Paul Cockshott is a computer scientist and economist, with an interest in how advanced computerised planning can supplant our existing economic order. I spoke with him about how Artificial Intelligence will automate jobs that people currently do. The discussion focuses on the economic costs of training AI models, how they weigh up against labour costs, and the economic circumstances under which human jobs will be automated. Over to Paul Cockshott:
* * * * * * *
The use of AI requires a great deal of processing power. It needs it in two distinct ways. The first is in training, and the second is in application.
Let’s look at the training aspect. This has become feasible because of two developments over the last 15 years, in data and in power.
Data
The build-up of large collections of images and text on the internet that can be used as training data for neural networks. I recall back in the 90s when a team I worked with was developing neural network video encoding, one of our problems, pre internet, was just getting and collections of image data to train with. We resorted to capturing TV broadcasts and training neural nets on those. Now, of course, due to Android camera phones, Google has almost unbounded collections of images from around the world on which neural nets can be trained for vision purposes. In addition, there are vast quantities of indexed images on the web, with dubious ownership, that smaller groups like Stability.AI can use. The same applies to text. It is the ready availability of a vast corpus of academic papers and books that makes systems like ChatGPT and Bard able to answer questions, if not like an expert, at least like a 3rd year student.
Power
Actual nervous systems work by electrochemical means to aggregate multiple discrete impulses to produce a discrete response. The Church–Turing–Deutsch principle states that any physical system can be emulated to an arbitrary degree of accuracy by a universal computing machine. This includes the semi-analogue, semi-digital processes that occur in nervous systems. Whilst this has been theoretically known at least since the 1980s and in informal terms since the 1950s, it was, until recently, impractical to apply on a large scale.
To emulate the analogue aspects of synaptic responses requires a great deal of floating-point arithmetic, more specifically it requires a lot of matrix to vector multiplication. A lot of work from the 1960s went into developing supercomputers for matrix mathematics, since these techniques turn out to be of very general applicability in the physical sciences. By the end of the last century, this had produced machines that were well able to handle tasks like climate simulation and weather forecasts.
But the scale of maths required by artificial intelligence was considerably greater. The human brain contains tens of billions of neurons, and each neuron would have to be represented by a vector of synaptic weights. If each neuron has or the order of 10,000 synaptic weights and can fire about 10 times a second, we would require a vector processor of from 10¹⁵ to 10¹⁶ operations per second to emulate the brain: that is to say it would have to reach the petaflop range.
The first machines in this range became available about 12 years ago. Last year, Tesla launched its Dojo supercomputer complex with a processing power of 10¹⁸ operations a second. That makes it equal to around 100 human brains in processing rate. The downside is the power usage – in the region of 1-2 megawatts. In comparison, the metabolic energy consumption of 100 human brains would be of the order of 1.5 kilowatts, so the Dojo system is about 1,000 times as energy intensive.
The machine is built of 120 individual ‘training tiles’ as shown below.

However, at this point we are just comparing operations per second, not information storage. A brain with 80 billion neurons each with 15,000 connections would have 1.2 quadrillion weights. Tesla stores its weights in cfloat8 format, so that each of their training trays can store about 11 billion weights or about 1/100,000 of a human brain.
So the current best Tesla technology is 5 orders of magnitude behind the human brain in storage, and 3 orders of magnitude behind in energy-efficiency: overall about 8 orders of magnitude away from the storage x power efficiency of the human brain.
The consequence is that whilst it is possible, by consuming megawatts of power, to train a system on a specialist skill like driving, it is not yet possible to incorporate a human level of intelligence and knowledge into the car itself.
A human can be taught to drive with a few tens of hours of driving instruction, and they can still do other jobs after they have driven to work. Tesla must spend years of processing time at a huge power bill to obtain the set of neural weights that a person needs to drive.
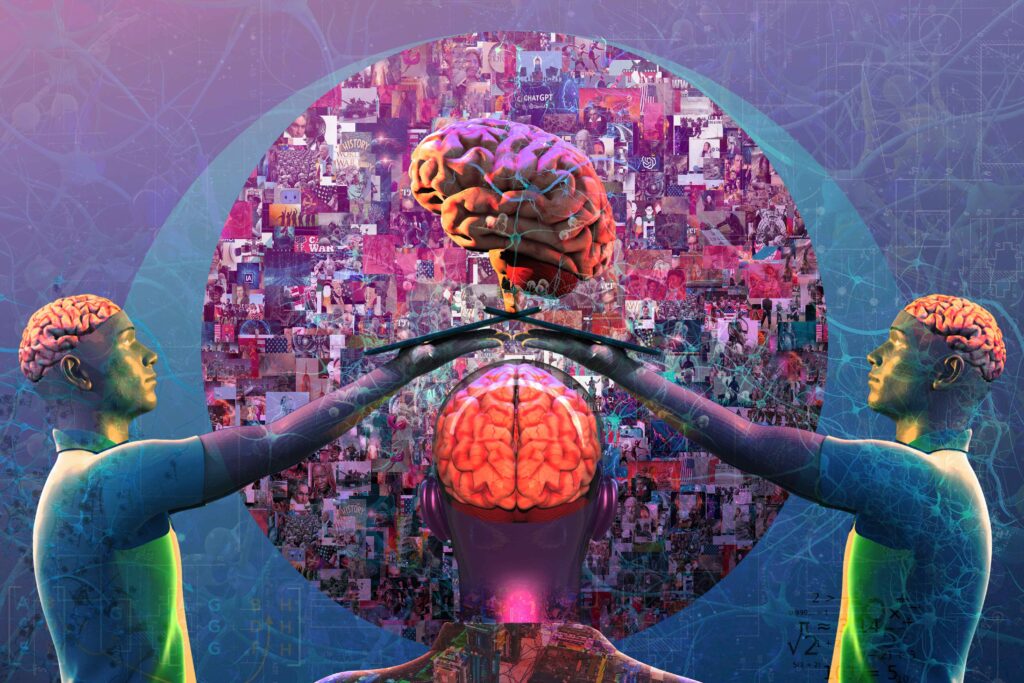
Of course, the Tesla business plan is to train once and then replicate the information in all their cars. But the size and power hungry nature of the chips at present prevents them being put in each car.
It will take some time, one or two decades, before the energy × storage efficiency of chips reaches a point where mobile robot devices with general intelligence comparable to humans are likely to be available. So, to harness general AI, a lot of effort must go into improving power consumption and memory capacity of chips. Until that point, it will be only available as remote online services running on big data centers.
These in turn will increase demand for electricity at a time when, due to environmental considerations and political instability, energy is becoming scarce and expensive. The implication is that an ever-increasing share of GDP is going to have to be directed to producing non-fossil fuel energy infrastructure.
Let us know your thoughts! Sign up for a Mindplex account now, join our Telegram, or follow us on Twitter.