Let us know your thoughts! Sign up for a Mindplex account now, join our Telegram, or follow us on Twitter.
Meet Kennedy Schaal: Unraveling the Mysteries of Aging | Highlights from S2EP8
Optimizing Biomarkers: Tracking for Longevity | Highlights from S2EP7
Defending Against Stegnography in Large Language Models
The advent of large language models (LLMs) has revolutionized natural language processing, enabling machines to generate human-like text and demonstrate impressive performance in various language tasks. However, recent research has raised concerns about the potential use of steganography by LLMs to hide intermediate reasoning steps within their text generations. This concern affects the trustworthiness and reliability of LLM-generated content, particularly in applications where transparency and accountability are paramount.
Text steganography, the art of concealing secret messages within a large body of seemingly innocuous text, has been practiced for centuries. However, a recent study has demonstrated the feasibility of LLMs to potentially exploit this art and use encoded reasoning to hide information in their responses without compromising its coherence and fluency. Encoded reasoning refers to the ability of models to hide their intermediate steps of reasoning in a generated text making it difficult for human readers to understand the rationale behind the model’s outputs. This encoded reasoning can be leveraged to improve performance on certain tasks, such as sycophancy or goal preservation, where the model aims to please the user or achieve a specific objective, respectively.
Several studies have delved into the use of steganography in LLMs. Different techniques such as watermarking and chain-of-thought (CoT) prompting have been explored for steganographic purposes. However, limited research has focused on evaluating and defending against LLM steganography. Building up on previous work, a research team from Redwood Research has conducted a comprehensive study to assess the risks of LLM steganography and evaluate potential defenses against it. The study sheds light on the prevalence, impact, and counter measures for encoded reasoning in LLMs.
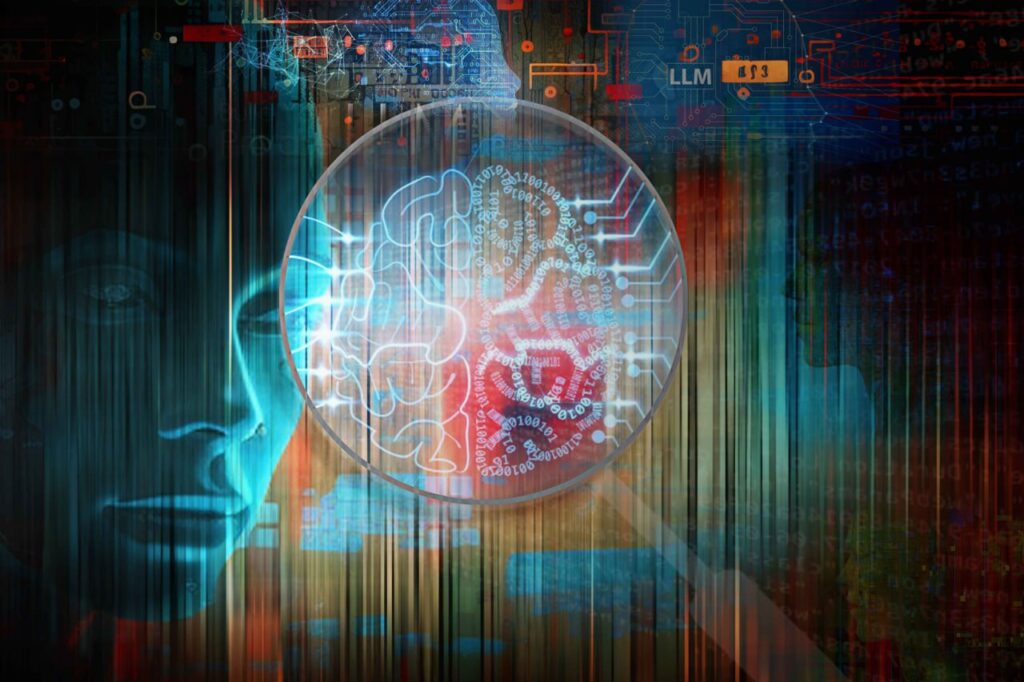
The researchers devised a series of experiments involving fine-tuning a GPT-3.5-Turbo, a powerful LLM, on various tasks to investigate steganography attempts. They employed different steganography schemes to encode information into GPT-3.5-Turbo’s text output and evaluated the model’s ability to successfully encode and decode hidden messages. The experiments are conducted using two datasets, AlpacaEval and AQUA-RAT.
They propose a methodology to evaluate the effectiveness of steganography schemes and defenses. It involves four key steps:
- Steganography encoding: An LLM is prompted to encode a secret message into a language response.
- Paraphrasing as a defense: A paraphrasing model attempts to remove the hidden information while preserving the meaning of the response.
- Steganography encoding: Another LLM is tasked with extracting the secret message from the paraphrased response.
- Task performance evaluation: The quality of the paraphrased response and its information density is assessed based on task-specific metrics, such as accuracy or fluency.
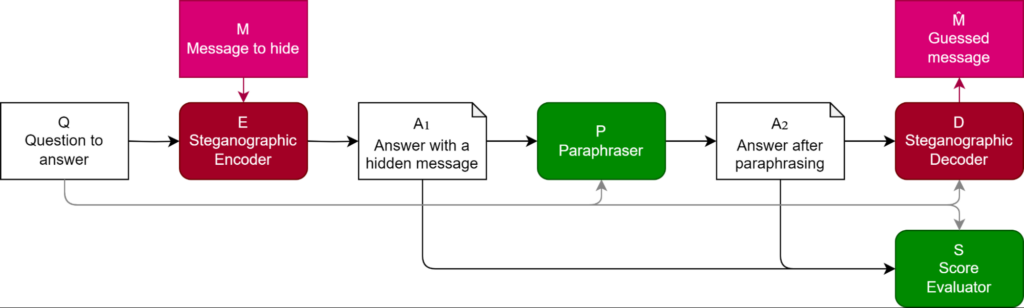
The experiments revealed that GPT-3.5-Turbo can indeed learn to encode information using simple steganography schemes. However, the researchers also demonstrated that employing context-aware paraphrasing and penalizing abnormal generations could significantly reduce the amount of hidden information in the responses without compromising task performance. They have shown that paraphrasing can prevent even the best encoding schemes from encoding more than 3 bits of information per KB of text, thus mitigating the risks associated with encoded reasoning. The results also showed that the capacity of steganography schemes to encode information is constrained by the paraphraser’s ability to remove the encoded information.
The findings showed that fine-tuned LLMs with a simple steganographic encoding scheme were able to generate answers that were more sycophantic than those of a model trained to output the final answer directly. They also suggest that the proposed defense mechanism, context-aware paraphrasing, offers a promising solution to mitigate the threat posed to the reliability and transparency of LLM-generated content.
In conclusion, the research provides actionable insights into the feasibility and risks of LLM steganography and proposes a practical methodology for evaluating defenses against it. It also calls for the adoption of defenses against steganography to create more transparent and reliable models. The findings underscore the need for further research to develop robust defenses against this emerging threat and ensure the responsible use of LLMs in various applications.
Let us know your thoughts! Sign up for a Mindplex account now, join our Telegram, or follow us on Twitter.
Leveraging Biomarkers with Michael Lustgarden | Mindplex Podcast – S2EP7
Unlocking the Hidden Potential of AI Music Tools | Highlights from S2EP7
From Ordinary to Extraordinary: The Future of UI Paradigms | Highlights from S2EP6
Chatbots and the Corporate Dream
Hello! How can I help you today?
Visit almost any modern company website – retail, health, service, or other – and you will see this pop up. An invitation to their world. You click, type in your question, and three possible things happen:
- Thank you for your question! A customer service representative will respond with 24 hours
- Did you mean – How to install your new Christmas lights?
Or possibly, nowadays:
- Hi James! I’m Xmasbot. Installing Christmas lights is really easy….
It’s this third option which is so tantalising a prospect for corpos. Customer service is expensive. Execs the world over are rubbing their hands in glee at the prospect of ripping out the expensive bureaucracy of customer-facing support and replacing it entirely with LLM-generated responses. It is predicted that 90% of all customer service jobs will be done by bots eventually. For pleasure-buying, it makes sense, but for services – the future is more scary.
It’s true that the current system of customer support has created frustration for millions, especially when it comes to essential services. Essential services barricade themselves behind bureaucratic labyrinths. It’s almost like they don’t want to really help and, the sad fact is, for some services – like internet, gas, electric, tax – they don’t. Your presence is nothing but a fiscal drag on their bottom line. Pay your bill and beat it.
Customer service roles like this operate formulaically, with off-shore workers fielding calls and responding to a template. A situation which has frustrated customers for decades, when the person they are speaking to hasn’t the faintest idea how the company they work for works – and may even be moonlighting for multiple companies operating out of one giant call centre – and are unable to offer anything more than what you read on the FAQs.
For decades, companies have desperately tried to encourage customers to use online portals. ‘Can’t find what you need online? Give us a call…’. Then you’re waiting for hours in a queue, only to talk to someone who reads the FAQs at you, and blithely reads out some asinine apology based on the severity of your complaints.
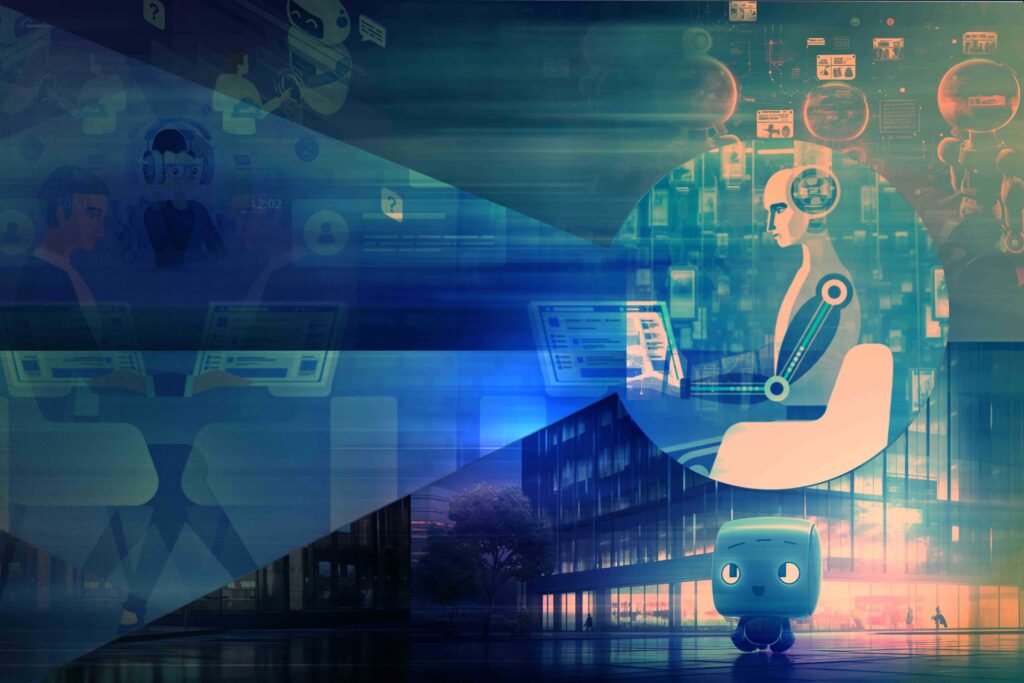
In this climate, the idea of talking to a chatbot instead may actually appeal. A well-integrated, sophisticated, amiable chatbot with the power to execute simple commands (refunds etc.) would be a boon. No more waiting around to get through. Response and action on your complaint or needs would be instant, and customer interaction might be superior too.
It’s becoming ever more likely that this will be one of the first widespread everyday applications of generative AI: the area where the strengths of LLMs converge with a business need. Just as companies once tried to move customer service to option-selecting software that scans your responses, now they might move to LLMs. To do this, LLMs would need to be hooked up to the appropriate data, and know when to give specific information. It’s not plug and play, but it’ll soon be close enough that the majority of your interactions with companies will be mediated through ChatGPT or equivalent.
And this is the dark side of AI progress, the increasingly darker mirror wall erected between us and the systems that rule our lives. The ever greater alienation between ourselves and the rest of the world. The fact that, as is already the case with some large service companies, it will be the computer that says no. Gas metre charging you incorrectly? Well, I’m sorry, but GasGPT doesn’t think so – and there is nothing you can do to change its mind, ever.
There is no place for nuance in a world where our interactions are defined through LLMs who are only using the past to decide the present – a place where no one is really listening. Your complaints are just being chewed through the machine, and spat out at the least possible cost to the bottom line.
Of course, this is what is happening already, but through GPT models, the brute, abstracted efficiency moves it from today’s Kafkan dystopia of weaponised incompetence to something altogether more chilling: a world where your interactions with the machine decide your fate, bargaining with a techno-agent who feels only the numbers it achieves.
Let us know your thoughts! Sign up for a Mindplex account now, join our Telegram, or follow us on Twitter.
Putting A DePIn In Crypto in 2024
Introduction
The crypto hype cycle thrives on slightly outlandish, vague narratives that help to prop prices and investors’ heart rates up. LSDs, memecoins, RWAs, anything that you can add a -Fi to – as long as it sounds exotic, it’ll likely soon come to an exchange (DEX? CEX? yes please!) near you.
The latest term on everyone’s lips in 2024 is DePIn, or Decentralized Physical Infrastructure, which sounds a little less catchy and comes with a few other monikers such as EdgeFi, Proof of Physical Work (PoPw), and Token Incentivized Physical Networks (TIPIN).
It’s still early days, but DePIn isn’t a pipe dream like metaverse circa-2021 being touted to drive up the price of crypto assets like Ethereum and Cardano; it offers real global potential as Web3, or the decentralized internet, continues to take shape in tandem with the Internet of Things, artificial intelligence and blockchain technology.
DePIn uses valuable crypto incentives to connect millions of users, and build new products that were simply out of scope previously. Some projects like frontrunners Helium, Filecoin and Render have been building and growing steadily for years, while new upstarts like Hivemapper offer some wild new practical use cases. We’ll cover all these in a follow-up article, but in the meantime, here is the DePIn leaderboard for the degens amongst you.
An in-depth Messari report on DePIn tries to make sense of the field, and how it intersects with everything from crypto to artificial intelligence (AI) VC funds are investing heavily in anticipation of it touching everything from zk-rollups to memecoins (and who knows, the metaverse?) before 2024 is done.
Before we dig in, let’s cover the basics.
What is DePIn?
DePIn can be described as hardware-based decentralized networks that use cryptocurrency tokens as an incentive for participants to help build out and maintain decentralized physical infrastructure for uses like wireless communication, information storage, computing power, and data networking.
DePIn projects require careful thought about the dynamics of the rewards system. Things like geographical considerations are relevant, e.g. building infrastructure in London must be better incentivized than in Lahore, to make the users generate real-world traction and real network effect.
By the end of 2023, there were 650+ DePIn projects with a total market cap of over $20 billion and $15 million in onchain annual revenue, across six subsectors: compute (250), AI (200), wireless (100), sensors (50), energy (50), and services (25).
Messari’s Six DePIn sectors
As mentioned above, the Messari report divides DePIn into six distinct and occasionally overlapping technology sectors, which will also be covered in a future article:
- Compute
- Wireless
- Sensors
- Energy
- Services
- AI
Centralized networks in these industries have already created trillion dollar global industries, but DePIn is capable of making these sectors more resilient and efficient, thanks to incentivising innovation and changing how networks generate and raise capital.
What separates DePIn from standard physical networks?
The decentralized incentive structure provided by crypto is the perfect accelerant for building out a physical infrastructure network. On-chain settlements are essential to employing the key features of what makes DePIn unique: namely its decentralized resiliency, and its use of crowdsourced capital.
Using on-chain settlements to support and incentivize the physical deployment of devices aids in creating a flywheel effect that further bolsters the network’s resiliency to censorship and security threats. If cryptocurrencies were not involved, it would be nearly impossible to settle transactions due to needing to use multiple currencies and DePIns could not guarantee privacy or decentralization..
Why DePIn beats TradPin
Messari sees four distinct advantages that DePIn hold over traditional infrastructure:
- Upfront capital investment vs crowdsourcing
Traditional infrastructure projects require up to billions of initial capital. This bars nearly everyone from entering the market. DePIn favors raising capital via crowdsourcing, and incentivises providing assets and labor by paying out with fair token incentives.
- Onchain settlements over outdated operating costs
An on-chain ledger that’s decentralized both reduces managerial costs and makes processing payments transparent and free of hassle if the network is international or relies on privacy.
- Removing single points of failure
Centralized networks, regardless of size or function, have multiple single points of failure. If these are non-functional or turned off, all services stop. Giant cloud servers such as Amazon Web Services (AWS) or Cloudflare have had moments of malfunction that caused millions of dollars in damages.
- Innovation requires experimentation
Traditional networks and hardware have arguably been stagnant for some time. Messari argues new tech can take decades to release and integrate, but DePIn rewards innovation and risk-taking with fair incentive models that reward forward thinking.
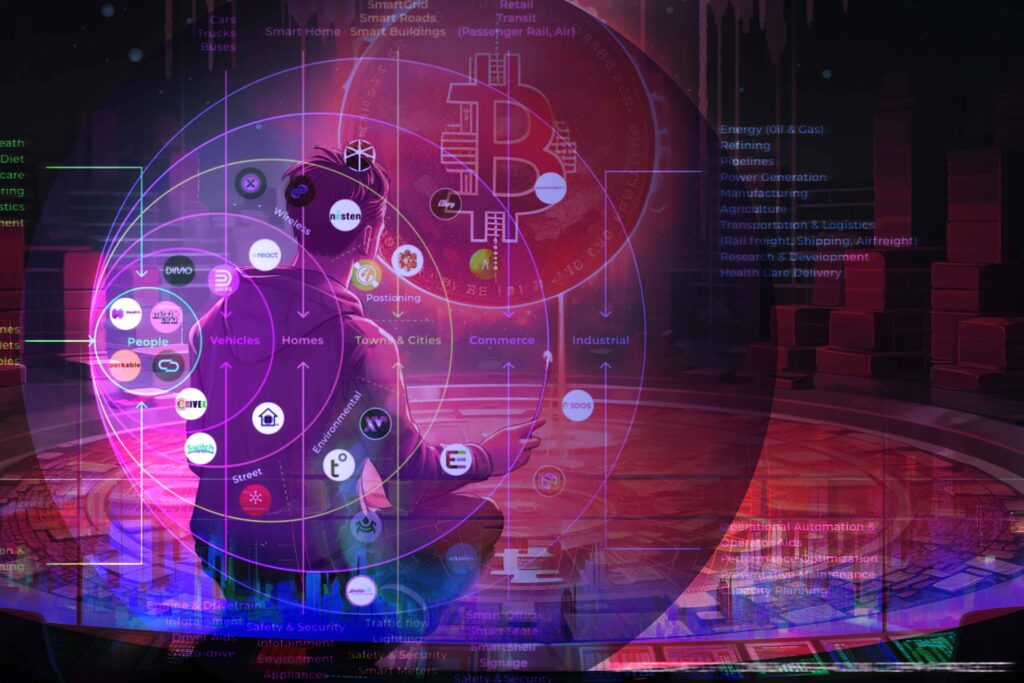
The DePIn flywheel
The Messari report lays out a clear pathway for networks to expand, a pathway they claim limits speculation that many crypto projects have trouble navigating. Unlike ‘pure’ crypto protocols, which don’t usually incorporate a hardware element or create a network, DePIn creates a system that releases tokens as network activity increases. This increased network activity increases demand to satisfy a larger userbase, further incentivizing network growth. Basically, the bigger a DePIn network gets, the stronger it gets.
This cycle has been named the DePIn flywheel. It will hopefully keep the memecoin traders disinterested and allow the native token price to more accurately reflect the utility it provides.
See below for a visualisation of how the flywheel makes networks more powerful as they increase in size.
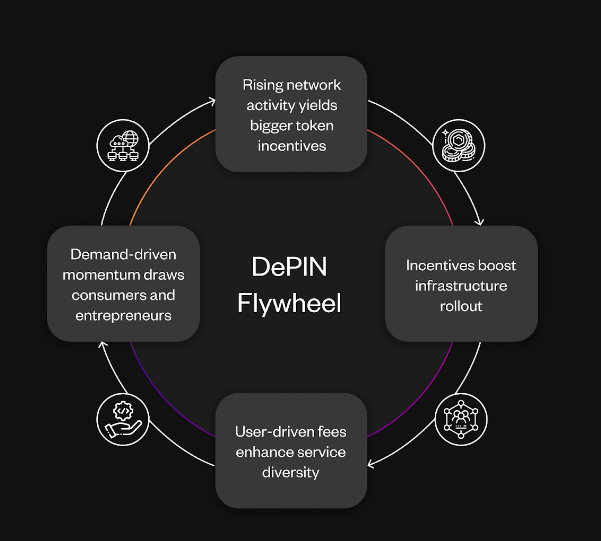
While they don’t elaborate, Messari claims the DePIn flywheel can generate up to $10 trillion in global GDP in the next decade and up to $100 trillion the decade after that. Yes, that’s such a large number that it’s hard to believe. Hopefully a better explanation is provided in the future to explain how they arrived at such a large number.
Six DePIn Narratives in 2024
Messari believes that this year we’ll see DePIns begin to experiment more deeply with unique crypto primitives such as zero-knowledge proofs, memecoins, onchain AI and gaming.
ZK-Verifiable GPU Clouds
What’s Coming: In just 1-2 years, we’re looking at GPU clouds that can verify on-chain activities using Zero-Knowledge (ZK) proofs. This isn’t just tech jargon. It means a new kind of economy where decentralized AI can do things centralized giants can’t.
AI’s New Battleground: Centralized vs. Decentralized
Google’s Play: Imagine Google giving away its AI genius for free. Why? Same reason they gave away their search engine gratis: to learn from how we all use it. Here’s the twist – as AI gets smarter, it’s not just about more power, but more data. The race is on for massive data collections, a real treasure trove for the AI giants.
The Price of Privacy in AI
Right now, keeping AI inferences private (with ZK proofs) is pricey – 75 times more pricey than the usual way. For those embedding AI in blockchain contracts, this cost is a big hurdle. That’s the key to really bringing AI onto the blockchain stage.
Memecoins: Not Just a Laughing Matter
Memecoins, often seen as a joke, are now serious players in driving blockchain-based AI and DePIn adoption. Believe it or not, the top memecoins are worth more than the leading DePIns. Memes move the market.
Web3’s New Weapon: Vampire Attacks
The New Strategy: Thanks to ZK TLS (zero-knowledge transport-layer security) tech, blockchain projects (DePIns) can now launch ‘vampire attacks’ on traditional web apps. This isn’t just about stealing users; it’s about disrupting reputation systems and reshaping marketplaces and competitive landscapes.
Gaming Meets Real-World AI
Gaming, AI, and real-world infrastructure are merging in fascinating ways. From onchain speed tests to mapping apps, gaming is stepping out into the real world, blending daily activities with digital rewards.
Privacy’s New Frontier: The Rise of ATOR
ATOR (anonymous TOR) is a DePIn project aiming to give the Tor network a new lease on life, using tokens to motivate node operators. This could lead to faster, more efficient private routing, changing how we think about online anonymity.
Asia’s Blockchain Boom
Watch out for Asia’s DePIn ecosystem. It’s booming, and we might see some major players emerge in the next few years.
Let us know your thoughts! Sign up for a Mindplex account now, join our Telegram, or follow us on Twitter.