I first learned about Vinay Gupta just about a year ago when Bruce Sterling told me he was in a discussion with a guy who was declaiming about how important MONDO 2000 was and how much he was influenced by it. I looked him up and learned that he had been involved in the launch of Ethereum, and since then has created something else called Mattereum. I was just starting to launching an NFT project called ‘I’m Against NFTs’. I started the work with former MONDO 2000 participants on the project at a time of gold fever for visual and audio NFTs. So I called upon Vinay, among others, to guide me through the fog around bringing NFTs to market.
As things evolved, the project became a song and an immersive environment with PlayLa.bZ and associates that was presented at MOZFest. The NFT fever abated, and the “I’m Against NFTs” offering was shelved. But I’d grown to treasure my connection to Vinay and to admire his philosophic depth and sharp intelligence regarding blockchain politics and the state of the world in general.
As I was completing my second column here on the topic of Risk and Precarity in Web3, I realized that I had to lean on Vinay’s wit and wisdom for “Risk and Precarity Part 3: Possible Solutions.” Here I present our conversation about the issues raised by my earlier columns.
Vinay Gupta helped to coordinate the release of the blockchain platform Ethereum, and is the founder and current CEO of Mattereum, which offers legal protections for physical assets sold on the blockchain. He’s clearly had a full life and has done a lot of other amazing stuff, some of which comes up during this conversation.
RU: I’ve been writing about tech culture’s romance with risk and adventure, and the scams we find in blockchain activities today. When you were helping to start Ethereum, was risk — personal, for other individuals, for the wider economy and culture — part of your calculation?
VG: Firstly, I want to say a huge thank you for your work on MONDO 2000. I was a Scottish teenager living in the crappiest part of a crappy town, before the age of the internet, and one day I was in a board game shop and I saw this glossy, colourful magazine. Picked it up, browsed through the contents, bought one, then another and it literally set the direction of my whole life! I knew I had to get out of Scotland and get to America where it was all happening. I arrived in 1995 and I was not disappointed.
I don’t know that I would have ever made that journey without you. MONDO 2000 was a beacon, a homing signal for me and I am sure many others. Well done. I think you’ve had more impact than you could ever know. Anyway, back to the question of risk.
Before I joined the Ethereum Foundation in 2014, I had spent about ten years as a worst case scenario disaster guy working for government and academia, including stints for a variety of military think-tanks at quite a high level in both America and the UK.
The terrain I worked in was unholy. “I don’t get out of bed for less than 1% mortality” was one of my sayings. In fact 1% mortality wasn’t a thing I ever really paid attention to. My work really kicked in at 10%, 20%, 30% mortality. Genocides, smallpox or worse pandemics including bird flu, nuclear terrorism – that sort of thing.
We got very, very lucky with Covid. It could easily have been ten times that bad. If it was bird flu it could have been fifty times that bad. I’m not kidding, these are the numbers.
To stay sane during that period I referred to my work as ‘apocalypse mitigation’ because my territory was after prevention has failed, now what? My business cards were designed to set the tone, and were legendary.
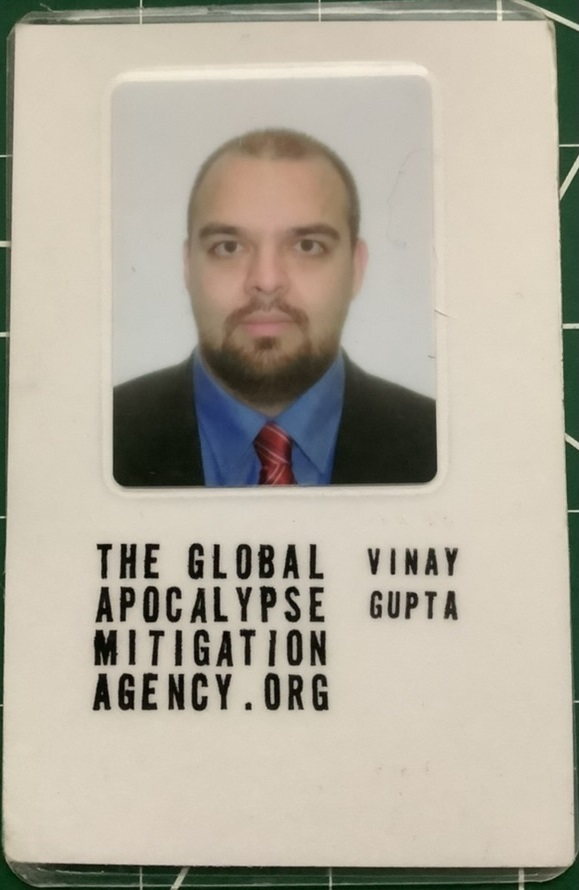
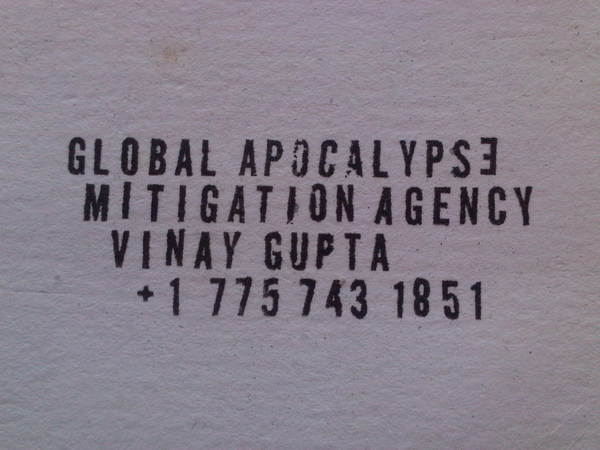
Of course climate change was high up my list because a wracking wave of global droughts and famines will also unleash resource wars and accelerate a global cycle of decline. This is why it’s so important we get into geoengineering early.
But nobody wanted to pay for the truth about risk. Discovering the truth about things like inadequate government preparedness for pandemics wasn’t paying the bills. Nobody in government was willing to do the work to fix the problem. As we have seen with Covid.
Eventually I just ran out of money. So I largely focused around my own survival and got a job in tech, with the Ethereum Foundation. Most of my civilian friendly work around the open source hexayurt refugee shelter system is linked from myhopeforthe.world which gives a summary of the approach. I’ll get back to it if I ever succeed in becoming independently wealthy.
So I would say I was one of the most risk-aware people in the world when I joined the Ethereum Foundation in 2014.
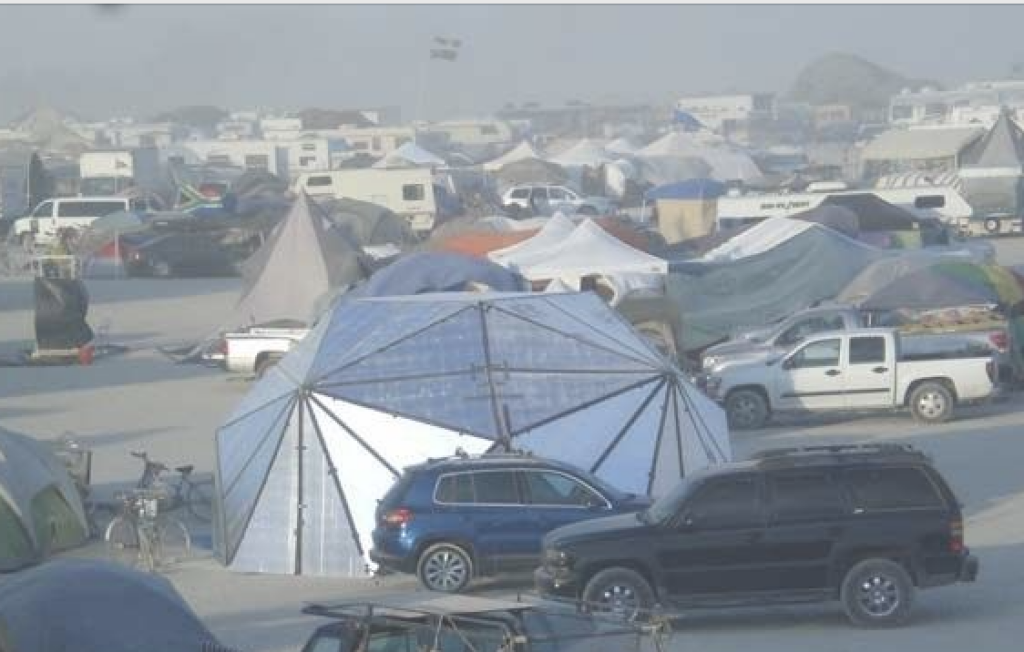
RU: What do you make of the emphasis on anonymity as a protection for individuals as it was emphasized by early crypto money people like David Chaum, the cypherpunks etc.?
VG: David Chaum and the cypherpunks are right. Modern crypto is mostly wrong. I say ‘mostly’ because crypto is littered with fully anonymous actors jealously guarding their True Names. Unfortunately a lot of those anonymous individuals are among the worst scammers in crypto.
But the rest of crypto went down the path of using centralized exchanges. KYC AML CFT PEP SOF* checks and all the rest. [KYC = Know Your Customer; AML = Anti Money Laundering; CFT = Combating Financing of Terrorism; PEP – Politically Exposed Person, SOF = Source of Funds] The problem is that these exchanges have a foot in both worlds: they’re cypherpunk on the side where they’re getting tokens from the raw chaos of the blockchains. They’re TradFi (traditional finance) where they are taking copies of passports and verifying home addresses. As a result, all the legal liability piles up in the exchanges and they also have huge opportunities for operating their own scams: see FTX.
The SEC case against Coinbase is likely to be where a lot of this mess gets sorted out but I am not liking Coinbase’s chances.
RU: So the crypto that you like — the anonymous crypto, uncompromised by TradFi… is it for the masses or is it an elite thing, at least at first? Does it translate into a meaningful means of exchange with which to get, say, food, clothes and housing, and is it a longer wait to get there than TradFi?
VG: Crypto gives the same kind of privacy that everybody had when they made payments before the invention of credit cards: cash. It’s not some magical new thing, it’s just using cryptography to enable computers to maintain our human and civil rights in the face of a changing technological environment.
Cory Doctorow seems to have a serious dislike of anonymous electronic cash, and I don’t understand why. It’s a defense of human rights, nothing more and nothing less.
My guess is that CBDCs (central bank digital currencies) will wind up wrapped in anonymizing layers to protect civil liberties in most jurisdictions: only the most repressive of regimes would force their populations to have all their transactions recorded on a central government ledger! That’s probably how cryptocurrency makes its way into the mainstream economy. That’s how people pay their rent, buy clothing and food, and everything else. CBDCs with privacy layers provided by third parties using cryptography to make private transactions with central bank digital cash.
In this scenario what becomes of Bitcoin? I think the answer is that Bitcoin doesn’t change much. Maybe they migrate to entirely green energy (please!) but other than that I think the honey badger don’t care.
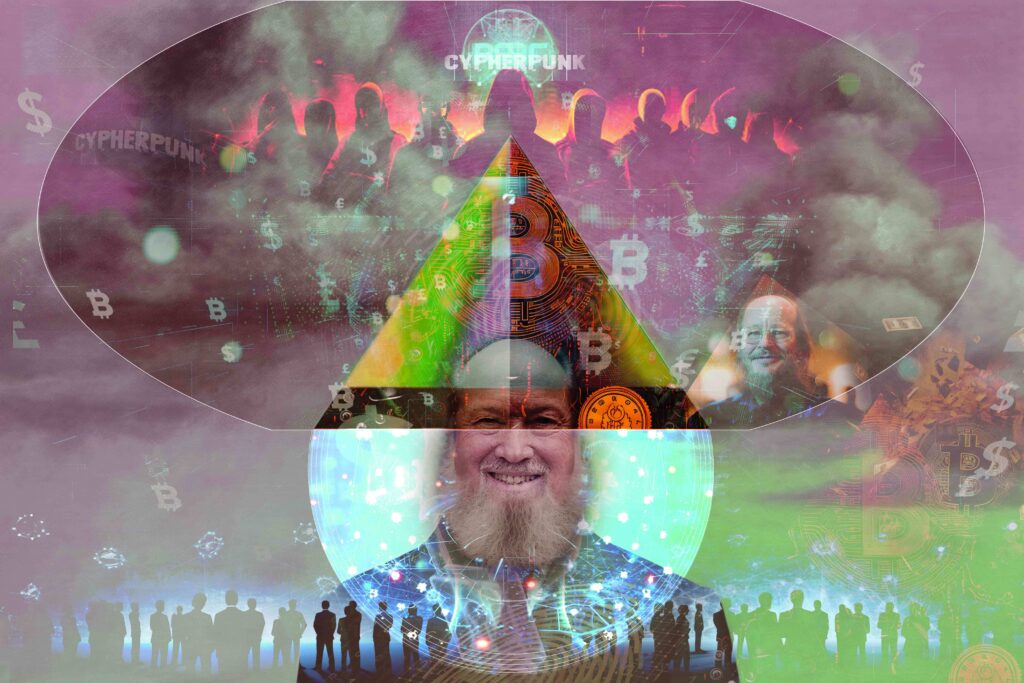
RU: With Ethereum and then with Mattereum, you’re working within the current reality of crypto largely connected to TradFi. What are some of the strategies for making the terrain as secure as possible for the average person looking to improve their lot via crypto?
VG: There are a lot of ways to earn money with crypto. Many of them are what I would term ‘zero sum games’ – every dollar gained by one person was lost by somebody else. It’s often like a lottery. There are two major non-zero sum ways to make money with crypto –
The first is people printing their own money rather than having nation states do it for them. Any good anarchist should support this and the libertarians of course do too. If that ‘People’s coin’ turns out to be widely adopted and more people want to trade goods and services for it then its purchasing power increases. Bitcoiners believe in this model above all others.
The second is a little more complex: if it costs 1.4% to pay with VISA, and 0.4% to pay using Polygon, then 1% of the value of the transaction is there to be split between the buyer and the seller. If there are goods on sale and it’s $100 with VISA or $99 with crypto you are going to pay with crypto every single time.
For retail transactions, that’s a relatively small effect. Right now the hidden costs of payment processing are mostly silently borne by the merchants. But there are similar margins being claimed by third parties all the way through the financial system. They implement patterns of trade unchanged since the Age of Sail. Digitizing these archaic trade systems properly – it has to be done right – is going to put a couple of percent on global GDP through sheer efficiency. That’s only the first step, mind you.
RU: How does your more idealistic notion of a circular economy intersect with crypto? And since this is a site for those enthusiastic or curious about AI, how do you see current AI (if we can call it that) being a part of any potential transition?
VG: The second step of trade digitization is AI-based trade optimisation. Right now the world is massively damaged. Waste starves many people of resources: we grow roughly twice the amount of food required to feed the entire world and waste enough to feed billions. That’s just one example: usable electronics rotting in drawers and camping gear that hasn’t been used in years. Cars people no longer drive but cannot be bothered to sell. All of it.
So once trade is mostly-digital and mostly-sensible, then we can start optimizing. Well-documented machine readable descriptions of what things are so that they can be found, valued, bought, sold, recalled and regulated effortlessly – then we can start to restructure the fundamental patterns of trade in a way which is compatible with the survival of the poor and nature itself.
This might sound pretty abstract, but here’s what we’re building at Mattereum. Every significant object in your life – Gore-Tex jacket, camera, laptop, e-bike, couch, airfryer – will have a unique ID either in its electronics or on a tag. There is a blockchain record of those objects – a Wikipedia for items – where every single object has its own page. All the data on that page is machine-readable and legally warrantied. If any of the information is inaccurate, you can make a claim for damages.
The first object to be tagged this way is my camera. It’s not science fiction!
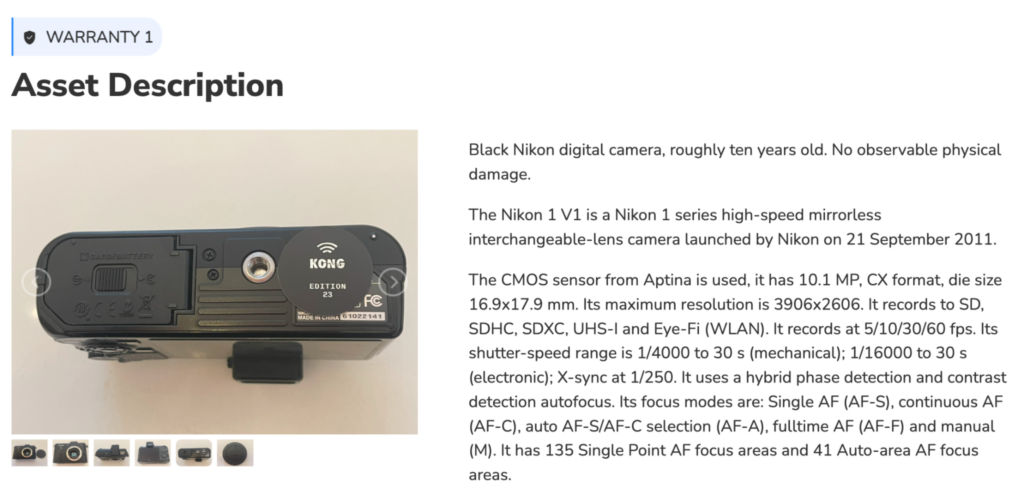
In our model of the future, when you sell an object to somebody else, usually an independent third party will take a look and verify the item’s condition before it is sold. That might be done by a bike shop or a camera shop or it could be a next-door neighbour. It could also be a fine art authenticator working for a Lloyd’s syndicate. It just depends. So goods can be bought and sold many times because you can always check what you are buying online and get reliable trustworthy data and cheap transactions: a global decentralized eBay which acts as a clearinghouse for all the stuff we’re done with and finds a good home for it.
What could that save on our environmental footprint? Probably 20% of all consumer goods manufacturing. It could easily be 50% once society has had a chance to adapt. Note that quality of life goes up: buy what you need, sell it again for almost exactly what you paid for it. Less waste, more of what you want when you want it. It’s like streaming music but for physical things. Not a sharing economy or a renting economy but a hyper-liquid second-hand economy.
AI can help in two different ways. The first is automated identification and condition reporting for physical items. Hold the toy in front of a camera and get an Asset Passport pre-populated with information about that toy line. It makes the whole scheme dramatically less labor-intensive.
The second way is optimizing what people have. Show the AI what you have and it can suggest things that you don’t use and should sell and also figure out things which you might like but do not currently have: a liquidity assistant helping to keep the markets moving. This sounds abstract, but one of our partner companies NeoSwap is using AI to build optimised barter networks so people can swap what they have for what they want even if there are dozens of people who all have to coordinate to make that exchange possible. It’s like magic.
RU: What I see with crypto is that average people see that wealthy people’s money makes money – and they want to do the same. They’re entering Casino Capitalism with high hopes and little data. Are you sympathetic to those who enter this arena purely to get some quick bucks?
VG: Bluntly, if US financial regulators had provided regulatory clarity about tokens ten years ago then we would be in an entirely different universe. Reg CF, Reg D and Reg A all provide regulated frameworks suitable for crowdfunding and other community-based fundraising. The legitimate projects raising funds could adhere to manageable SEC requirements to put their token projects on a secure legal basis, and the Ponzi schemes would have been unable to enter the market and compete against the legitimate projects. But without that regulatory clarity, the innovators were forced into legal gray areas, and it’s been a disaster. We needed more regulation early, not less. All kinds of seedy characters flooded into that gap and it really has been a betrayal of everything that I had hoped to achieve with my work in the Ethereum ecosystem.
Mattereum sat on its hands through not one but two bull markets in crypto and did not issue a token because the legal and regulatory structures were not mature enough to support a token issue on terms that we liked. This year we intend to issue a token on a fully regulated exchange in the European Union, playing by the rules. It should have been like that for everybody.
We’ve just announced a partnership with the Swarm Markets exchange to do things like splitting ownership of real estate into tokens – so you start with something like an industrial building and break the ownership up into thousands of tokens which are bought and sold on a regulated exchange by real estate industry professionals. It can be done for high-value cultural assets like fine art too, Mattereum has been working towards doing this for things like Stradivarius violins as part of a more general conservation strategy for fine instruments. We think the ability to transfer the ownership of heritage assets to tens of thousands of people is a good way of securing their long term future, as long as you can also get the governance right. A 300-year-old violin cannot be handled like an office block!
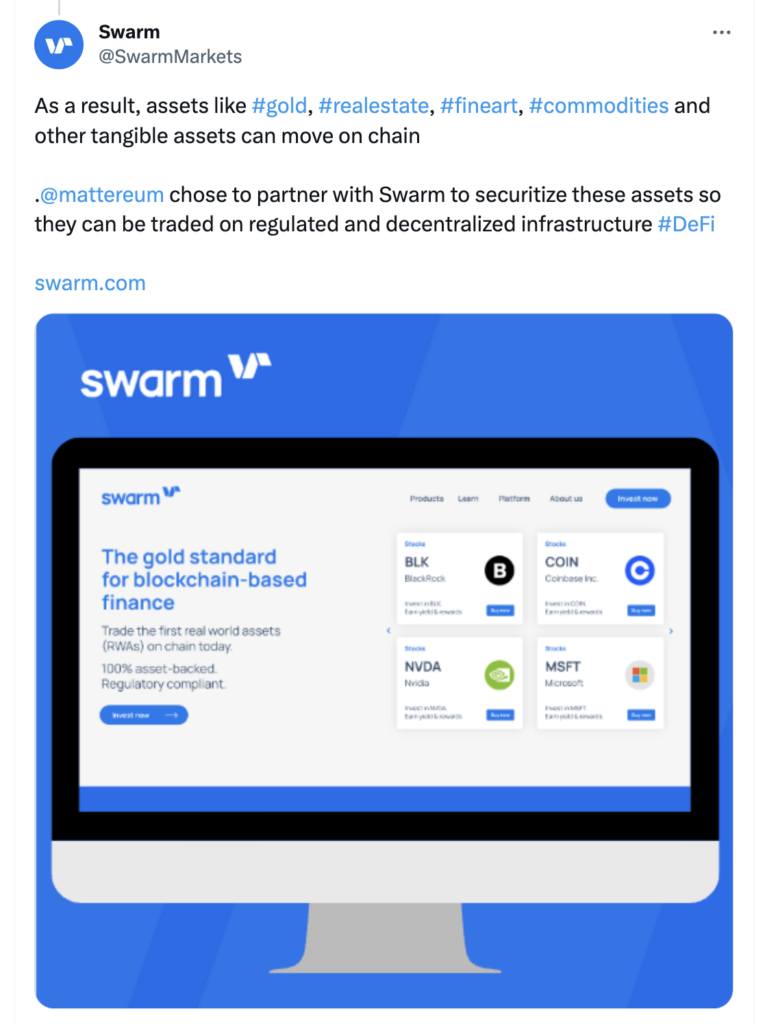
A lot of people in the blockchain industry have been chasing this vision for years. I would argue in fact that it is the original Ethereum vision from 2014 and 2015 which did not see the blockchain as existing outside of ‘the system’ in some kind of crypto utopia, but foresaw Ethereum as a mainstream part of everyday life particularly in business and commerce. That was always my vision for the future of Ethereum too.
RU: This sounds very TradFi. What about preserving what’s good about the cypherpunk intentions?
VG: The ultra transparent nature of the blockchain is a great fit for business, but it does not serve the cypherpunk vision of a crypto-anonymous society particularly well at all. In the UK there is intense support for this vision of the blockchain from our judges and legal think tanks, and we feel that is 100% the right way forwards.
The Law Commission in the UK is laying out an agenda for legislation which stands up digital assets not just as a class of regulated financial assets, but as a new class of property: digital stuff you can own rather than just ‘a financial instrument on a new technology platform’. I feel like in time this new legal approach might sort out a lot of the horrible problems we have handling the internet in law: copyright, software licensing, control of personal data like images or click trails, all that might be covered by the same class of legal abstractions.
But I 100% believe that cypherpunk pirate finance still needs to exist as a hedge against tyranny. That does not mean it has to be the dominant mode of finance using crypto technology. Rather, there should be a diverse environment with self-issued anarchist and libertarian tokens at one end, through to safe-as-houses blue chip regulated bond offerings from major financial institutions and even governments. We can’t afford to throw away either end of the market: everybody needs better transactional technology.
But this ponziconomy stuff is just a disaster. I really wish the whole token boom had happened inside of an equity crowdfunding framework under things like Reg D offerings rather than the mess we have now. And that’s the capability that this new EU-based partnership gives us.
Of course it did not have to be this way. The crypto industry could have established a credit rating agency, some kind of independent project review board, checks-and-balances to protect token buyers from unaudited smart contracts, rug pulls and unstable token designs. But that’s not what happened – a market failure to be studied by economists for the ages – and when you get a market failure that large, of course government steps in.
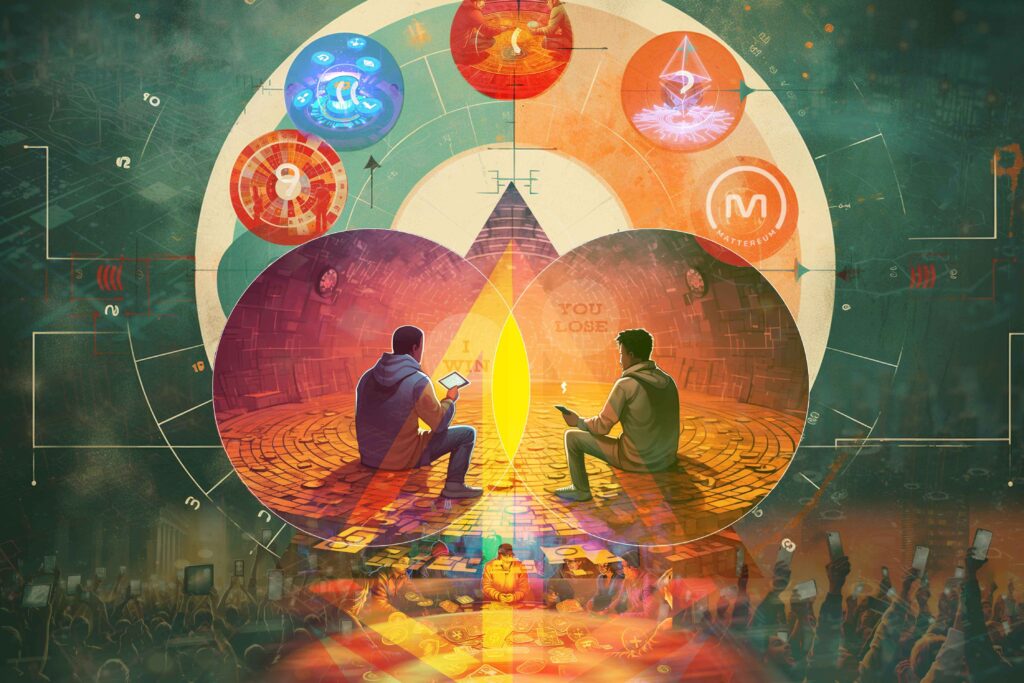
RU: Your ideas for saving the world sound pretty good. Of course, what actually occurs is likely to be messier even with the best intentions and the smartest actors.
But putting that aside, do you see anything in this bag of tricks for the precariat today in terms of immediate (in years not decades) relief? A lot of people feel stretched thin. They’re increasingly hostile to tech solutions, and they’re ready for populist revolutions… which tend to be quite authoritarian — either at inception or eventually.
I don’t even mean the wretched of the earth. Just the ordinary folks, say, in the US or Great Britain.
VG: Well, let’s talk about the wretched of the earth for a second first, then we can get on to the former middle classes. The rhetoric is that things are getting better for them. However as with unemployment statistics and inflation numbers the books are cooked. How do we define poverty? Is it really $1.90 a day? Is it $7.40 a day? It depends who you ask.
These folks are by-and-large seeing a ton of improvement in their lives: access to clean enough water, fertilizer to improve crop yields, gadgets like LED lights which displace kerosene, phones, the internet, all of that is helping. We’re climbing the stairs incrementally.
However at the end of that staircase there’s no landing: there’s a 40-meter drop into the abyss called climate change. Climate change brings drought or flooding, and that brings famine.
A reasonably stable one-acre farmer growing most of their family’s own food can expect slow, incremental quality of life improvements on the current trajectory. Most people agree on this. But then climate change comes and the land becomes unfarmable with crops dying unpredictably in the heat or the drought and suddenly those farms are completely wiped out, just like the dust bowl experience of America in the 1930s.
The poor farmers are the ones that climate change is most likely to kill in enormous numbers. They weigh on my mind constantly.
What can be done for the poor? Prevent climate change!
Now let’s turn our attention to the working class and middle class.
First up, working class and middle class are now the same class. What made the middle class the middle class is that their work could not be done by machines. Now that we have AI here and more AI coming over the horizon, everybody with a job is working class. It’ll take a generation or so for that shift to be felt fully but it is going to change everything. The middle class will rapidly find themselves even more disempowered and disenfranchised. The working class will have a “this is what we were telling you about” attitude to their new brethren. I don’t know what happens to the precariat and the gig workers, but I fear it comes down to: “Are you cheaper than a robot or not?” which is no way for a human to live. But it has been the experience of the industrial workers for centuries, and our lack of sympathy for their plight should be ringing in our ears now.
My writing has improved since I started feeding my copy through an AI system and asking it to edit. Shorter sentences, less commas. I’ve got maybe a million words of my own writing out there for a training data set: how long before the AI trained on my work is a replacement for me in many situations?
I view that as legacy, a way of scaling myself. But it’s also creating my own competitor. How weird does this get in ten years?
As for what is to be done, as the Irish say when asked for directions, “If I was you, I wouldn’t start from here.”
RU: Is there anything in all this that can be acted on now with rapid effect?
VG: Socialism as implemented in poorer societies has a bad habit of turning into genocide. I hate this but it is true. Socialism in affluent societies seems to work pretty well. The Scandinavian model is quite excellent. It secures human decency and avoids the extremely high personal and systemic financial costs of things like homelessness, delayed cancer treatment, untreated mental illness and similar problems which can be very inexpensive to prevent but hugely expensive in their later stages. The American system lets people crash through all the cheap-and-easy-fix stages of problems and right into the life destroying and budget wrecking late stages. This is unacceptable.
And I think that this is actually the way forward for us. There are a lot of questions about “what is the State?” How you answer that question shapes how you imagine the State helping people. I’m quite fond of “a prince is but a stationary bandit” as a general model, but social services are real, and the State does a lot more than steal. It also serves.
RU: OK so do you have a favorite model for what the state should be?
Mariana Mazzucato thinks of the State as being a kind of super-VC making 60-year bets on billion-dollar megaprojects and then reaping the rewards through tax revenue. In particular she points at Silicon Valley as a product of decades of defense spending on semiconductors and other primary technologies, on top of all the WW2-era codebreaking research on computation. Another common model is the idea that the State is there to maintain the rules of the game and ensure fair competition: a model rooted in concepts like antitrust legislation and consumer protection. A third model is that the State is kind of like a superparent. Germans used to call Chancellor Angela Merkel the Bundesmutter (Federal Mother).
I’d like to focus more on the idea of the State as an ‘insurer of last resort’. It has played that function in a truly spectacular way in the financial markets: the 2008 bailout damn near bankrupted entire countries and cost trillions of dollars. It’s probably the root cause of the inflation we are seeing now. “If we’re bailing out corporations, why not people?” runs a common logic. But it’s not the right logic: the bailouts arrive when the situation is at its most expensive to fix. Waiting for people to become homeless before getting them mental health treatment is the worst possible way to do things. We take that approach for everything: medicine by the emergency room, civil order by the gun, and ultimately peace only after war. If the State steps in to make sure that there’s enough money in the system to treat problems at the point where they are cheapest to treat, overall economic efficiency goes way, way up. Get the tooth filled, not crowned. Get the tooth crowned, not pulled. Get the tooth pulled when it’s rotten, not when it’s causing systemic infection. It’s not rocket science.
Same for homelessness and mental health treatment. I really am talking about insurance here, not a generic welfare mandate. I believe it’s so much cheaper to insure risks like major medical risks than to try to pile up enough money to pay: $50,000 for lifetime medical insurance, versus $2 million for a worst-case scenario expensive, rare, treatable illness.
And these systems don’t just operate at the national level. Friendly societies, ROSCAs, and many other structures exist to let people insure-and-invest together. The basic recipe is always the same: everybody pays money into a common pot, and if somebody has a major problem money can be taken back out again to help them. Exactly what the rules are varies, but it’s a kind of mini-institution which creates social solidarity where none previously existed. I’ve read estimates that as many as 40% of American men were members of such structures in the 19th century.
So that’s my suggestion. People who are scrambling for survival should look at the models from the old days, models that worked when life was hard, and update them for modern norms and problems. Pooling regular payments into shared pools which are carefully controlled and audited – or maybe theft-proofed using technology – has enormous creative power. My cryptographer friend Ian Grigg turned me on to the concept of the chama, which is like the bottom-up economic building block of Kenya. I look at that and think there’s basically no place in America which couldn’t use and adopt this model.
We have to rebuild bottom-up social power and I think it is a good idea to start there.
Let us know your thoughts! Sign up for a Mindplex account now, join our Telegram, or follow us on Twitter.