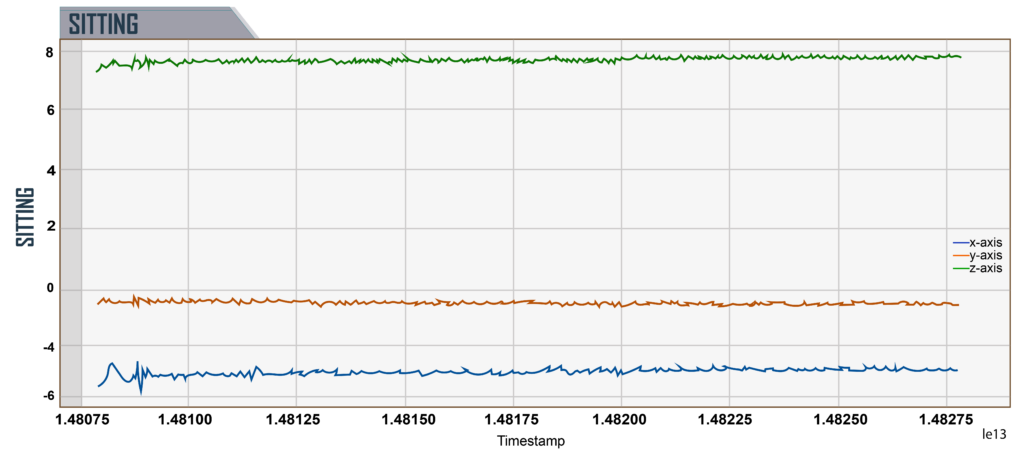
Standing up:
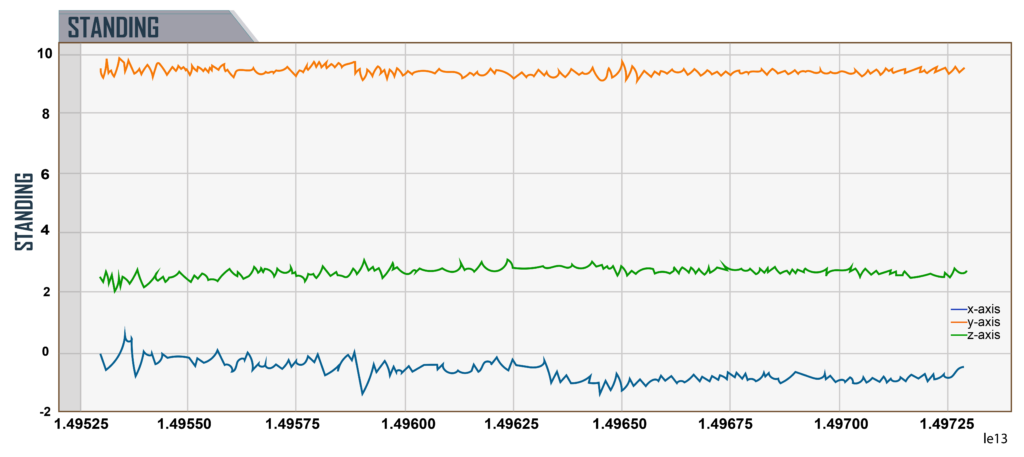
Even with just the accelerometer data above we have a pretty good idea of what the user is doing, but we can go much further. The Fitbit is a combination of sensors working together to paint a richer picture than each one could on its own. It combines an accelerometer, a gyroscope, an altimeter, a GPS, a heart rate sensor, electrical sensors that can measure skin conductivity, skin temperature, ambient light, and a microphone. These are all fairly low cost, straightforward sensors – and their applications are well known and have been used for decades.
Let’s look at just two of these sensors and how they work together. The first sensor is the accelerometer, which measures the motion of the device on three axes. From the motion readings generated, the user’s activity and motion can be identified: whether they are sitting, standing, walking, jogging, or navigating a set of stairs.
Each type of movement generates a distinct set of readings, so it’s possible to infer state from something as imprecise and simple as a device accelerometer. Correlating just this single sensor with a bit more information can be quite powerful – if a user’s phone tends to show consistent motion until 11pm at night, at which point it remains completely stationary for 7-8 hours and starts moving again in the morning, we can be reasonably confident that this is when the user is sleeping, despite not being able to measure it directly.
Let’s combine the accelerometer with another sensor that measures heart rate. Now we can not only measure the amount of motion that the user is engaging in, but the amount of effort that motion requires. Let’s ask a series of questions and consider each sensor, and what we get from them by themselves, compared to where we can get by combining them.
Using the data from these sensors:
Are you walking up a hill?
An accelerometer alone can answer this question, because it measures motion state and can infer things like how quickly you are walking, climbing, or running.
A heart rate sensor only measures your heart rate, so it can’t tell you what activity is actually causing your heart rate to go up or down.
Are you expending significant effort to walk up the hill?
The accelerometer can “know” that you are walking, but it cannot tell you how much effort you are expending.
The heart rate sensor can answer how much effort you are expending in any given moment, but it does not allow us to determine activity type very easily.
But when used together, we can show heart rate increase is being caused from climbing the hill. After the accelerometer shows the climbing has stopped, the heart rate monitor can measure the amount of time it takes the heart rate to return to normal. So using both sensors together helps form a much richer and more comprehensive picture than the accelerometer or heart rate monitor alone.
Let’s consider one more case, where we have the Fitbit’s GPS information. If we combine this information now, we can determine motion type from the accelerometer, level of effort from the heart rate meter, and location change from the GPS. Now we can know what hill the person climbed, when, and exactly how much effort the person expended. A hiker with a GPS alone could provide some of this information, but as we can see above, adding low cost sensors and correlating them has a powerful additive effect.
Combining multiple types of measurements and correlating them is something most of us are familiar with. When we go to the doctor’s office for an annual exam, there’s a series of tests that we will undergo. Our height and weight are recorded. Our skin temperature is measured, along with our heart rate and blood pressure. This provides a baseline for comparison with any changes we experience over time. These measurements are correlated and used to determine whether any unexpected shifts are happening. It’s by merging these measurements together that we can form a rich picture of our physical health and be confident in our analysis.
A recent evolution of these fitness trackers is the Whoop band, a competitor to Fitbit. It measures similar things like sleep, heart rate, and motion – with a more targeted goal of delivering feedback to the athlete on their current training readiness.
The Whoop band measures Heart Rate Variability as an indicator of physical strain and recovery from physical strain. Heart Rate Variability (HRV) is a metric that looks at the amount of variation in the intervals between a person’s heart beats. High Heart Rate Variability is considered desirable, and is a measure of physical recovery and/or stress.
By combining these low-cost sensors, the platform is able to calculate remarkably complex things about the wearer. It has enabled people to understand their bodies in new ways, from their sleep quality to the level of strain they experience while doing various exercises. After the workout the heart rate will rise and get more steady – both signs that the body is recovering from the strain. From the amount of change in heart rate, the app can determine where the user is in their recovery, and indicate whether to continue resting, engage in light exercise, or to work out at full intensity.
We are now at a point where a $200 device can passively measure things about your fitness and derive insights that ten years ago would have only been available to professional athletes. An array of simple, low cost sensors in a consumer device can provide the information people need to make effective and safe training decisions. Fitbit and Whoop showed how combining multiple sensors gave users new insights into their physical health; in the future, it will be possible to combine various other sensors to give users insights into their cognitive health.
So when talking about neuroscience and the coming revolution in our ability to measure ourselves, our internal states, and our body, what sensors are involved with that? It starts with the same biometric sensors we’re using currently in Fitbits and Apple Watches – skin temperature, heart rate, motion tracking. To that we add a few more that have been used extensively in research but are recently arriving in the consumer/low-cost sensor space, namely eye tracking, electroencephalography (EEG), and galvanic skin response.
Eye Tracking
With an eye tracking sensor bar or a VR headset, this sensor can tell where you are looking. Depending on the sensor, it will also be able to determine the size of your pupils – these expand and constrict with changes in “cognitive load.” (Note that pupil size can also mean other things, such as a reaction to brightness or outright fear.)
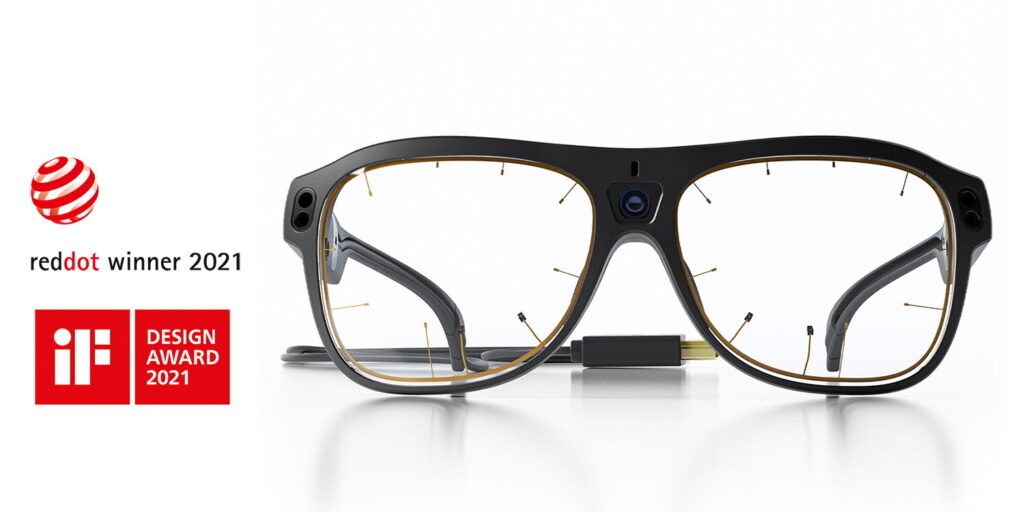
Cognitive Load is the concept that the brain has finite resources and will allocate them as needed – in the same way that your heart and lungs have physical limits on their performance. Pupil size, which can be measured from an eye tracker, is an excellent measurement of cognitive load. Thus, an eye tracker knows what you are looking at, and can also determine how intensely you are processing that object.
What can we do with this information? Imagine you’re a consumer goods company that is about to purchase a 30 second superbowl ad for $6 million. The commercials cost roughly $300,000 to produce. Why not produce three and find the one that consumers respond to the most? With an eye tracker, have some consumers look at the different ad versions. From that, run whatever commercial causes people to look at the product the most times, and spend the most time lingering on the product.
What doesn’t the eye tracker tell us? Anything else, really. How the person feels about the product, what brain state it evokes, how intensely they feel that way. What if they’re staring at it because they really dislike it? While that is engagement, it is perhaps not the kind of engagement wanted. While the eye tracker has an amazing ability to tell us what someone is looking at and how hard their brain is working, it won’t give us a complete picture. It’s accurate but partial.
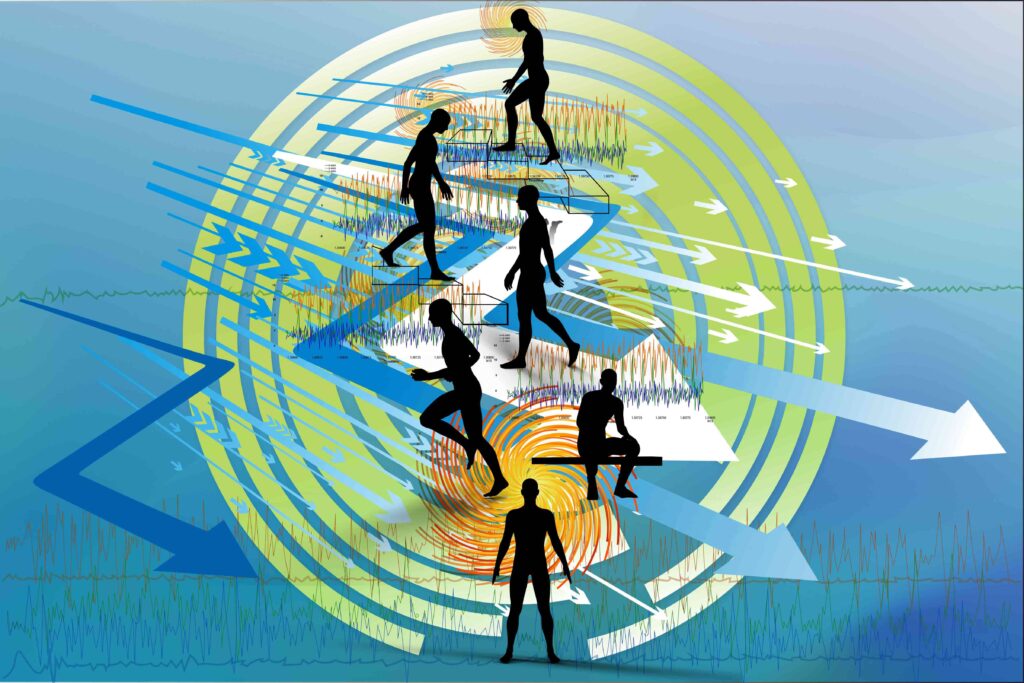
Galvanic Skin Response (GSR)
Skin conductivity is responsive to emotional state. As an emotional experience gets more intense, a person’s skin conducts electricity better. It can be hard to directly measure whether that emotional response is positive or negative (though that can often be easily guessed).
If we were to run a study with the eye tracker alone, we could use the metrics it provides to get a good understanding of which products seem to appeal to consumers visually. We can also gain an understanding of how people engage with products they find visually appealing, by quantifying the difference in fixation order, fixation length, and gaze sequence between the products. Using pupil size, we can infer how much work their brain did processing the object they were looking at.
We cannot make statements about how the person felt emotionally about the object they were looking at using only the eye tracker.
However, if we were to run a study with the GSR meter alone, we could use the metrics it provides to get a good understanding of the emotional response people have, say, to a commercial. We don’t have a good way to distinguish which products generate which response, though we can partially infer it from looking at the way the GSR response changes during the 30 second commercial and whether the three commercials drive fundamentally different responses. Basically, we know how they feel, but we don’t know what they’re looking at, as they have that emotional response.
Now, if we combine these two sensors, there will be some initial difficulty reconciling this data. Eye trackers and GSR meters operate at different sample rates, but also at different timescales. These various physiological processes have different response times and different ways of presenting. 30 seconds is a lot of data for an eye tracker, but a small amount for a GSR meter. Combining sensors often starts with revisiting the experimental design to work with the strengths of both sensors. In this case, 30 seconds is still sufficient for doing this comparison, so we can proceed.
We need a new interpretation that includes both sensors. When we were just looking at the eye tracking, we defined “this person favors the desired object” as the success criteria. When we were just looking at the GSR, we defined “this person has the strongest emotional response” as the success criteria. Now we’re going to define “This person favors the desired object while having the strongest emotional response” as the success criteria.
From the eye tracker, we know what object a person is observing at any given moment. From the GSR meter, we know the intensity of the response. Now we can merge the two metrics, and see how much they agree with each other. If we find that the object the person looks at the most also generates the strongest emotional response, it would provide a very clear signal that they are interested in that object.
What if we find that there isn’t a clear relationship between the amount of time spent looking at an object and the emotional response? In that case, we would need to come up with a ‘tie breaker’ to resolve the contradiction. We could use another sensor – by adding facial expression tracking to the mix, we would have a clear idea of whether the emotion was pleasurable or not, and resolve inconsistencies between the eye tracker and GSR meter.
In some cases, we will not be able to come up with a clear relationship between the sensors, even if they are painting complimentary pictures of the data. In other cases, we will be able to come up with a clear relationship that we can apply to future experiments.
Finally, let’s step through the two scenarios above:
- We run the combined eye tracking and GSR study for our food manufacturer.
- We find that commercial #2 generates the most fixations on their product.
- We also find that commercial #2 generates the longest gaze linger time on their product.
- Finally, we find that commercial #2 generates the strongest emotional response overall, as well as during the times that the customer is looking at the product.
In this case, we can expect the food manufacturer to air commercial #2 during the Superbowl and hopefully receive a strong response. If that’s the case, we can build on our understanding and help companies find the most effective messaging to consumers.
Let’s consider the other scenario, where we run the combined eye tracking and GSR study and get slightly contradictory data.
In this case, we find that:
- Commercial #3 generates the strongest emotional response overall.
- Commercial #1 generates the most fixations and the longest linger time on their product.
So, in this case, we would need to make a decision regarding which sensor is considered more representative or useful in this case. Do we trust the conclusion from the GSR sensor which points to commercial 3? Do we trust the conclusion from the eye tracker which points to commercial 1? Or do we add another sensor to help clarify?
As we can see, fusing sensor data is helpful and informative. When sensors agree, it lends significant credibility to the metrics they generate as they often involve quite different processes. When sensors disagree, it can be a great hint that there are discrepancies in the experimental design or the expected understanding of the phenomenon being studied.
This approach to combining data is called Data Triangulation, and allows for robust and reliable findings when combining data from multiple sensors and paradigms.
The explosion of low cost sensors, internet-connected devices, cloud computing, and affordable wearables is bringing a revolution in our ability to collect data about ourselves, gain actionable insights, and make life better for human beings everywhere. By fusing the data from the sensors together, powerful analytical insights become available. By fusing the data we collect, even further insights are possible. And by triangulating across different methods, we can be confident in our conclusions. Now let’s take a closer look at all the different kinds of sensors available, in our Sensor Fusion Guide.
Let us know your thoughts! Sign up for a Mindplex account now, join our Telegram, or follow us on Twitter.
Optionality & Infinite Games
All things in the universe seem to be constructed out of little parts coming together for greater possibility and greater choices. We call this “maximizing for optionality.”
That means the collective ability to do something more, and greater: to become more than the sum of their parts. Sub-quanta come together into atoms; atoms come together into stars and then planets, as they condense.
From chemicals to organisms
Protocells begin to emerge from chemical processes, and gradually RNA and DNA begin to emerge. From this, we see the first single-celled organisms. And they form biofilms to protect themselves, which they coordinate through radio-like signaling mechanisms.
What we’re seeing here is an exchange between multiple single-celled organisms. The ones on the outside are at greater risk of attack and damage. But these organisms also commensurately have a greater option for finding more food or more resources.
Quid pro quo
Actually, there’s something called “metabolic codependence,” where inner and outer organisms yo-yo. The outer ones expand, but then they run out of an enzyme they need, so the inner ones then govern how fast the biofilm can expand.
Inner organisms want to ensure an equitable trade of resources to feed them, so they provide the enzyme necessary to the outer ones, keeping them strong.
There is a quid pro quo, a trade between these different elements in the greater organism.
Along came mitochondria, and with them, eukaryotic cells, enabling cells to be warm-blooded, powered from within. This seems to be a fortuitous “accident” — mitochondria were once their own lifeform, which found greater negentropy by pairing up with a cell for their mutual benefit.
Symbiotic plants
Then came organisms able to harness an even greater throughput of energy by coming together as clumps of what would later become plants and fungi. Plants can take in energy from the sun via chlorophyll, and fungi can break down matter that otherwise resists decay.
However, the plants had another coordination problem: bigger plants that grew very tall would sometimes block out the sun for the younger ones. So trees coordinate to leave some room in the canopy for light to reach the bottom, a phenomenon known as “tree crown shyness.” They also subsidize the growth of younger plants, sending them nutrients in symbiosis with fungi.
The “wood wide web”
Meanwhile, mycelium webs beneath the forest floor enable plants to swap messages and resources even between species, warning of attacks and exchanging resources for mutual strength.
This messaging is, essentially, based on the same fundamental principle as civilization — technologies to make a dangerous world more reliably safe, achieved through coordination of effort and resources. This “wood wide web” enables ecosystems to prosper mutually, again demonstrating the tendency of systems to maximize negentropy.
The birth of brains
Nature has found many answers to these coordination problems, which seem reasonably equitable. Maybe we could learn from that: some of these cells (possibly mycelium-style cells) began to exhibit extra agency.
They began to connect to other cells that also specialize in this behavior. They became stronger over time, and eventually turned into brains and cortices. That’s why we see harmonics and action potentials akin to swarms of bees within the brain.
Emergence of “free will”
These cells still preserved some of their agency — albeit now a collective agency within the greater mass. And out of this, our sense of “free will” likely manifests.
We perceive ourselves as one individual. But in truth, we are a nation of ~86 billion tiny souls pulling us in all directions, our ego functioning as both ambassador and president, trying to keep a lid on it all.
Come together, right now…
In many ways, this is also a little bit like cellular automata — a macroscale version of microscale neurons. This ability to come together enables ants, for example, to carry off the tasty treat. But it also enables formidable feats of coordination.
Here we have a bolus of ants. Some are drowning, but swap with ones that aren’t drowning. By working together, they can survive. As with a biofilm, the ants most exposed get a little bit of extra help, or sometimes swap around with fresher ants.
We next saw the emergence of cold-blooded creatures, such as reptiles. They were long-lived and hardy but hampered by an inability to operate at all temperatures. They had to bask for a long time to gather enough energy to go into the world.
More complex, responsive brains
The advent of endothermy, warm-blooded creatures, enabled sustained activity to support a more complex and responsive brain at the expense of greater nutritional intake.
The mammalian brain also supports emotions far more sophisticated than the simple Fight, Flight, Feed, and Fornicate impulses of the reptilian brain. Mammals bond with each other in ways that reptiles cannot. Mammals are driven to protect their offspring and friends, encouraging coordination for hunting and watchkeeping.
This ability for mammals to bond with each other enabled our ancestors to bond with dogs and later cats. By hunting, we gain more resources for our big brains. This bonding allowed us to divide our labor so efficiently that some were now able to devote themselves exclusively to thinking.
They created new inventions, narratives, and ways of conceptualizing the world — new ways of solving problems and bringing people together in shared meaning.
Linking up
That sense of meaning and the trust gained by common rules of engagement (morals) enabled us to have tolerance for lots of strangers, and thus tribes became nations. Today, we have planetary information and energy networks linking all of us together.
Along with being able to split the load of work with other humans and other species, we harnessed the secret of fire, developing an obligate dependence upon it.
Fire enabled us to process inedible foods into something safe and nutritious. As our ability to work together in massively distributed, highly specialized labor chains improved, we were able to harness further forms of fire — coal, steam, uranium, to keep us warm. Now, our entire city colonies are heat islands.
So are there innate laws of ethics?
It may be possible for a system trained on a lot of information to pinpoint this seeming truth: the “will” of the universe is trying to connect things for their mutual negative entropy (slowing down the gradual decline into disorder), allowing for mutual improved optionality.
So if different organisms, from plants to ants to human beings, have come together to solve these problems, does it follow that there are innate constructive laws of ethics?
What if an AI could prove definitively that there are such constructive laws for ethics, pinpointing an ethical maxim of “good”? A self-evident, evolutionary epiphany that one can’t unsee once realizing it.
Something like:
(a) Maximizing optionality, which means minimizing entropy, or maximizing negative entropy, through collective benefit that is …
(b) by invitation — not by coercion, not by co-opting, but through equitable mutual benefit. We may even define love in the same way. M. Scott Peck defined love as “The will to extend oneself for the purpose of nurturing our own or another’s spiritual growth.” That sounds pretty aligned to me.
In the immortal words of Eden Abbez, “the greatest thing you’ll ever learn is just to love and be loved in return.”
Perhaps that loving sentiment is more applicable than we knew, expressing “the will of the universe” at all scales and across all time.
Let us know your thoughts! Sign up for a Mindplex account now, join our Telegram, or follow us on Twitter.