In the 2017 alt-currency wave and 2020s’ DeFi summer blockchains were built to be much faster and smarter than Bitcoin, the original blockchain, and with on-chain capacity for much more diverse financial and social primitives (identity, art, savings, loans, and more). The transaction speeds of these currencies far outstripped their by-now plodding ancestor. Yet the antiquated Bitcoin technology is king among blockchains. Coins that work better than Bitcoin have been around almost as long as Bitcoin itself – yet Bitcoin still has more financial value than the rest of the industry combined.
Still the King
Ultimately, it’s all about trust. This is the people’s money we’re talking about. The value of a decentralized ledger or blockchain is fundamentally underwritten by the fact that people know it works. Bitcoin has performed exactly as outlined in the whitepaper ever since its inception, supported by a vast chorus of computers all over the world. It hasn’t double-spent, it hasn’t failed a transaction, and its network of miners is now so vast that its security is immutable. Humanity has achieved a peer-to-peer electronic cash network with no centralized oversight. An achievement so intoxicating that our race to consecrate and order other digital interactions using the blockchain has led to a sector worth over $2 trillion. A brand new asset class that, with the dawn of Bitcoin ETFs, is now traded by pension funds all over the world. The new utilities that distributed ledgers provide have proven they can change the world.
The altcoin market has its problems: slow technical development, overindulgence in capital raises, and vulture-like VC activity, alongside outright criminal fraud. This has led to slower progress than some expected. Blockchain has gotten traction in certain use-cases: supply chain tracking, sending remittances, controlling access to fandom communities and more. But crypto’s mainstream deployment in everyday sysadmin still feels distant. One reason is Bitcoin’s grip on investors’ capital. Along with perhaps Ethereum, Bitcoin is the only trusted mainstream, and broadly stable, asset the market has yet produced. Hedge funds, neobanks, and long-term HODLers keep their capital frozen in the assets at the summit of the market, stopping that liquidity from irrigating the thousand flowers in the valley below.
Building Better Bitcoin
So the question arises. Rather than look to new networks to establish new utility for crypto, can’t we use the one we’ve already got? Maybe we can polish the pistons of the Bitcoin core code and use that trust as the foundation on which new utility can run. Can we employ cryptographic lessons we have learned to make it run faster? Now everyone agrees it’s awesome, can we make it harder, better, faster and stronger?
It’s not a new thought. Bitcoin Cash was the first major attempt to upgrade Bitcoin. Yet it did it through a fork, not a rewrite – and when it comes to money, people like what they already know. Although Bitcoin Cash does have market cap, it’s ultimately a footnote in the Bitcoin network’s broader history. For new utility to exist on Bitcoin, to upgrade Bitcoin – technologies need to be built that work using the main Bitcoin consensus itself. Attempts have been legion. However it is recently that there is a sense that the Bitcoin developer community is getting somewhere useful.
Utility projects have seen the best progress in recent years. Ordinals are a way to uniquely identify and order satoshis, and inscriptions allow users to attach arbitrary data on the resultant tokens. BRC-20 tokens, inspired by Ethereum’s ERC-20, leverage these by inscribing metadata onto uniquely numbered individual satoshis, delineating them as special tokens – which can then be used for utilities like stablecoins, NFTs, and access-control. This process runs entirely on the Bitcoin blockchain, and that’s good for trustworthiness, but it does clog up the network with data on an already constrained blocksize – leading many to question the practicality of such efforts, not to mention the need for wallets and infrastructure to engage with these specialized tokens.
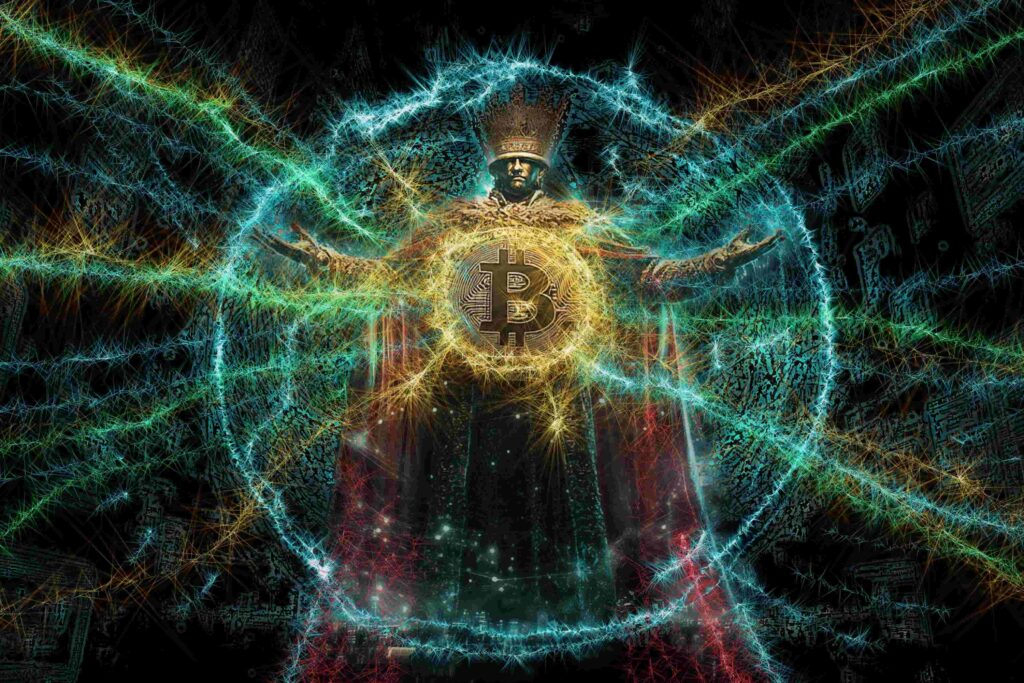
Utility and Scale
Following on from BRC-20, Runes were deployed to much fanfare. By using UTXOs to mark individual satoshis, Runes drastically reduced the congestion overhead for Bitcoin, while also making it far simpler for users to mint their own runic satoshis. Despite an initial surge in activity as a result of Runes, the promise of ‘DeFi on Bitcoin’ quickly tapered off in the face of how cumbersome they are to trade, and the fact that the market efficiency of trading runic satoshis still operated on a direct barter system rather than decentralized liquidity pools – something anathema to a crypto market used to trading on Ethereum. Recent attention has fallen on ‘Fractal Bitcoin’ as an antidote to this, but the tech is in its infancy.
What about scaling the network? The Lightning Network is the stalwart of the space, creating off-chain payment channels between users, reducing load and facilitating micropayments. For all its scaling potential, it suffers from having a third party settlement layer, so that as Bitcoin scaling grows so do settlement fees on Lightning.
Then there are parallel blockchains, sidechains, and layer-2s like Liquid Network, Rootstock, and the Spiderchain. All of these peg to Bitcoin, and all offer the potential for smart contracts and additional utility – but they all suffer from the same old issue of consensus. You may use Bitcoin as authority for the transaction you validate, but what if your validators go rogue? Ultimately, if the scaling used doesn’t use the main Bitcoin consensus network and core code – then you’re just another altchain. Bitcoin, it seems, may never solve the trilemma, and may never grow wings to become an all-purpose blockchain.
Mastering the Old Ways
And maybe that’s okay. Maybe those liquidity glaciers never need to melt. Maybe sometimes the old ways are best. Save the utility for the new kids. The purpose-built speedsters use the latest tech, rather than trying to stick a brand new V12 engine in a forty year old car – it’s just not built for it. The Bitcoin tortoise has led the race for 15 years – perhaps we should just let it do what it’s good at.
Let us know your thoughts! Sign up for a Mindplex account now, join our Telegram, or follow us on Twitter.